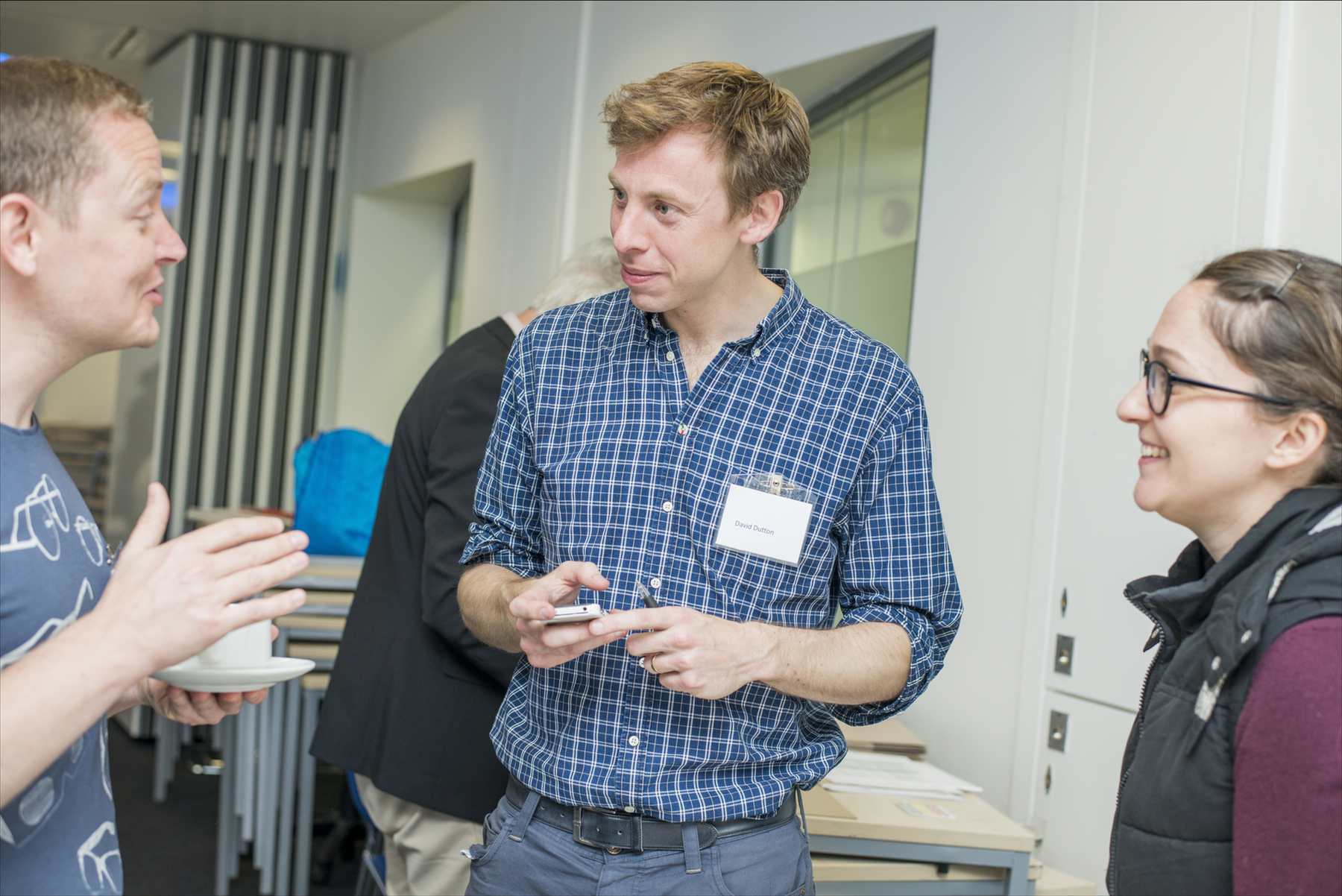
Free Associate Membership for Medical Students
Join the BSH to receive reduced registrations fees for the ASM and other BSH events and to receive regular member communications. After one year of membership you will be eligible to apply for BSH grants.
Membership is free of charge for medical students. For full member benefits and to apply for membership, please visit the Membership page.
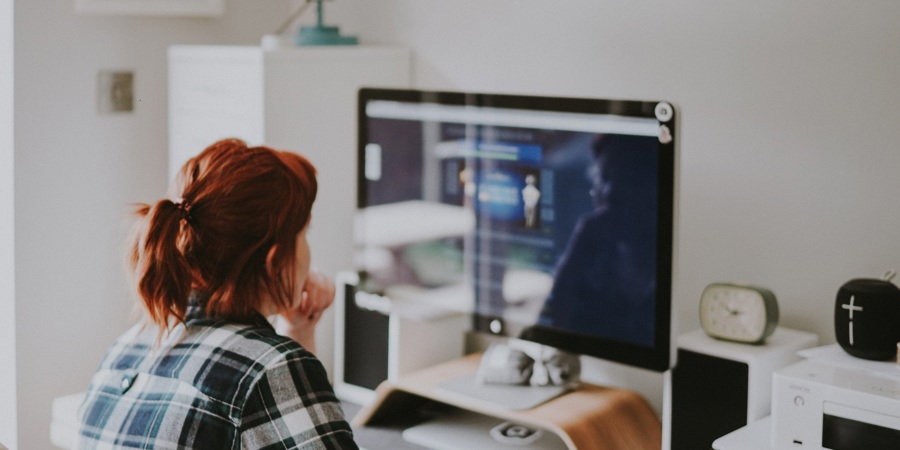
Haematology Student Societies
University societies are student-led groups which aim to bring together students with a shared interest in a particular field or activity. There are many haematology societies across the UK universities with the general aims of:
- promoting haematology as a diverse and interesting medical specialty
- providing haematology revision for medical students
- advertising haematology events, meetings and conferences
- increasing awareness and fundraising for haematology charities
A list of haematology university societies available for university students to join can be found here.
If you are interested in setting up a haematology society for your university, please contact us at [email protected].
Student Representatives, BSH Education Committee
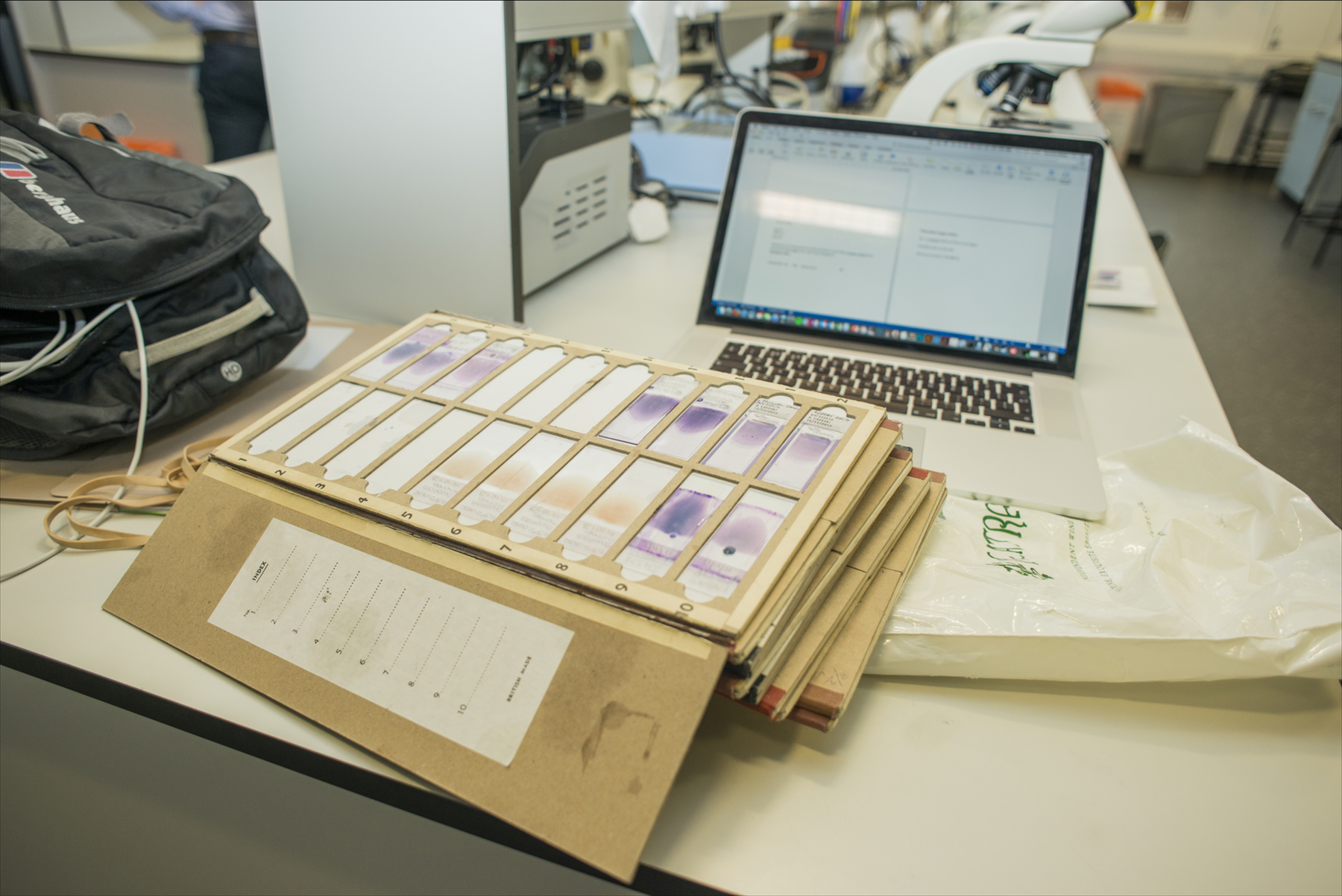
BSH education resources
Our educational resources can support your professional development. Whether you are an undergraduate, a junior doctor, a specialist trainee, a nurse, allied health professional or a healthcare scientist – there will be something here to suit you.
Basic level questions and tutorials on our education resources pages are aimed at undergraduate students.
These resources are open to non-members but you will need to create a profile on our website.
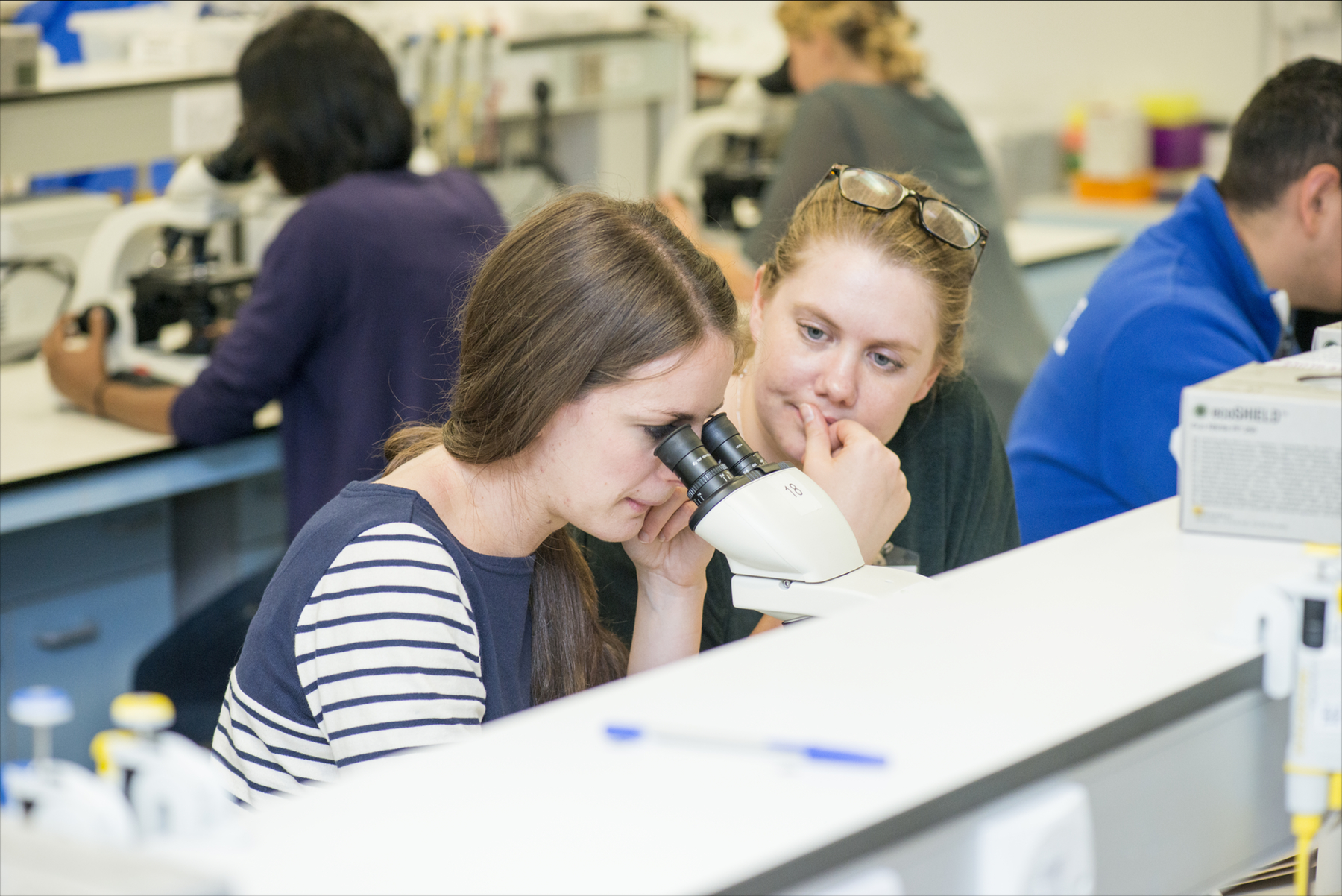
Careers in Haematology
Our Careers in Haematology booklet (PDF), aimed at undergraduate students, discusses the attractions of haematology, the range of conditions that haematologists treat and what makes a good haematologist
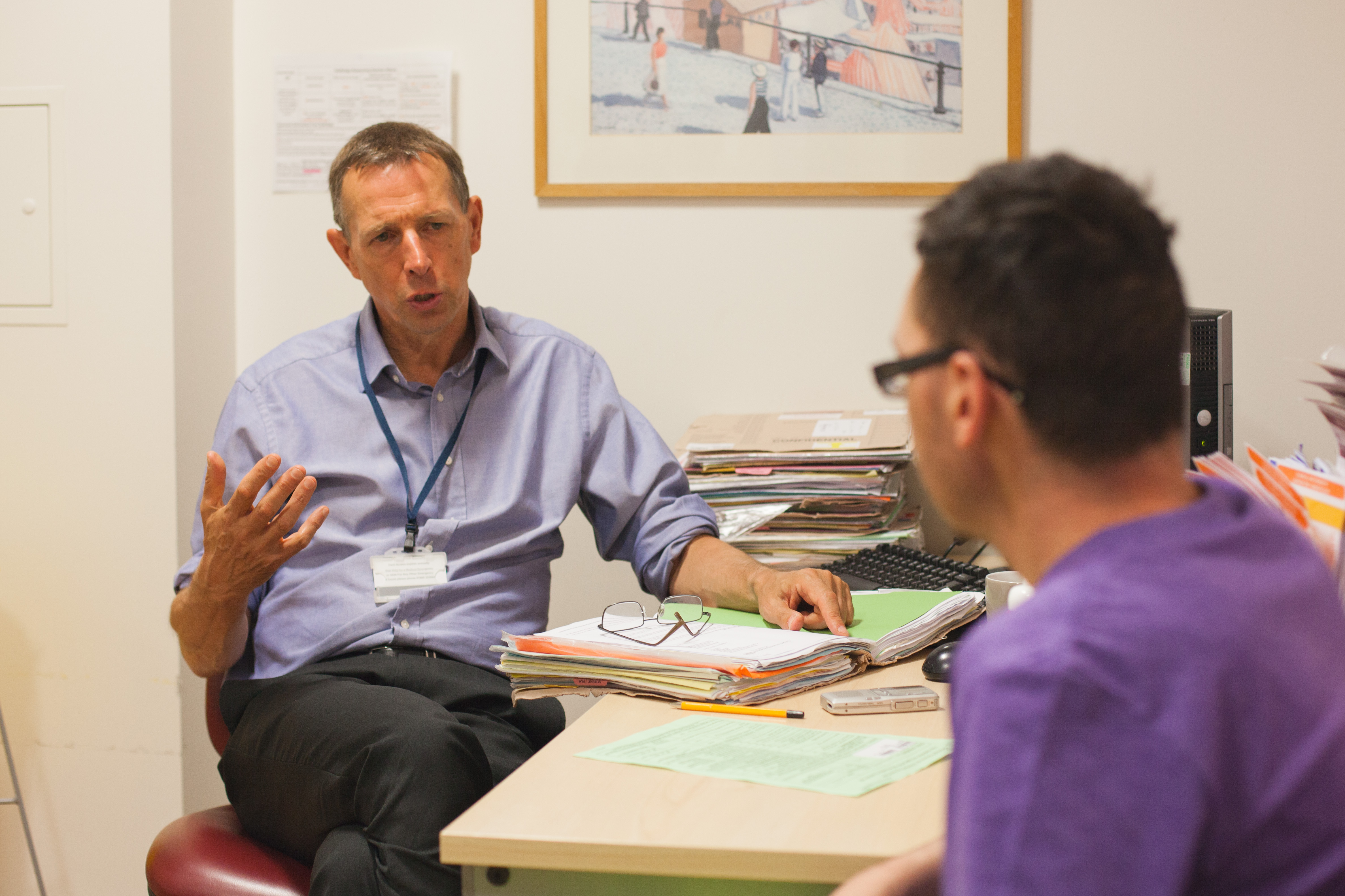
Haematology Curriculum for Medical Students
This curriculum is intended to act as a guide both to students and medical schools as to the extent and depth of knowledge of haematology that would be expected of newly-qualified doctors commencing their first posts. It is expected that this knowledge will be acquired during both the pre-clinical and the clinical years
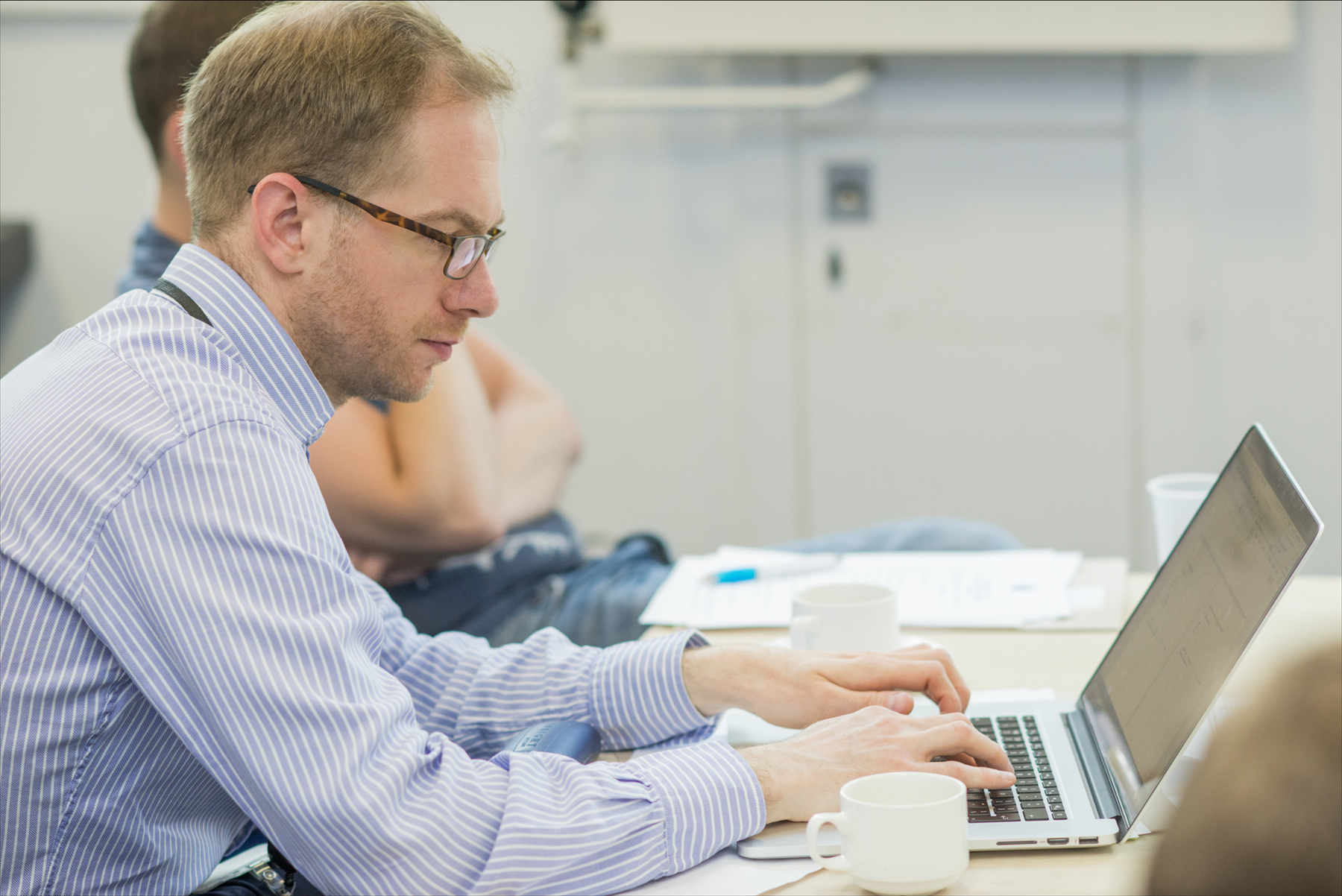
The title for the 2024 prize is 'How do we improve the design of clinical trials in haematology to meet the needs of patients?.
The deadline for submissions is 5.00pm on Friday 9 February 2024.
For further details please visit the Awards and Prizes page.
In 2024 the essay title was 'How do we improve the design of clinical trials in haematology to meet the needs of patients?'. The winner was Justine Chan, 4th year Medical Student from the University of Cambridge and the runner-up was Ananya Kannan, 4th year Medical Student from the University of Oxford.
“A physician is obligated to consider more than a diseased organ, more than even the whole man — he must view the man in his world” (Harvey Cushing).
Clinical trials in haematology have enjoyed some of the highest rates of success compared to trials in other medical fields.1 Many of these are randomised controlled trials (RCTs), the ‘gold standard’ to which most interventions are measured against and hence the focus of this essay. In RCTs, researchers select the population, the intervention and a comparator, to which participants are randomly allocated, and the outcomes of interest.
However, despite these trials’ success, they fail to account for the participating patients’ emotional, logistical and medical needs, as well as real-world patients’ needs. This leads to real-world outcomes that fall short of trial-reported data, as exemplified by comparing data from clinical studies on multiple myeloma and real-world reports in Table 1.
Table 1: Comparison of progression-free survival data shows that real-world utility may be lower than predicted by clinical studies, data from Richardson et al.2
Examples of multiple myeloma treatments |
Ranges of median progression-free survival (in months) from multiple studies |
|
Phase 3 clinical studies |
Real-world reports |
|
Carfilzomib |
14.9-22.2 |
3.2-10.6 |
Proteasome inhibitor-alkylator triplet |
12-18.4 |
6.5-16.2 |
Injectable proteasome inhibitor-immunomodulatory drug triplet |
18.3-29.6 |
9.4-25.3 |
Lenalidomide and dexamethasone |
11.1-184 |
6.6-21 |
Many of these failures can be attributed to fundamental flaws in the design of clinical trials. By improving four cornerstone aspects of trial design - patients, intervention, comparator and outcomes (PICO) - clinical studies can better meet the needs of patients participating in the trial, making the trial process better for these patients (Figure 1). This would arguably mean that the approved drugs better meet future patients’ needs for a treatment that balances medical success and quality-of-life.
Figure 1: Improvements (in red) at each step of simplified schematic of RCT design (in blue)
Patients: patient selection and participation
The first aspect of study design is the selection and recruitment of patients as participants. In this respect, meta-analyses of haematological clinical trials have highlighted that current patient selection criteria are not representative of the actual patient population, as stringent participant eligibility criteria explicitly excludes a large proportion of said patients.2 Consequently, the drugs produced may not meet the needs of all kinds of future patients.
For example, many trials restrict the age, degree of comorbidities, stage of disease, and prior treatment exposure of patients. This leads to older patients, those with more comorbidities and severe disease to be underrepresented in trials, such that the developed regimens may not meet the needs of a greater proportion of real-world patients. For example, an analysis of patients from the CONNECT-MM registry of multiple myeloma patients in the United States found that 40% of patients who are ineligible for RCTs have a significantly lower three year survival rate than those who are eligible.3 In many haematological conditions including multiple myeloma, these characteristics of advanced age and comorbidities are important considerations as they can predict mortality and toxicity, two factors clearly of importance to these patients’ needs for an effective but tolerable drug.
Some patient groups are also implicitly systematically excluded by the current design of many haematological clinical trials, including particular ethnic groups. For example, a study found that African American patients are over thirteen times underrepresented in clinical trials compared to real-world populations.4 This may be due to limited access: only 35.9% of African American individuals live in areas offering CAR T-cell therapy trials, a multiple myeloma biologic therapy.5 The rarity of some haematological disease also means that clinical trials may be disproportionately clustered at larger academic centres, which may not be accessible to Black Americans who are disproportionately more likely to be uninsured or cared for in underfunded hospitals. This disparity in access is particularly pertinent to haematological trials, as some haematological malignancies like multiple myeloma have almost two-fold incidence in blacks compared to white Americans, while some haematological diseases like sickle cell anaemia are more likely in those of African descent.6 Hence, it is particularly important that clinical trials are designed to be accessible to the population that needs it most.
Trials therefore need to work on expanding their patient selection criteria, to address explicit exclusion, as well as increasing the percentage of groups who are historically underrepresented. While the former is more easily achieved, the latter requires addressing structural barriers to participation. This could be incorporated into clinical trial design by matching the enrolment proportion of some ethnic groups that are historically underrepresented to the proportion of the ethnic group in the incident cases.7 Although this may result in trial delays, it may help to design drugs that are relevant to these patient groups. Clinical trials could design more decentralised participant recruitment strategies, using community medical centres as research sites, which are more accessible to such patients. They could also work with patient advocacy groups that have built trust with patients, such as the Leukaemia & Lymphoma Society’s collaboration with Native News Online to increase the awareness of clinical trials amongst Native Americans.8
Intervention: the emotional and physical toll of trial participation
Although it may be instinctive to argue that clinical trials should design interventions with the greatest medical benefit to patients, trials should also account for the emotional and physical burden that these interventions can cause patients.
From an emotional perspective, studies have shown that in many trials, patients feel like ‘guinea pigs’ or a ‘number’, rather than an appreciated part of the clinical trial. For example, a sentiment analysis on data from an online cancer support group showed that patients worried about being used only to the benefit of the trial/career of the lead investigator.9 This could be improved by involving patients in the study from early stages of conceptualization and planning of trial procedures, for example by including patients in workshops of protocols, allowing them to design trials with patients at the forefront. Andrew Scholl, a leukaemia survivor and founder of Patient Power, an organisation designed to support clinical trial participants in the United States, suggests that study designs should formally incorporate patients as “investors” in the trial, by systematically providing updates on the entire trial, not just their own health, as well as on the disease area in general.10
Such communication is another aspect of trial design that overlooks the information and educational needs of patients, which if unmet can lead patients to feel uninformed and therefore afraid, which was the most frequently reported unmet need in a systematic review of patients with haematological malignancies.11 When designing trials, investigators should therefore be careful to ensure that the trial is presented in a way that is possible for those with non-medical backgrounds to understand. For example, a shared platform with updates on the trial could be incorporated into the design of how the trial reports its outcomes, used by everyone from investigators to patients.10
Clinical trial interventions also create an additional logistical need of haematological patients. Failing to meet these needs can be burdensome for participants, many of whom already suffer a high disease burden compared to other patients. For example, transportation to and from the trial centre for treatment may be expensive and complicated for participants for multiple reasons. Many haematological patients are immunocompromised from disease and consequence of treatment, so going out repeatedly for trial check-ups, medication appointments and scans may be at odds with shielding at home. A systematic review of older patients in RCTs found that difficulties accessing the study site, especially at clinical research sites in expensive parking landscapes, were a significant barrier to participant recruitment.12 All in all, trials which are already medically onerous for patients may be made further physically taxing by such complex logistics.
These hidden costs represent failure on two counts: firstly, they fail to meet the participants’ logistical needs. Secondly, even if trial participants maintain compliance during the trial, such study designs also fail patients in the future, as the hidden costs of treatment regimes are suffered by those outside the motivating environment of trial participation.2 This leads to treatment fatigue, reducing the generalizability of trial results and failing to meet patients’ needs for feasible medical treatments.
To address these needs, study designs may be modified in two ways: firstly, sites should be made easier for patients to access. Study designs could consider using multiple local affiliated sites to obtain data, reducing the travel distance needed to one single study site. Lessons from clinical trials during the COVID-19 pandemic can also inform study designs that better meet patients’ logistical needs. For example, when participants were unable to attend in-person visits during the pandemic, decentralisation measures made use of telemedicine, like videoconference assessments for remote consent, and remote phlebotomy methods, which was reported to have high concordance with traditional phlebotomy methods in a COVID-19 antibody trial.13 These are particularly suited towards haematological disorders in which outcomes are mostly blood-based like sickle cell disease, as opposed to some oncological disorders in which outcomes may require bone marrow aspiration. Nevertheless, for data like quality-of-life, which should be a key component of most studies, studies could collect this remotely.
Secondly, study protocols should be simplified to reduce the number of unnecessary visits by these patients. An analysis of seven trials with 580 follicular lymphoma patients showed that in lymphoma clinical trials, bone marrow biopsies that are performed routinely before and after therapy to confirm complete response are not actually of value to predict overall or progression-free survival14. The authors found that the results of bone marrow biopsies only changed the assessment of complete response in 1% of subjects, and that in those who did have confirmatory biopsies, there was no difference in survival outcomes compared to those who elected not to have confirmatory biopsies. Hence, eliminating such unnecessary procedures can make the design of clinical trials more patient-focused.
Overall, these suggestions to study design are important not just to meet the logistical and financial needs of current patients, but also the needs of future patients for a feasible and realistic treatment regimen.
Outcomes: not clinically relevant to future patients
Clinical trials also need to select the outcomes against which the effectiveness of the intervention is assessed, however, these are not always outcomes that are the most relevant to patients’ needs. A systematic review of 538 randomised haematological cancer trials found that 85% of trials use ‘surrogate endpoints’, which are measures of treatment effects that may correlate with real clinical endpoints.15 Clinical endpoints of the greatest utility to patients are usually overall survival and quality-of-life, but in haematological cancer studies, they are commonly substituted for by progression-free survival and response rate. On one hand, although the use of these surrogate criteria speeds up clinical trials, arguably meeting patients’ demands for new drugs, they may not produce drugs that have the same effect in the real world. For example, studies on chronic lymphocytic leukaemia showed that in treatment-naïve patients, those receiving a treatment had a statistically significant improvement of progression-free survival but not of overall survival.16
Even amongst those using clinical endpoints, the aforementioned systematic review reported that only 20% of these studies used quality-of-life as their primary endpoint. This leads to actual treatments being poorly tolerated by patients as the trial design therefore does not adequately account for patients’ quality-of-life needs. For example, although recent studies of relapsed/refractory multiple myeloma reported discontinuation rates due to toxic side effects of 6.7-20.9%, real-world studies of cancer therapies have suggested that they are actually much higher, between 15.3-32.0%.2 Including such endpoints in haematological studies is therefore critical to producing drugs that meet patients’ needs for a tolerable treatment plan. This is particularly true for haematological malignancy patients, who suffer high symptom burden and impaired quality-of-life in both advanced and early stages of the disease.17
To remedy this, reporting methods could be standardised to include clinically relevant endpoints, such as quality-of-life measures. This should include haematological disease-specific questionnaires to reflect the specific aspects of disease and treatment burden. For example, in a survey of doctors and multiple myeloma patients, both parties rated symptom intensity as important for quality-of-life, but patients also reported that they were highly concerned about sexual function, weight gain, uncertainty about health and difficulty planning for the future, aspects which were not highly rated by doctors.18 These concerns are consistent with the protracted treatment necessary for many haematological disorders, as well as the plethora of treatment options which may worry patients as to whether they are making the right choice. It is therefore important to include patient discussions in the planning of the study to ensure that patient-reported outcomes that are of high priority to the patients themselves are embedded in the study.
Beyond PICO: questioning RCTs themselves
The proposed modifications thus far have focused on modifying each stage of the design of RCTs. However, this trial design often requires a large sample size to have statistical power. In haematological malignancies, for instance, in which the patient pool is inherently rare, adaptive randomisation and/or adaptive dose-ranging could be one way in which maximum patient benefit is achieved while still carrying out an investigation into the investigation’s effectiveness19.
This was exemplified by Giles et al., who was investigating three induction therapies for acute myeloid leukaemia in elderly patients20. Although they began with equal randomisation to three different arms with a planned recruitment of 75 patients, they used a responsive-adaptive randomization scheme that shifted the randomisation probabilities towards the therapies that showed early signs of being more efficacious. As the trial proceeded, the probability of randomisation to the treatment arms decreased steadily until randomisation to the standard-of-care control arm reached more than half, allowing for the trial to be stopped after only 34 patients. This example shows how adaptive trial design can be used to minimise the harm to patients and meet their individual needs for the best possible treatment available.
Conclusion
In conclusion, it is clear that haematology clinical trials have two populations’ needs to address: the needs of the trial participants, and the needs of the broader patient population with their disease. Many of the proposed improvements in this essay may help to meet these needs. Although this essay has focused on larger-scale phase III clinical trials, which affect the most patients, many of the recommendations can be applied to earlier phase trials as well. Those to highlight include suggestions regarding study site selection, which can ease both logistical needs of participants and broaden the patients that are recruited to make the trial more representative, as well as prioritising patients’ concerns by including them in the design process for patient-relevant outcomes and reducing the burden of the interventions. By prioritising the needs of participants, trials can therefore be more realistic and produce more relevant data to the needs of real-world patients.
References
- Mullard, A. Parsing clinical success rates. Nat Rev Drug Discov 15, 447–447 (2016).
- Richardson, P. G. et al. Interpreting clinical trial data in multiple myeloma: translating findings to the real-world setting. Blood Cancer Journal 8, 109 (2018).
- Shah, J. J. et al. Analysis of Common Eligibility Criteria of Randomized Controlled Trials in Newly Diagnosed Multiple Myeloma Patients and Extrapolating Outcomes. Clinical Lymphoma Myeloma and Leukemia 17, 575-583.e2 (2017).
- Rusli, E. et al. Racial Differences in Multiple Myeloma Survival and Treatment Using Pooled Clinical Trial and Real-World Electronic Medical Record Data. Blood 136, 9–9 (2020).
- Alqazaqi, R. et al. Geographic and Racial Disparities in Access to Chimeric Antigen Receptor–T Cells and Bispecific Antibodies Trials for Multiple Myeloma. JAMA Netw Open 5, e2228877 (2022).
- Kirtane, K. & Lee, S. J. Racial and ethnic disparities in hematologic malignancies. Blood 130, 1699–1705 (2017).
- Awidi, M. & Al Hadidi, S. Participation of Black Americans in Cancer Clinical Trials: Current Challenges and Proposed Solutions. JCO Oncology Practice 17, 265–271 (2021).
- Lannon, J. How Can Clinical Trials be More Inclusive? Alira Health https://alirahealth.com/education-hub/how-can-clinical-trials-be-more-inclusive/ (2023).
- Chichua, M., Filipponi, C., Mazzoni, D. & Pravettoni, G. The emotional side of taking part in a cancer clinical trial. PLoS ONE 18, e0284268 (2023).
- Scholl, A. How Can We Improve the Patient Experience in Clinical Trials?
- Tsatsou, I. et al. Unmet Supportive Care Needs of Patients with Hematological Malignancies: A Systematic Review. Asia-Pacific Journal of Oncology Nursing 8, 5–17 (2021).
- Buttgereit, T. et al. Barriers and potential solutions in the recruitment and retention of older patients in clinical trials—lessons learned from six large multicentre randomized controlled trials. Age and Ageing 50, 1988–1996 (2021).
- Wixted, D. et al. Comparison of a Blood Self-Collection System with Routine Phlebotomy for SARS-CoV-2 Antibody Testing. Diagnostics 12, 1857 (2022).
- Rutherford, S. C. et al. Relevance of Bone Marrow Biopsies for Response Assessment in US National Cancer Institute National Clinical Trials Network Follicular Lymphoma Clinical Trials. JCO 41, 336–342 (2023).
- Wesson, W. et al. Characteristics of clinical trials for haematological malignancies from 2015 to 2020: A systematic review. European Journal of Cancer 167, 152–160 (2022).
- Beauchemin, C., Johnston, J. B., Lapierre, M. È., Aissa, F. & Lachaine, J. Relationship between Progression-Free Survival and Overall Survival in Chronic Lymphocytic Leukemia: A Literature-Based Analysis. Current Oncology 22, 148–156 (2015).
- Ramsenthaler, C. et al. The impact of disease-related symptoms and palliative care concerns on health-related quality of life in multiple myeloma: a multi-centre study. BMC Cancer 16, 427 (2016).
- Wagner, L. I. et al. Content Development for the Functional Assessment of Cancer Therapy-Multiple Myeloma (FACT-MM): Use of Qualitative and Quantitative Methods for Scale Construction. Journal of Pain and Symptom Management 43, 1094–1104 (2012).
- Pallmann, P. et al. Adaptive designs in clinical trials: why use them, and how to run and report them. BMC Med 16, 29 (2018).
- Giles, F. J. et al. Adaptive Randomized Study of Idarubicin and Cytarabine Versus Troxacitabine and Cytarabine Versus Troxacitabine and Idarubicin in Untreated Patients 50 Years or Older With Adverse Karyotype Acute Myeloid Leukemia. JCO 21, 1722–1727 (2003).
Clinical trials (CTs) have the potential to generate high-quality evidence about the efficacy of interventions to improve patient care. Limitations of existing treatment options in haematology such as the debilitating side effects of chemotherapy/radiotherapy used to treat acute leukaemias and the lack of disease-modifying treatment for sickle cell disease (SCD) highlights the need for new therapies that align with the needs of patients. To achieve this, patients must be at the heart of CT design – CTs must address research questions that matter to patients, assess outcomes that are relevant to patients, recruit appropriate patient populations and engage and empower patients participating in the trial, as well as utilising novel trial designs to facilitate clinical translation. In this essay I will be discussing the above to explore how the design of haematological CTs can be improved to meet patients’ needs, and thus meaningfully impact clinical decisions and patients’ lives.
Choosing the appropriate question
To meet the needs of patients, it is essential to understand their experiences – this can be done by asking patients what symptoms matter the most to them, and targeting these areas in CTs. This is already being done by the James Lind Alliance (JLA), a non-profit organisation that brings patients, caregivers and clinicians together in JLA Priority Setting Partnerships (PSPs) to discuss and establish their top 10 healthcare priorities for future research1. A JLA-PSP established for Bleeding Disorders chose determining ‘what is the role and cost effectiveness of blood clotting tests that give immediate results at the bedside in managing medical, surgical or obstetric haemorrhage?’ as their top priority1. Given that major haemorrhage affects up to 40% of all trauma patients, 10% of cardiac surgery patients and is one of the most common causes of death worldwide in women at the time of delivery2, it is evident why the detection of coagulopathy in severe haemorrhage matters to both patients and healthcare practitioners. However, although this priority was identified in 2018, there is still a lack of high-quality data that evaluates the use of point-of-care viscoelastic haemostatic assays (VHAs) alluded to by the JLA-PSP, such as thromboelastography (TEG), thromoelastometry (ROTEM) and Sonoclot. These are quicker than laboratory coagulation tests, and although they are more expensive, when used correctly they may decrease inappropriate transfusion and product wastage, thus increasing cost-effectiveness3. Despite this, the 2022 British Journal of Haematology guidelines still recommend the use of serial laboratory coagulation tests (SLTs) every 30-60 minutes4, despite these having slow turn-around times (27-77mins) and a limited ability to predict major bleeding2. The needs of patients therefore still are not met in this area – future research should focus on comparing efficacy and cost-effectiveness of VHAs with SLTs.
However, a similar initiative was carried out by the American Society of Haematology Research Collaborative (ASH RC) for sickle cell disease (SCD)5. In 8 community workshops, researchers asked patients with SCD “outside of a cure, what SCD symptoms would the ideal medication address?”; the largest proportion of patients (30%) chose acute pain/crisis. Patients with SCD experience acute ischaemic pain episodes (otherwise ‘vaso-occlusive crises’) due to polymerisation/’sickling’ of deoxygenated sickle haemoglobin (HbS). These crises can limit educational achievement, career opportunities and quality of life (QoL)5 – this highlights the importance of designing CTs to limit acute pain crises to meet patients’ needs. One CT that attempted to do so was the phase II SUSTAIN CT; this investigated the efficacy of crizanlizumab, a humanised monoclonal antibody that binds to P-selectin and blocks the interaction between erythrocytes, endothelial cells, platelets and leukocytes, in preventing vaso-occlusive crises6. They demonstrated that crizanlizumab significantly reduced the annual rate of acute pain episodes that resulted in a medical facility visit vs placebo (1.63 vs 2.98). Crizanlizumab was subsequently approved by NICE in 2021, the first new agent for SCD to be approved in the UK in the last 2 decades7 – the SUSTAIN trial is thus an example of a CT attempting to cater to patients’ needs in haematology through focusing on a research question that addresses patients’ priorities.
Choosing appropriate outcomes
However, a recent multinational phase III CT (STAND) suggests that crizanlizumab may in fact not be effective8 – it showed no significant difference between crizanlizumab/placebo in annualised rates of vaso-occlusive episodes leading to a healthcare visit (2.49 vs 2.30). This led to the Medicines and Healthcare products Regulatory Agency (MHRA) revoking marketing authorisation for crizanlizumab and NICE withdrawing their recommendation7. The contradicting results of STAND and SUSTAIN may be due to the use of surrogate endpoints (reduction in painful crises requiring healthcare admission) in both trials. Given that STAND was multinational, it is likely that differences in cultural norms for pain treatment, healthcare usage and access to healthcare may have impacted the results. The use of surrogate endpoints is a key reason why CTs fail to meet patients’ needs and in the case of crizanlizumab, was a major blow to patients as the hope they had previously obtained was shattered. Despite this, 78% of randomised trials for all haematological malignancies between 2015-2020 used primary surrogate end-points rather than primary end-points of overall survival or QoL9.
One way of overcoming these limitations is using outcomes more relevant to patients, such as patient-reported outcomes (PROs). These are defined by the FDA as ‘measurements of any aspect of a patient’s health status that comes directly from the patient, without the interpretation of the patient’s responses by a clinician or anyone else10’. As SCD is a chronic disease, the use of PROs (e.g. QoL) as efficacy end points is particularly vital given that patients may be on lifelong treatment, which can substantially impact their QoL. Furthermore, pain is subjective, and there is evidence that physicians who have been treating the same patients for years may poorly judge patient’s symptoms – this is seen in chronic myeloid leukaemia (CML), where physicians frequently underestimate the severity of fatigue, muscular cramps and musculoskeletal pain11. However, unlike in SCD, in CML PRO data was used by the FDA in support of approving imatinib12. Imatinib is a tyrosine kinase inhibitor (TKI) that blocks the activity of the Bcr-Abl protein (which speeds up cell division and inhibits DNA repair), a product of the t(9;22) chromosomal translocation (the Philadelphia chromosome) that is the pathophysiological cause of CML. In the IRIS study12, newly diagnosed patients with chronic phase CML were randomly assigned to imatinib or interferon alpha with subcutaneous cytarabine (IFN + LDAC), and patients were asked to complete cancer-specific QoL and utility questionnaires at baseline and throughout treatment. The primary QoL endpoint was trial outcome index (TOI), a measure of physical function and well-being. They found that whilst patients receiving IFN + LDAC experienced a large decline in TOI, those receiving imatinib maintained their baseline level. Furthermore, patients who crossed over from IFN + LDAC to imatinib experienced significant improvement in their QoL. Since its approval in 2001, imatinib and other TKIs have revolutionised CML treatment, not only through directly targeting the pathophysiological cause of CML, but also by allowing most patients to have a good QoL compared to former chemotherapy drugs. This thus demonstrates the importance of selecting appropriate, patient-relevant outcomes as well as targeted therapies to ensure interventions meet the needs of patients.
Recruiting an appropriate patient population
As well as choosing appropriate outcomes, it is vital to recruit appropriate patient populations to meet patient needs. This is especially true in multiple myeloma (MM), an incurable malignancy of clonal plasma cells seen in the elderly which disproportionately affects ethnic minority groups in incidence and outcome13. Despite this, these groups are consistently underrepresented in MM CTs – the enrolment-incidence disparity for MM is 21% for African-Americans and 4% for Hispanics14. This lack of diverse patient populations in MM CTs reduces the external validity of the results to the patients most affected by the disease, as well as hindering our understanding of underlying biological differences that drive differential responses across ethnic groups. This may then compound the poorer outcomes seen in ethnic minorities.
To improve diversity in CT recruitment, it is important to use transparent communications that address mistrust in research. Unfortunately, there is evidence that fear of experimentation following cases of historic exploitation of ethnic minorities in medical research (such as the story of Henrietta Lacks and her immortalised cell line and the Tuskegee Study of Untreated Syphilis in the African American Male) is a barrier to CT participation5. To overcome this, community-directed CT education programs could be developed including testimonials of previous trial participants that future participants trust and can identify with.
Another barrier to CT diversity is eligibility criteria. General exclusions based on function status, organ function and comorbidities limit eligibility of elderly patients13. Furthermore, a recent retrospective pooled analysis of MM clinical trials found that Black patients (24%) and other race subgroups (23%) had higher ineligibility rates than White patients (17%), where failure to meet haematological laboratory treatment-related criteria was the most common reason for ineligibility among Black patients15. This may be due to higher rates of anaemia in Black patients with MM, as well as physiological benign neutropenia existing in Black patients. It is therefore essential that CT eligibility criteria should support the inclusion of older patients and ethnic minorities through considering ethnicity-based variations in laboratory values and using evidence-based criteria for haematological function.
An alternative solution to combat strict eligibility criteria is using real world data (RWD) to complement CT data – this has been done for diffuse large B-cell lymphoma (DLBCL), the most frequent high-grade Non-Hodgkin lymphoma, where <50% of real-world patients are eligible for most recent trials due to strict, numerous eligibility criteria16. However, using electronic health records, the Lymphoma Study Association developed an observational, multicentric prospective cohort study in France (REALYSA), and demonstrated that this (and thus RWD) can be used to assess the transportability of CT results to the general population16. This suggests that RWD can be used to combat the problem of reduced generalizability due to strict eligibility criteria, and thus aid the aim of meeting patients’ needs.
Trial designs
Another way to ensure CT results will translate to meeting patients’ needs is through ensuring CT design is rigorous. One step is ensuring trials are randomised rather than non-randomised – there has been a significant decrease in proportion of randomised CTs for haematological malignancies, from 26% in 2015 to only 19% in 20209. Randomisation increases the quality of evidence-based studies by minimising selection bias that may affect the outcome of results and thus reduce translation.
Alternatively, innovative CT designs reflecting an evolution in personalised/precision medicine could be used to increase translatability to patients’ needs. One example is biomarker-driven trials, which involve using molecular/genetic diagnostic/prognostic biomarkers to stratify and determine the eligibility of patients for CTs, so that CTs and thus subsequent interventions are targeted to those most likely to benefit. CTs using biomarkers such as FLT2, IDH1/IDH2 and Bcr-Abl have a 3- and 7-fold higher success rate for acute lymphoid leukaemia (ALL) and acute myeloid leukaemia (AML) respectively compared to those without17 – this thus demonstrates how biomarker-driven trials may help increase success rates of CTs and thus avoid costly failure and meet the needs of patients.
Furthermore, with advances in biomarker-targeted interventions, rather than following the traditional path of sequential phase I, II and III CT designs, there has been a drive to use master protocols that evaluate therapies in a more flexible fashion. These include basket trials, where biomarker-specific therapies target a common biomarker across multiple cancer types, umbrella trials, where multiple different biomarkers are assessed in a single cancer type, and adaptive platform trials, which use Bayesian statistics to evaluate multiple hypotheses in a single protocol (e.g. antineoplastic and supportive therapies in AML)18. Platform trials may serve especially effective in evaluating common and evolving haematological conditions such as venous thrombosis, as ineffective interventions can be dropped early on whilst new, more promising interventions can be added during the trial. However, the time and financial cost of biomarker screening for CT recruitment can be limiting – early screening of all patients for biomarkers as part of standard care and the subsequent creation of centralised database containing this information could overcome this difficulty and thus facilitate patients’ needs being met.
Meeting needs of patients participating in CTs
Finally, it is essential that the needs of patients participating in CTs are also met. To do so, patients participating could be asked what their ideal experiences would be, what information they would like and their preferred communication methods prior to the trial – trial designers can then try to implement these. This was done by the ASH RC for SCD5 – key aspects identified were empowerment (patients wanted to be treated as partners by study personnel, to be able to ask questions and understand how their participation made a difference), transparency (patients wanted all study information to be accessible, to be informed of their progress and health and to have results of the trial communicated to them) and practical considerations (study clinics having Wi-Fi, compensation provided throughout the CT rather than just the end, and minimal impact on personal life). To meet these needs, it is critical to have open communication with patients throughout the trial, to disseminate results using plain language summaries and to improve accessibility. This could potentially be aided by digitalisation, such as electronic consent forms and PRO surveys. One study investigating the feasibility of electronic PRO surveillance in patients receiving haematopoietic cell transplantation demonstrated that 94% of patients chose to use the electronic PRO system over paper-and-pencil, and that 100% of patients completed the surveys throughout hospital discharge19. Furthermore, patients were satisfied with readability, comfort and questionnaire length. Consequently, digitalisation is feasible and may improve patient convenience, thus meeting their needs. However, it is important to ensure minorities who may lack access to electronic devices are not disadvantaged by this – electronic devices and education should be provided appropriately, or attempts could be made to bring trials closer to patient residence (e.g. using home visits and local laboratory/imaging facilities where feasible).
Conclusion
In conclusion, CTs have the potential to hugely impact patients’ clinical outcomes and QoL, yet many haematological CTs fail to meet the needs of patients they intend to help. This is due to a lack of research into areas that patients prioritise, as seen in clotting tests for haemorrhage, a lack of focus on patient relevant outcomes, as seen in SCD, a lack of recruitment of appropriate patient populations, as seen in MM, inappropriate eligibility criteria, as seen in DLBCL, poor design of CTs and a failure to meet the needs of patients participating in CTs themselves. However, solutions to these problems are available, such as engaging/communicating with patients, using PROs, selecting appropriately diverse patient populations and eligibility criteria and using novel CT designs. Indeed, many of these changes are supported by recommendations made by the MHRA, which has recently announced a new regulatory framework to improve CTs in the UK20. Implementation of these suggestions and putting patients at the heart of CT design will hopefully enable CTs to meet patients’ needs. By doing so, they can achieve their aim, which should ultimately be to improve the lives of patients.
Bibliography
- Shapiro, S. et al. The top 10 research priorities in bleeding disorders: a James Lind Alliance Priority Setting Partnership. British Journal of Haematology 186, e98-e100 (2019). https://doi.org/https://doi.org/10.1111/bjh.15928
- Curry, N. S. et al. The use of viscoelastic haemostatic assays in the management of major bleeding. British Journal of Haematology 182, 789-806 (2018). https://doi.org/https://doi.org/10.1111/bjh.15524
- Srivastava, A. & Kelleher, A. Point-of-care coagulation testing. Continuing Education in Anaesthesia Critical Care & Pain 13, 12-16 (2012). https://doi.org/10.1093/bjaceaccp/mks049
- Stanworth, S. J. et al. Haematological management of major haemorrhage: a British Society for Haematology Guideline. British Journal of Haematology 198, 654-667 (2022). https://doi.org/https://doi.org/10.1111/bjh.18275
- Lee, L. H., Whisenton, L. H., Benger, J. and Lanzkron, S. A community-centered approach to sickle cell disease and clinical trial participation: an evaluation of perceptions, facilitators, and barriers. Blood Advances 5, 5323-5331 (2021). https://doi.org/10.1182/bloodadvances.2020003434
- Ataga, K. I. et al. Crizanlizumab for the Prevention of Pain Crises in Sickle Cell Disease. New England Journal of Medicine 376, 429-439 (2016). https://doi.org/10.1056/NEJMoa1611770
- National Institute for Health and Care Excellence. Crizanlizumab for preventing sickle cell crises in sickle cell disease: Technology appraisal guidance TA743 [Internet]. London; 2021. [Accessed 08 February 2024]. Available from: https://www.nice.org.uk/guidance/ta743
- Abboud MR, Cançado RD, De Montalembert M, Smith WR, Rimawi H, et. al. Efficacy, Safety, and Biomarker Analysis of 5 Mg and 7.5 Mg Doses of Crizanlizumab in Patients with Sickle Cell Disease: Primary Analyses from the Phase III STAND Study. Paper presented at 2023 American Society of Hematology (ASH) Annual Meeting in San Diego, California. December 9 - 12, 2023
- Wesson, W. et al. Characteristics of clinical trials for haematological malignancies from 2015 to 2020: A systematic review. Eur J Cancer 167, 152-160 (2022). https://doi.org/10.1016/j.ejca.2021.12.037
- US Food and Drug Administration. Guidance for industry: Patient-reported outcome measures: Use in medical product development to support labeling claims. US Department of Health and Human Services Food and Drug Administration. [Internet]. December 2009. [Last Accessed 08 February 2024]. Available at: http://www.fda.gov/downloads/Drugs/GuidanceComplianceRegulatoryInformation/Guidances/UCM193282.pdf. Accessed 06 February 2024.
- Efficace, F. et al. Patient- versus physician-reporting of symptoms and health status in chronic myeloid leukemia. Haematologica 99, 788-793 (2014). https://doi.org/10.3324/haematol.2013.093724
- Hahn, E. A. et al. Quality of life in patients with newly diagnosed chronic phase chronic myeloid leukemia on imatinib versus interferon alfa plus low-dose cytarabine: results from the IRIS Study. J Clin Oncol 21, 2138-2146 (2003). https://doi.org/10.1200/jco.2003.12.154
- Habr, D. and Corsaro, M. Reimagining diversity in multiple myeloma clinical trials. Hematol Oncol 40, 689-694 (2022). https://doi.org/10.1002/hon.2997
- Loree, J. M. et al. Disparity of Race Reporting and Representation in Clinical Trials Leading to Cancer Drug Approvals From 2008 to 2018. JAMA Oncol 5, e191870 (2019). https://doi.org/10.1001/jamaoncol.2019.1870
- Kanapuru, B. et al. Eligibility criteria and enrollment of a diverse racial and ethnic population in multiple myeloma clinical trials. Blood 142, 235-243 (2023). https://doi.org/10.1182/blood.2022018657
- Ghesquières, H. et al. Challenges for quality and utilization of real-world data for diffuse large B-cell lymphoma in REALYSA, a LYSA cohort. Blood Adv 8, 296-308 (2024). https://doi.org/10.1182/bloodadvances.2023010798
- Li, A. J., Dhanraj, J. P., Lopes, G. and Parker, J. L. Clinical trial risk in leukemia: Biomarkers and trial design. Hematological Oncology 39, 105-113 (2021). https://doi.org/https://doi.org/10.1002/hon.2818
- Neal, M. D., Lawler, P. R. and Zarychanski, R. Emerging clinical trial designs may accelerate translation in hematology: lessons from COVID-19. Blood Advances 6, 4710-4714 (2022). https://doi.org/10.1182/bloodadvances.2021005963
- Wood, W. A. et al. Feasibility of frequent patient-reported outcome surveillance in patients undergoing hematopoietic cell transplantation. Biol Blood Marrow Transplant 19, 450-459 (2013). https://doi.org/10.1016/j.bbmt.2012.11.014
- Medicines and Healthcare Products Regulatory Agency. Government response: consultation on legislative proposals. [Internet]. March 2023. [Last Accessed 08 February 2024]. Available at:
https://assets.publishing.service.gov.uk/government/uploads/system/uploads/
attachment_data/file/1144407/Clinical_Trials_Final_government_response_to_consultation.pdf
In 2023 the essay title was 'How do we prevent blood shortages in future?'. The winner was Sam Parsons, 4th Year Medical Student from the Swansea University Medical School and the runner-up Anatolia Nix, 2nd Year Medical Student from the University of Leicester.
Introduction
Transfusions of blood and blood products save countless lives every year. However, ensuring a steady supply of blood is a constant challenge for healthcare organisations, and the COVID-19 pandemic has further exacerbated the situation. Each year, approximately 2.5 million units of blood are transfused in UK hospitals.1 Nationally, at least six days' supply of red cell components are held to ensure their availability. However, in October 2022, shortages of red blood cells across multiple blood groups led to an amber alert being triggered, indicating a significant risk to supply and warning of potential impact on clinical care.2 The question now is: how can we prevent blood shortages in the future?
History and Context
The history of transfusion medicine dates to 1667 when Dr Jean-Baptiste Denys attempted the first recorded instance of a human blood transfusion. It was not until the 20th century, with the discovery of blood groups and development of safe storage and transfusion methods, that blood transfusion became widely accepted and ultimately the integral component of medical practice it is today. We rely on blood transfusions in the management of many conditions including leukaemia, sickle cell disease, and bone marrow failure syndromes such as aplastic anaemia, by replacing deficient or damaged blood cells with healthy ones.3,4 Moreover, without blood products many life-saving surgeries would be either impossible or too risky to perform.
The vital supply of blood products in the UK is managed by organisations such as the NHS Blood and Transplant (NHSBT) that oversee the collection, testing, and distribution of donations to hospitals in England. The Welsh Blood Service (WBS) and The Scottish National Blood Transfusion Service (SNBTS) are the respective regional services for Wales and Scotland. These organisations work together by sharing best practices, research, training, and by collaboratively monitoring and managing blood inventory levels and ensuring adequate supplies.
This essay will explore the various factors that contribute to blood shortages, such as changing donor behaviour and demographic, changes in medical practice, storage and transportation, and health events such as COVID-19.5 It will address potential strategies for preventing shortages, ranging from immediate practical approaches focussing on increasing donor participation, to the ongoing research in future technologies such as lab-grown blood.
Key Factors Contributing to Blood Shortages
Donor Factors
The supply chain has two key limiting factors at its foundation: the number of donors and the capacity of the collection service. The supply of blood products relies on the generosity and willingness of the public: increase the number of donors and we increase supply of blood. However, studies have shown that convenience is critical to donor retention: if blood collection services are unable to provide enough appointments, potential donors may be unable to book at a convenient time, or they may feel that donating is too much effort.6 Moreover, these donors may consequently be deterred from donating in the future, further exacerbating the shortage of blood products. For these reasons the present capacity of the service should be viewed as fundamentally linked to the number of potential donors, with far-reaching implications for future supply.
The factors pertaining to personal experience of donors is key, including service location, process, and education. If collection services are not in an easily accessible location, an area with limited public transportation for example, then potential donors may be discouraged. The actual process of donation is another important factor; some find the thought of donating blood intimidating whilst others may have had previously negative experiences. Finally, many eligible donors may not fully appreciate the importance of donation and therefore not prioritise it.
There are eligibility criteria that limit who can donate blood. For example, certain health conditions, medications, and lifestyle choices disqualify individuals, thereby further limiting the pool of potential donors. The exact criteria are determined by many factors, including epidemiology of the donor population, and have varied both around the globe and across time. For example, prior to 2021 there were additional restrictions on ‘men who have sex with men’ (MSM) in the UK.
Emerging diseases have been, and will almost inevitably be again, a concern for blood product production. An example is variant Creutzfeldt-Jakob disease (vCJD), the rare and deadly prion disease. To reduce the risk of transmission of vCJD through blood products, the UK government banned the use of domestic human plasma for the preparation of blood products in 1999 and had to import blood plasma from abroad.7 The US Food and Drug Administration implemented a ban on those who had resided in the UK over six months from 1980 to 1997, and this was projected to reduce blood donations in the US by 2.2%.8 More recent concerns about the future availability of immunoglobulins led to a recent review of the safety of UK-derived blood plasma. The UK Commission on Human Medicines deemed the risk of vCJD cases arising from the use of UK plasma as negligible, and in 2021 the ban on plasma for use in the manufacture of immunoglobulin products was lifted.7,9
It is important to note that when thinking about future blood shortages we must consider not simply volume, but also diversity. A diverse blood donor pool, including for example sufficient African and Caribbean donors, is crucial in the UK since these populations have a higher prevalence of certain genetic traits and blood antigen types. These blood types are important, particularly for those who require transfusions for conditions such as sickle cell disease.10
The complexity surrounding the regulation and supply of blood products can be seen as a barrier to formulating effective solutions. Therefore, it makes sense to concentrate efforts on straightforward, but still significant, hindrances to blood supply. Approximately 10% of blood donations are not accepted simply due to the donor having a low haemoglobin level, making this an area to be leveraged in the fight to ensure a steady supply of blood.11
Ageing Population
The world is witnessing a remarkable trend towards an ageing population. This shift is a result of a host of contributing factors, including extended life expectancy, and declining birth rates, and it is impacting both developed and developing nations alike. The ageing population presents a significant challenge to healthcare systems, including the supply of blood products. Because the elderly population is more prone to age-related health issues, they are more likely to require blood products. One study suggests those over 70-years-old have an eightfold higher consumption rate of red blood cells compared to those between 20-50.12 This, coupled with the relative decrease in eligible younger blood donors, presents a daunting outlook.
Supply Chain and Demand
Advances in medicine such as the increased volume of complex surgeries, advanced cancer treatments such as chemotherapy, and the growing number of transplant procedures, have placed a growing demand on the supply of blood products. In addition, emerging infectious diseases, most notably in recent history COVID-19, have the capacity to disrupt blood collection and donation, leading to decreased supply and increased stress on the blood system.7 The impact of the COVID-19 pandemic served as a reminder of the value of blood, and scarcity as a resource.13 Studies have shown that generally a conservative approach to transfusion is preferable, when taking into account benefit against risk, yet individual practices or broader policies may not always reflect this evidence base.14
The introduction of additional pathogen testing and reduction protocols for donated blood has heightened its complexity and cost. This presents a trade-off between improved safety and the reduction in the pool of donations combined with increased expense per unit due to additional overheads. This is a particular consideration as we continue to develop further testing capabilities in the future.
Solutions and Strategies for Preventing Future Blood Shortages
Donor Factors and Eligibility Criteria
The previous section examined several important factors affecting past and future blood supply. So, what scope is there to mitigate or address these problems?
Regarding donor participation: both recruitment and retention must simultaneously be addressed. Convenient locations and timings for appointments are critical. This means having sufficient blood collection sites located in accessible areas, flexible donation hours, and ensuring that the process of booking and donating is as quick and straightforward as possible. Moreover, for both recruitment and retention of donors, providing incentives such as recognition programmess and sharing feedback on the value of their donation can help reinforce the behaviour.
Even with well-placed centres there must be sufficient donor awareness and motivation to attend, therefore sufficient and effective communication and public outreach are essential. Further, ensuring the process of booking and donating is efficient and positive is key to retention of donors. Achieving these aims will rely on adequate numbers of well-trained and experienced staff, as it is ultimately the workforce that underpins the service. Therefore, adequate investment in staff is perhaps one of the most important aspects in securing future blood supply.
To maintain a diverse blood supply, it is crucial to identify and address any obstacles that may prevent individuals from different cultural backgrounds from donating. There is a lack of high quality research into the perspectives and attitudes of individuals from diverse cultures surrounding blood donations, therefore conducting further research can inform the customisation of services and outreach efforts, thereby effectively attracting a wider range of donors.15 Interventions should be made culturally relevant and use appropriate language. Additionally, the service should have in its employ members from relevant cultures in substantive roles.
A significant number of potential blood donors are declined due to low haemoglobin levels, which may only be a minor deficiency. Addressing this problem is critical as it leads to the waste of resources and time for all parties involved. Moreover, some donors may become discouraged from future donations if they repeatedly experience this issue. Additionally, studies have shown that alternate-day iron supplementation may be more effective than daily supplementation due to the regulation of iron homeostasis by hepcidin.16 Therefore, regular engagement with donors, reminders for iron supplementation, including the most up-to-date and evidence-based advice, and use of home-testing kits for haemoglobin levels, could help resolve this problem.
Supply Chain, Testing Factors, and Usage
The logistics and shelf life of blood products is key to the overall supply. Bacterial screening of platelet components has enabled the increase in shelf life, and increased safety.17 Further research into testing techniques for various diseases can help improve the supply of blood. Both in the UK and globally, where epidemiology differs, developing relevant screening tests that are cheap and reliable may make it feasible to relax some of the current restrictions on blood donation, thereby increasing the pool of available donors and resultantly improving the supply of blood products.
To help reduce the consumption of blood it is important we continue to focus on the education and training of physicians on evidence-based transfusion practices. This means promoting conservative approaches to transfusion and their rationale, along with the use of alternative treatments where appropriate. Transfusion guidelines and protocols should reflect the latest research on the most effective and efficient use of blood. Regular audits and evaluations of transfusion practices are a further opportunity for physicians to reflect on their own use of blood and identify areas for improvement.
Ageing Population, Decreased Supply, and the Future
Numerous methods have been discussed that can help buffer the potential for future blood shortages. However, they all rely on human supply, which as discussed, may in future be in jeopardy for reasons such as future pandemics, changing epidemiological factors, and the ageing population. A proposed alternative has been the use of artificial blood substitutes (ABSs).
ABSs have been an area of research since the 1980s, they include haemoglobin-based oxygen carriers (HBOCs) and perfluorocarbons (PFCs). Perfluorocarbons (PFCs) are synthetic blood substitutes made from fluorine and carbon-containing chemicals. They are chemically inert and effective in dissolving and transporting oxygen. HBOCs are blood substitute products made from human or bovine haemoglobin. These products have the potential to be used as alternative oxygen-delivery systems in medical emergencies and surgeries. However, they have been plagued by safety issues and a clinically approved oxygen carrying red blood cell (RBC) substitute is still evasive. Nevertheless, as our understanding of natural RBC function and biology continues to improve, it seems likely that research in this area will be key to securing future blood product security.
Lab Grown Blood
What if, rather than relying on the public and the inherent challenges present and future associated with their donations, it was possibly to produce required blood products in a lab? Numerous research groups have made significant progress towards producing human platelets for transfusion in vitro. In a recent study, the differentiation process of megakaryocytes (MKs), from induced pluripotent stem cells (iPS) to mature MKs, was successfully mapped opening up new possibilities for further research and development.18 Other advances in iPS cells are driving us closer to the production of red blood cells in sufficient quantity for transfusion.19
The main advantage of iPS cells is that they allow the generation of cells from individuals of known blood group type. Additionally, new methods of generating iPS cells, such as mRNA transfection have been developed, and recent studies have suggested that intermediary steps exist in the conversion of adult cells to iPS from which hematopoietic stem cells can be generated.20 Despite these promising developments, there are still several challenges that need to be addressed to translate these advances into clinical practice. One of the most significant of these is perhaps scaling production.
Another important challenge is ensuring the safety of the product. Removing contaminating human embryonic stem cells or iPS cells that could potentially give rise to teratomas in the recipient is of utmost concern.
In conclusion, in vitro cell culture theoretically represents an exciting potential solution to the limitations in blood cell production worldwide. The challenges of scaling production and ensuring product safety will need to be addressed for this possibility to be fully realised.
Conclusions
The availability of blood products remains a persistent challenge faced by healthcare organisations worldwide. The root causes of blood shortages encompass a range of factors, including donor behaviour, changes in medical practices, storage and transportation, as well as demographic shifts such as an aging population. To counter these challenges, a multi-faceted approach is required, incorporating both short-term and long-term strategies. Immediate measures include campaigns to increase donor participation and diversity and addressing key donor factors such as appointment availability and haemoglobin management. Longer-term plans involve investing in research for future technologies such as lab-grown blood. Close surveillance and continuous improvement of the entire blood supply chain is imperative, recognising that investing in personnel at every stage of the process, from donor recruitment to distribution, is essential to ensure a reliable supply of blood products for those who require it, now and for the future.
References
- Transfusion FAQs [Internet]. NHS Blood and Transplant. [cited 2023 Jan 29]. Available from: https://www.nhsbt.nhs.uk/what-we-do/blood-services/blood-transfusion/transfusion-faqs/
- Estcourt L, Royal College of Pathologists. Blood Shortages [Internet]. [cited 2023 Jan 29]. Available from: https://www.rcpath.org/profession/publications/college-bulletin/january-2023/blood-shortages.html
- Aapro M, Beguin Y, Bokemeyer C, Dicato M, Gascón P, Glaspy J, et al. Management of anaemia and iron deficiency in patients with cancer: ESMO Clinical Practice Guidelines†. Annals of Oncology. 2018 Oct 1;29:iv96–110.
- Killick SB, Ingram W, Culligan D, Enright H, Kell J, Payne EM, et al. British Society for Haematology guidelines for the management of adult myelodysplastic syndromes. British Journal of Haematology. 2021;194(2):267–81.
- Stanworth SJ, New HV, Apelseth TO, Brunskill S, Cardigan R, Doree C, et al. Effects of the COVID-19 pandemic on supply and use of blood for transfusion. The Lancet Haematology. 2020 Oct 1;7(10):756–64.
- Schreiber GB, Schlumpf KS, Glynn SA, Wright DJ, Tu Y, King MR, et al. Convenience, the bane of our existence, and other barriers to donating. Transfusion. 2006;46(4):545–53.
- Risk assessment: The risk of variant Creutzfeldt-Jakob disease transmission via blood and plasma-derived medicinal products manufactured from donations obtained in the United Kingdom [Internet]. European Centre for Disease Prevention and Control. 2021 [cited 2023 Feb 4]. Available from: https://www.ecdc.europa.eu/en/publications-data/risk-assessment-risk-variant-creutzfeldt-jakob-disease-transmission-blood
- Gottlieb S. FDA bans blood donation by people who have lived in UK. BMJ. 1999 Aug 28;319(7209):535.
- Critical risk assessment report: use of UK plasma for the manufacture of immunoglobulins and vCJD risk [Internet]. GOV.UK. [cited 2023 Feb 4]. Available from: https://www.gov.uk/government/publications/critical-risk-assessment-report-use-of-uk-plasma-for-the-manufacture-of-immunoglobulins-and-vcjd-risk/critical-risk-assessment-report-use-of-uk-plasma-for-the-manufacture-of-immunoglobulins-and-vcjd-risk
- Chou ST, Evans P, Vege S, Coleman SL, Friedman DF, Keller M, et al. RH genotype matching for transfusion support in sickle cell disease. Blood. 2018 Sep 13;132(11):1198–207.
- Mast AE. Low hemoglobin deferral in blood donors. Transfus Med Rev. 2014 Jan;28(1):18–22.
- Ali A, Auvinen MK, Rautonen J. The aging population poses a global challenge for blood services. Transfusion. 2010 Mar;50(3):584–8.
- McGann PT, Weyand AC. Lessons learned from the COVID-19 pandemic blood supply crisis. Journal of Hospital Medicine. 2022;17(7):574–6.
- Chen JH, Fang DZ, Tim Goodnough L, Evans KH, Lee Porter M, Shieh L. Why providers transfuse blood products outside recommended guidelines in spite of integrated electronic best practice alerts. J Hosp Med. 2015 Jan;10(1):1–7.
- Makin JK, Francis KL, Polonsky MJ, Renzaho AMN. Interventions to Increase Blood Donation among Ethnic/Racial Minorities: A Systematic Review. J Environ Public Health. 2019 Apr 15;2019:6810959.
- Stoffel NU, Zeder C, Brittenham GM, Moretti D, Zimmermann MB. Iron absorption from supplements is greater with alternate day than with consecutive day dosing in iron-deficient anemic women. Haematologica. 2020 May 1;105(5):1232–9.
- McDonald C, Allen J, Brailsford S, Roy A, Ball J, Moule R, et al. Bacterial screening of platelet components by National Health Service Blood and Transplant, an effective risk reduction measure. Transfusion. 2017;57(5):1122–31.
- Lawrence M, Shahsavari A, Bornelöv S, Moreau T, McDonald R, Vallance TM, et al. Mapping the biogenesis of forward programmed megakaryocytes from induced pluripotent stem cells. Sci Adv. 8(7):eabj8618.
- Mountford JC, Turner M. In vitro production of red blood cells. Transfusion and Apheresis Science. 2011 Aug 1;45(1):85–9.
- Lacoste A, Berenshteyn F, Brivanlou AH. An Efficient and Reversible Transposable System for Gene Delivery and Lineage-Specific Differentiation in Human Embryonic Stem Cells. Cell Stem Cell. 2009 Sep 4;5(3):332–42.
Background
We currently rely exclusively on donated blood from healthy donors, but this is a finite resource with a short shelf life. Between 2010 and 2011 in the UK, over 10,000 units of red blood cells (RBCs) were wasted (Thomas et al., 2013), and it is estimated that by 2050 supply will no longer meet demands (Shah et al., 2014).
Haemorrhage causes approximately 1.9 million deaths each year, with 1.5 million of these due to trauma (Cannon, 2018). In 2010 alone, there were 114,800 deaths due to haemoglobinopathies and haemolytic anaemias (Lozano et al., 2012). Preventing blood shortages is vital to enable the treatment of various clinical conditions, from catastrophic bleeds to transfusion-dependent chronic conditions such as sickle cell disease and β-thalassemia major.
Instances of blood shortages have been increasing worldwide. This is thought to be linked to the increasing demand for transfusions, decreasing donations, fewer new donors, stricter screening protocols and an ageing population (Sojka and Sojka, 2008). During the COVID-19 pandemic and associated lockdowns, donations were further reduced (Stanworth et al., 2020) alongside increased use of blood products in intensive care settings, a combination that resulted in national shortages. Many hospitals cancelled non-urgent surgeries or transfusions primarily to reduce the risk of coronavirus exposure, but with a secondary consequence of reducing demand for blood products (Al Mahmasani et al., 2021). This strategy is also employed in times of blood shortage such as the amber alert issued late last year (NHS Blood and Transplant, 2022). While changing prioritisation to reduce demand may work in the short term, it is understandably distressing for those on the already lengthy waiting lists. Alternative measures aimed at reducing consumption as well as increasing supply may be more effective in preventing future shortages.
Reducing consumption
Stock control measures
RBCs have a shelf life of around 35–49 days when refrigerated (Stanworth et al., 2020) between 2 and 6°C (Thomas et al., 2013) to maintain blood safety. However, if too strict, this can lead to unnecessary wastage.
Currently, RBCs must also be discarded after 30 minutes if left at room temperature despite limited evidence of safety risk. Guidance is relaxed slightly during transport due to the difficulty in controlling temperature, allowing for temperatures up to 10°C for 12 hours. An additional 5 hours is permitted in the case of refrigeration failure. Thomas et al. (2013) found that RBCs left at 30°C for 60 minutes for up to three times per unit of blood led to no significant increase in haemolysis. Increasing the time frame by which RBCs can remain at room temperature is likely to result in reduced wastage as there is more time available to return them to the blood bank when they are found not to be required. One limitation of this study was that, while it did measure factors associated with the viability of RBCs, it did not measure bacterial growth which would affect the safety profile of the blood. Further research is therefore needed before recommending this as a policy change.
Restrictive blood transfusion strategies represent a further opportunity. These have been used in times of short supply and aim to reduce the amount of blood used in order to preserve stock levels, but if safe, these could be implemented as standard procedure. Using erythropoietin, iron, folic acid or vitamin B12 to stimulate erythropoiesis in pre-operative or chronically ill patients could pre-emptively reduce their requirement for transfusions. Lowering target haemoglobin (Hb) values in patients on extracorporeal membrane oxygenation (ECMO) would also reduce the number of units used (Al Mahmasani et al., 2021). Changing thresholds for transfusion is limited because it is not suitable for many patient groups, and preventing access to blood transfusions may lead to harm or a worse quality of life.
Cell salvage
This technology involves using the patient’s own blood, saved from wounds or during surgery, for transfusion, and has the potential to reduce the need for donor blood. This is useful in patients with religious views that do not permit transfusions of allogenic blood, and those with rare blood types. However, the cost to set up and maintain the service is higher due to the process requiring specialist equipment and a trained staff member, which may limit its use for the majority of patients (Davies et al., 2006).
This technology could reduce demand for donated blood, especially in elective surgery, maintaining stock levels in the future. Although, despite using cell salvaging, some patients still required a transfusion (Davies et al., 2006), meaning that it is unlikely to be able to replace blood donations fully.
Increasing blood donation
Motivation to donate
Altruism was the most common self-reported reason why people continued donating blood. However, influence from family and friends, closely followed by the impact of media campaigns, were reported as the main influences to start donating (Sojka and Sojka, 2008). NHS Blood and Transplant regularly runs media campaigns (fig. 1) encouraging donors to share that they are a donor on social media with hashtags such as “#thisisamazing”. As friends and family strongly influence the decision to commence donating, this is likely to be very effective. The main slogan, “Save a life, Give blood” (NHS Blood and Transplant, 2021), will also likely play on the altruistic motivation to donate.
One potential method of increasing donations is by offering incentives, which can be controversial. While they may increase donations, research has yet to provide consistent results. It may increase the likelihood of attracting potential donors engaging in higher-risk behaviours or those with known blood-borne viruses (BBVs) being less likely to disclose this in order to receive the incentive (Chell et al., 2018).
Younger and first-time donors were found to be more likely to want an incentive, suggesting that this may be beneficial in attracting these groups to donate. However, having an incentive which is later removed is likely to reduce repeat donations (Chell et al., 2018). The NHS currently provides a selection of snacks and biscuits after a donation (NHS Blood and Transplant, 2016) which can be seen as an incentive.
Established donors were more likely to be against incentives, especially cash, but less so for health checks and low-value incentives such as cinema tickets (Chell et al., 2018). Donating via the NHS involves screening for Hb levels and BBVs; these health checks encourage some donors to continue donating (Sojka and Sojka, 2008). A cholesterol test sent to a lab from primary care costs approximately £3.19, and HbA1C costs £3.80 (El-Osta et al., 2017). While this adds to the cost to the NHS for each donation, it may provide good value for money if it increases donor retention. Additionally, when combined with a blood donation, it saves the staff-time cost associated with the tests being done at a general practice. Changes to the commercial model must be considered carefully prior to implementation however. A study by Willis et al. (2019) found that including a health report for every donor following blood donation was unlikely to increase donations enough to be cost-effective. The study found that each additional unit donated as a result of introducing health checks would in fact cost £136, clearly indicating additional factors must be taken into account, such as donor centre staff time and admin costs. Furthermore, not all donors may want or need additional health checks on top of current Hb and BBV testing as they do not have any bearing on the quality or safety of the donation.
Existing data thus implies that media campaigns may be more effective at increasing donations compared to introducing new incentives. Encouraging social media use by donors may present the most financially viable option.
Reducing barriers to donate
When asked about barriers to donating, the most common reason reported was laziness, followed by fear of needles (Sojka and Sojka, 2008). Often, little can be done to reduce a patient's fear of needles, but making donating easier can reduce the impact of laziness. The NHS Give Blood app allows instant appointment searching and booking, exports appointments to a calendar app and sends reminder texts (NHS Blood and Transplant, 2016). Increasing appointment times to include weekends and evenings made donating more accessible whilst not significantly increasing the cost to the NHS (Willis et al., 2019).
In 2021, changes to donation guidance aimed to increase donations by allowing more people to donate blood. For example, men who have had sex with men in the past three months were previously not allowed to donate; this ban has now been removed. Screening potential donors for sexual contact with people from HIV endemic countries has also been stopped, which had previously disproportionately affected Black African ethnic groups (The Lancet Haematology, 2021). These changes have the potential to encourage more people to donate in the future by reducing stigmatisation. NHSBT and UKHSA Epidemiology Unit (2022) found that the proportion of new donors of Black and Asian ethnicities did increase by 3.5% between 2020 and 2021, reinforcing this idea. Donors from these ethnic backgrounds are more likely to have rare blood groups and have a greater risk of sickle cell disease, which can require transfusion (The Lancet Haematology, 2021), highlighting the importance of increasing donations in these groups.
Even with these changes, there is room for improvement. Current guidance still prevents anyone who has ever injected drugs from donating, no matter the timeframe or BBV status (The Lancet Haematology, 2021). All donations are screened for BBVs, including HIV, hepatitis B and hepatitis C, with one in 6,000 testing positive and being discarded in 2021 (NHSBT and UKHSA Epidemiology Unit, 2022).
With improvements to BBV screening, as well as the treatment of donations to inactivate pathogens, the benefits of increasing access to donating seem to outweigh the risks. Hopefully in the future, anyone willing to donate will be able to.
Alternative transfusion sources
Stem Cells
There are many options to increase supply currently in development which we may see being used in hospitals in the near future. One example of this is producing blood products for transfusion from stem cells.
Haematopoietic stem cells (HSC) are multipotent cells in the bone marrow that produce RBCs through erythropoiesis. While they can proliferate, it is more difficult to mass-produce RBCs for transfusion than other immortal precursor cell lines (Ebrahimi et al., 2020). HSCs can be derived from cord blood (CB) or adult peripheral blood (PB). CB generates significantly more RBCs than PB, with a single unit predicted to yield 500 transfusable units. However, this is predominantly foetal Hb, compared to PB-derived RBCs which mainly contain adult Hb (Shah et al., 2014).
Human pluripotent stem cells (PSCs), such as embryonic stem cells (ESCs) or induced pluripotent stem cells (iPSCs), are far more suitable for large-scale production as they are immortal cell lines. ESCs have shown promise in the production of viable RBCs however do come with ethical considerations due to the use of human embryos. iPSCs have comparable properties but less ethical controversy as they are derived from somatic cells reprogrammed with transcription factors to achieve pluripotency (Ebrahimi et al., 2020). Most of the RBCs produced from these cell lines also provided primarily fetal Hb (Shah et al., 2014).
If production can be scaled up, stem cell derived RBCs could negate the requirement for donated blood entirely. iPSCs have the potential to generate RBCs from the patient's own cells which are a perfect match for transfusion in patients with rare blood types (Shah et al., 2014). However, limitations of RBC transfusions derived from iPSCs include a lack of clinical trials (Ebrahimi et al., 2020), therefore any risk to the patient is undetermined. Further research is also needed to determine the impact of RBCs containing predominantly foetal Hb in adult patients, and whether changes to the manufacturing process could result in these expressing adult Hb (Shah et al., 2014). Finally, this is currently a costly process (Ebrahimi et al., 2020) and likely to be expensive if scaled up, making it a potentially unsuitable investment for the NHS.
Haemoglobin Vesicles
A future technology currently undergoing phase I clinical trials is the use of haemoglobin vesicles (HbV) which could replace newly donated blood for transfusions. With Hb being the primary protein for oxygen transport, it was hypothesised that purified Hb could be used in lieu of RBCs in transfusion. However, it was found that free Hb has nephrotoxic effects; packaging it into vesicles essentially eliminates this risk (Sakai et al., 2022). Outdated donated blood is processed to extract purified Hb; pasteurisation and nanofiltration inactivates and removes any viruses, with the remaining Hb packaged into liposomes, now known as HbV. Deoxygenated, the HbV can then be stored with nitrogen for over two years at ambient temperature, vastly exceeding the current shelf life of RBCs (Sakai, 2017). Animal studies have been very promising, with HbV fluid resuscitation resulting in increased survival following haemorrhagic shock. The phase I clinical trial has also so far shown no severe adverse effects at doses of 10, 50 and 100 ml, with the continuation of the trial expected to start at doses of 100 ml (Sakai et al., 2022). Furthermore, a high-yield encapsulation method has allowed for more efficient production of HbV, which has implications for mass production in the future (Sakai et al., 2022).
HbV provides an ideal transfusion alternative for situations with limited access to blood donations or resources, such as refrigeration. Examples of these situations include pre-hospital emergency medicine or in the military. It also prevents pathogen contamination and removes the need for cross-matching, as the liposomes will be antigen-free (Sakai, 2017). This method, however, still relies on donations to source Hb and is likely to be expensive to operate at scale.
Future directions
As with many approaches in public health, prevention is better than treatment. Making minor changes to prevent blood shortages now will allow us to be better prepared to deal with future issues without sacrificing patient care.
Strategies to reduce consumption include reducing waste and supplementing donated blood with cell salvaging. Combining this with encouraging increased donations sets a good foundation for preventing shortages, without putting too much additional strain on NHS resources.
Finally, significantly reducing or eliminating the requirement for donated blood through innovative technologies such as iPSCs-derived RBCs and HbVs are likely to have a much greater impact in the future. They are exciting and potentially game-changing avenues for future research.
Bibliography
- Al Mahmasani L, Hodroj MH, Finianos A, Taher A. COVID-19 pandemic and transfusion medicine: the worldwide challenge and its implications. Ann Hematol. 2021 May;100(5):1115-1122. doi: 10.1007/s00277-021-04441-y. Epub 2021 Feb 1. PMID: 33527161; PMCID: PMC7850517.
- Cannon JW. Hemorrhagic Shock. N Engl J Med. 2018 Jan 25;378(4):370-379. doi: 10.1056/NEJMra1705649. PMID: 29365303.
- Chell K, Davison TE, Masser B, Jensen K. A systematic review of incentives in blood donation. Transfusion. 2018 Jan;58(1):242-254. doi: 10.1111/trf.14387. Epub 2017 Nov 6. PMID: 29106732.
- Davies L, Brown TJ, Haynes S, Payne K, Elliott RA, McCollum C. Cost-effectiveness of cell salvage and alternative methods of minimising perioperative allogeneic blood transfusion: a systematic review and economic model. Health Technol Assess. 2006 Nov;10(44):iii-iv, ix-x, 1-210. doi: 10.3310/hta10440. PMID: 17049141.
- Ebrahimi M, Forouzesh M, Raoufi S, Ramazii M, Ghaedrahmati F, Farzaneh M. Differentiation of human induced pluripotent stem cells into erythroid cells. Stem Cell Res Ther. 2020 Nov 16;11(1):483. doi: 10.1186/s13287-020-01998-9. PMID: 33198819; PMCID: PMC7667818.
- El-Osta A, Woringer M, Pizzo E, Verhoef T, Dickie C, Ni MZ, Huddy JR, Soljak M, Hanna GB, Majeed A. Does use of point-of-care testing improve cost-effectiveness of the NHS Health Check programme in the primary care setting? A cost-minimisation analysis. BMJ Open. 2017 Aug 15;7(8):e015494. doi: 10.1136/bmjopen-2016-015494. PMID: 28814583; PMCID: PMC5724165.
- Lozano R, Naghavi M, Foreman K, Lim S, Shibuya K, et al. Global and regional mortality from 235 causes of death for 20 age groups in 1990 and 2010: a systematic analysis for the Global Burden of Disease Study 2010. Lancet. 2012 Dec 15;380(9859):2095-128. doi: 10.1016/S0140-6736(12)61728-0. Erratum in: Lancet. 2013 Feb 23;381(9867):628. PMID: 23245604.
- NHS Blood and Transplant. #ThisIsAmazing. NHS. 2021. [Accessed: 2023Feb8]. Available from: https://www.nhsbt.nhs.uk/how-you-can-help/get-involved/download-digital-materials/thisisamazing/
- NHS Blood and Transplant. What happens on the day [Internet]. NHS Blood Donation. 2016. [Accessed: 2023Feb9]. Available from: https://www.blood.co.uk/the-donation-process/what-happens-on-the-day/
- NHS Blood and Transplant. Amber alert issued on blood stocks – existing O Neg and O Pos donors asked to make appointments. 2022. [Accessed: 2023Feb9] Available from: https://www.blood.co.uk/news-and-campaigns/news-and-statements/amber-alert-issued-on-blood-stocks-existing-o-neg-and-o-pos-donors-asked-to-make-appointments/
- NHS Blood and Transplant (NHSBT), UK Health Security Agency (UKHSA) Epidemiology Unit. HPR Volume 16 issues 9 and 10: News (29 September 2022). GOV.UK. NHSBT and UKHSA Epidemiology Unit; 2022. [Accessed: 2023Feb8]. Available from: https://www.gov.uk/government/publications/health-protection-report-volume-16-2022/hpr-volume-16-issues-9-and-10-news-29-september-2022
- Sakai H, Kure T, Taguchi K, Azuma H. Research of storable and ready-to-use artificial red blood cells (hemoglobin vesicles) for emergency medicine and other clinical applications. Front Med Technol. 2022 Dec 23;4:1048951. doi: 10.3389/fmedt.2022.1048951. PMID: 36619343; PMCID: PMC9816666.
- Sakai H. Overview of Potential Clinical Applications of Hemoglobin Vesicles (HbV) as Artificial Red Cells, Evidenced by Preclinical Studies of the Academic Research Consortium. J Funct Biomater. 2017 Mar 15;8(1):10. doi: 10.3390/jfb8010010. PMID: 28294960; PMCID: PMC5371883.
- Shah S, Huang X, Cheng L. Concise review: stem cell-based approaches to red blood cell production for transfusion. Stem Cells Transl Med. 2014 Mar;3(3):346-55. doi: 10.5966/sctm.2013-0054. Epub 2013 Dec 20. PMID: 24361925; PMCID: PMC3952923.
- Sojka BN, Sojka P. The blood donation experience: self-reported motives and obstacles for donating blood. Vox Sang. 2008 Jan;94(1):56-63. doi: 10.1111/j.1423-0410.2007.00990.x. PMID: 18171329.
- Stanworth SJ, New HV, Apelseth TO, Brunskill S, Cardigan R, Doree C, Germain M, Goldman M, Massey E, Prati D, Shehata N, So-Osman C, Thachil J. Effects of the COVID-19 pandemic on supply and use of blood for transfusion. Lancet Haematol. 2020 Oct;7(10):e756-e764. doi: 10.1016/S2352-3026(20)30186-1. Epub 2020 Jul 3. PMID: 32628911; PMCID: PMC7333996.
- The Lancet Haematology. Removing unfair barriers to blood donation. Lancet Haematol. 2021 Dec;8(12):e863. doi: 10.1016/S2352-3026(21)00347-1. PMID: 34826403.
- Thomas S, Hancock V, Cardigan R. The 30 minute rule for red blood cells: in vitro quality assessment after repeated exposure to 30°C. Transfusion. 2013 Jun;53(6):1169-77. doi: 10.1111/j.1537-2995.2012.03890.x. Epub 2012 Sep 21. PMID: 22995049.
- Willis S, De Corte K, Cairns JA, Zia Sadique M, Hawkins N, Pennington M, Cho G, Roberts DJ, Miflin G, Grieve R. Cost-effectiveness of alternative changes to a national blood collection service. Transfus Med. 2019 Apr;29 Suppl 1(Suppl 1):42-51. doi: 10.1111/tme.12537. Epub 2018 May 16. PMID: 29767450; PMCID: PMC7379655.
In 2022 the essay title was 'Will genomics replace the haematology and transfusion laboratory in the future?'. The winner was Jessica Whittle, Specialist Biomedical Scientist Haematology & Transfusion from the University of Oxford and the runner-up Scarlett Harris, 5th Year Medical Student from the University of Oxford.
Genetic tests, of known pathogenic variants, have a well-established role in the diagnosis of many haematological disorders, including haemophilia, thrombophilia, and various haematological malignancies, with the more recent integration of multi-gene panel testing. The advent of Next-Generation Sequencing (NGS) technologies in 2008 significantly reduced the costs and improved throughput of DNA sequencing, mediating a shift towards vast genome-wide research, substantially increasing the clinical applications of genomics. The development of UK infrastructure such as the NHS Genomics Medicine Service (GMS) is set to integrate the field of genomics, which interrogates the whole genome of an individual, into the routine care of many patients, presenting unique opportunities and challenges in healthcare. We are emerging on a new era of medicine, driven by molecular data; but what will this mean for current laboratory services? Will there still be a role for the haematology and transfusion laboratory in future medicine?
A New Era of Genomics-Driven Medicine
Every cell in the human body is constructed from one mutual blueprint – its genome. How each cell utilises its genome for differentiation and specialised cellular functions is mediated by the epigenome – regulating which genes are transcriptionally active or inactive. Genomics underpins the intricate process of haematopoiesis, during which all blood lineages self-renew. This commences with haematopoietic stem cells, which relay their genome to all subsequent cells, with epigenomic mechanisms directing differentiation into the various blood cell lineages. Haematopoiesis is not without error, with an estimated 14 base substitutions occurring every year of life1; hence many haematological disorders arise from defects occurring during this process. Therefore, it is reasonable to expect that functional aberrations observed within a cell can be often traced back to the genome, which has been replicated throughout haematopoiesis.
Inherited differences in the genome are widely recognised as the underlying cause of certain disease states, such as haemochromatosis and haemophilia. Most research to date has focused on the exome to characterise causative variants in inherited disorders, for which genetic testing has become essential in the diagnostic pathway. More recently, the improved accessibility to whole genome sequencing (WGS) has paved the way for large scale genome-wide association studies (GWAS) to better identify causative disease variants. For example, GWAS studies identified additional risk loci for Von Willebrand’s disease which has advanced biological understanding, extending beyond VWF to also include ABO, STXBP5, STAB2, and UFM12. Future genomics services will benefit from the outcomes of ongoing GWAS studies, and WGS may become routine in suspected inherited disease, to maximise the diagnostic yield in a single comprehensive test. Genomics should continue to supplement haematology practice in the inherited disease setting, without replacing the services required to identify individuals suspected of such disorders.
Towards Precision Cancer Medicine
Haemato-oncology has been at the forefront of molecular oncology developments, beginning with the identification of the BCR-ABL fusion protein in chronic myeloid leukaemia (CML) and subsequent development of imatinib, the first tyrosine kinase inhibitor of many in the field of oncology. Genetic testing forms an essential part of the diagnostic pathway for many haematological malignancies and is a key factor in the therapeutic strategy. For example, JAK2, MPL, or CALR variants occur in most myeloproliferative neoplasms (MPN) with the exception of rare triple-negative cases. Each variant carries different prognostic value and is used with clinical information to alter patient care, with targeted therapies such as ruxolitinib being available for JAK2-mutated myelofibrosis (MF) cases.
In the context of acute myeloid leukaemia (AML), WGS has demonstrated an improved diagnostic yield over conventional methods such as cytogenetics and improves risk stratification3. The array of clinically significant genomic aberrations, and the demonstrated clinical utility, has since led to the NHS GMS providing WGS for AML. This provides a comprehensive all-encompassing assay to optimise the diagnostic yield and implement the most effective therapeutic strategy; this approach will undoubtedly be applied to other haematological malignancies imminently. WGS offers some unique benefits, improving structural variant detection, including non-coding regions, providing global genome measurements such as mutational burden, and deriving mutational signatures offering potential prognostic and therapeutic value4,5. Initiatives such as the Genomics England chronic lymphocytic leukaemia (CLL) pilot study are beginning to integrate this information, to refine risk stratification for this highly heterogenous leukaemia6. Studies have also used WGS to improve minimal residual disease (MRD) testing, with personalised variants selected for quantitative monitoring7. Genomics has a clear role in the future of haematology services, and future diagnostic services should embrace this and enjoy the unique opportunities this field presents.
Genomics in Blood Transfusion
Most transfusion laboratories routinely rely upon antibody-based methods using the A, B and RhD antigens to determine a patient’s blood group and provide compatible blood. This is an efficient method, which can be automated, and has provided safe transfusions for many years. However, this is an oversimplification of the complex molecular basis of blood grouping, which contains a wide range of subtypes governed by the genome. Currently, blood group genotyping is only undertaken in specific circumstances such as for multi-transfused or DAT positive patients where standard phenotyping cannot be performed, and compatibility testing is problematic.
With the advancements in sequencing technologies, could blood group genomics become standard practice for all patients? DNA sequencing certainly offers many advantages, as it is feasible to test multiple grouping systems in a single test. There would be less uncertainty around discrepant blood grouping results, such reduced expression of red cell antigens, and rare subgroups can be identified8. Broader spectrum testing could look beyond ABO and RhD, having the potential to reduce incidence of transfusion antibodies which is especially important in chronically transfused patients. Blood group genomics could also improve antenatal care; genomics already dominates this application, with assays capable of detecting foetal cell free DNA in maternal peripheral blood. Genomics can confirm maternal and paternal zygosity status, improving predictions of foetal blood grouping and informing future pregnancies. By implementing genomics, blood group determination could be safer and more robust than ever, allowing detailed compatibility testing by application of a single test.
The Bigger Picture: An Unmet Need in Genomics
Haemato-Oncology: Phenotype Matters
The added value in genomic testing is evident, however the interpretation of genomics data is complex and requires the wider biological context to ensure appropriate application. Returning to the setting of MPNs – these are a group of disorders including polycythaemia vera (PV), essential thrombocythaemia (ET), and MF. There is a degree of overlap in the pathogenic variants driving MPNs, and variants found in clonal haematopoiesis of indeterminate potential (CHIP) which is considered benign. Detecting JAK2 V617F for example, is not diagnostic in isolation, and requires other haematological and clinical data to contextualise the genomic data and support any suspected diagnoses. Similarly, variants detected in myelodysplastic syndromes (MDS) can overlap with those found in AML, requiring a circulating blast percentage to differentiate. Subtle changes in the genome can lead to changes in cellular mechanisms, or may bear no change at all, hence the best way to accurately detect disease progression of MDS-AML is through a circulating blast count. While WGS is likely to become a key diagnostic test, in the field of haemato-oncology at least, wider laboratory and clinical data are crucial to applying the genomic data appropriately and form an essential part of diagnosis, staging, and monitoring.
Barriers in Transfusion Genomics
While the concept of genotype-matched blood seems ideal, there are several logistical and biological concerns with this approach. ABO blood groups are characterised by the expression of transferases capable of converting the red cell antigen H into A or B antigens, with group O being the product of an inactive transferase incapable of modifying H, dictated by ABO polymorphisms. Studies have highlighted subtle single base changes at the ABO locus can alter blood group antigens expressed, and there may be other mechanisms influencing gene expression which are not yet recognised8,9. ABO-incompatibility is an immune-mediated response to cell surface antigens, therefore testing directly for such antigens is the safest way to prevent a transfusion reaction, as subtle variants in the genome may confer an unexpected cellular phenotype.
The blood transfusion service relies solely upon donations for stock maintenance, and this is not an unlimited supply. So, is there a benefit to genotyping all blood recipients, when the donor pool is not rich enough to comprehensively match everyone? Does a one-off transfusion justify this level of testing? Integration of genomics more readily into transfusion services may reduce some difficulties for transfusion dependent patients, however its use in ad hoc transfusions will increase complexity and put pressure on the donor pool, without clinical benefit.
Most importantly, genomics does not account for serology, and overlooks pre-existing antibodies which cannot be detected using molecular techniques. The gold-standard ‘safe’ transfusion is based on compatibility testing of the donor red cells with the patient plasma, to observe any potential reactions in vitro before transfusion takes place. Genomics will not be able to match this gold-standard. Genomics techniques also cannot screen for haemolysis markers, in particular markers of complement activation, in which transfusion should be avoided or undertaken cautiously. Despite some benefits, genomics cannot meet all essential requirements of a transfusion service, emphasising the crucial role of the current transfusion laboratory.
Real-World Limitations of Genomics
Acute Care: A Demanding Discipline
It is vital to remember that the haematology and transfusion laboratory serves various clinical departments, covering a range of disorders. There is more to human biology than the genome we originate from, and not all disorders covered within the laboratory remit are governed by genomic aberrations. Routine haematology assays, such as full blood counts and clotting screens, are critical in the acute care setting. Accurate measurement of a haemoglobin is used to identify patients requiring transfusion and is essential in the management of acute bleeding episodes which could not be managed using genomics. Patients with idiopathic thrombocytopenic purpura (ITP) may present with a very low platelet count, but this is often immune and not associated with defined molecular changes. Patients with disseminated intravascular coagulation (DIC) present with a consumptive coagulopathy, are often a high bleeding risk, and require imminent intervention. DIC can be initiated via mechanisms such as infection or inflammation, this is not assessed using genomics. These are just a subset of many examples, proving current laboratory services are essential
Many haematology laboratories provide essential services to acute care facilities 24 hours a day, 7 days a week, with rapid turnaround times and automated, reliable equipment. Hospitals rely on this service to operate, to see patients at any time for any reason, and act on clinically urgent cases rapidly. Patients rely on this service to reach a diagnosis and be discharged as early as possible. There are several scenarios deemed medical emergencies, that are facilitated by the support of the haematology and transfusion laboratory. This includes diagnosis of acute promyelocytic leukaemia (APML), thrombotic thrombocytopenic purpura (TTP), and the urgent provision of suitable blood products, to name a few. The current laboratory services are equipped to handle these scenarios rapidly; a full blood count can be analysed in minutes, blood morphology can be examined within an hour, a clotting screen can be performed within half an hour, blood products can be provided on demand – this is something that genomics services cannot compete with.
Big Data Ethics
Novel omics technologies generate vast datasets, and while this presents unprecedented diagnostic opportunities, it also confers ethical and privacy implications. A considerable proportion of blood samples processed in many haematology laboratories are within normal range; current laboratories cater for this with automated processing systems and auto-validation of normal results. However, the definitions of ‘normal’ and ‘abnormal’ are much more ambiguous in genomics, and there is potential for incidental or secondary findings, with implications for the patient, their relatives, and in family planning. Data storage, security and traceability are also issues in the field, as is the potential for future scientific developments to change result interpretations and demand data re-analysis. These are novel ethical considerations which require informed consent beyond what is currently required for routine blood tests, likely requiring appropriate genomic counselling for the patient, who may refuse testing as a result10. This raises the key question of whether it is ethically appropriate to perform genomic testing routinely on healthy individuals, when there are much less complex blood tests available – genomics is a highly personalised data-rich field, and the complexities around big data ethics will make this a challenge to integrate into primary care settings.
Future Perspectives
The Future of Genomics
Future genomics services will almost certainly offer genome-wide analyses for a range of conditions across several medical specialties, although this has the limitation of requiring additional layers of clinical context for accurate interpretation. The field of genomics is set to expand beyond the genome, with new multi-omics workflows showing promise in the field of haemato-oncology. A recent study integrated WGS, targeted sequencing, bulk RNA-sequencing, and mass spectrometry proteomics and phosphoproteomics to define biological mechanisms in AML, which could be applied to select treatments11. Future genomics services may integrate various omics parameters to define cellular biology, improve risk stratification, and select therapies.
Single-cell analysis technologies are also an upcoming tool in cancer genomics – while recently emerging in the research setting, as the technology matures this could become translatable to future diagnostic services. Standard techniques such as WGS, analyse cancer as a single entity to provide an average readout, when cancers are in fact a diverse and complex network of cellular populations. This technology allows molecular interrogation of intratumoral heterogeneity in great depth, with newer methods beginning to integrate multi-omics of single cells12. This has translatable potential to optimise precision medicine approaches to haematological malignancies, by defining cellular hierarchies and designing a therapeutic strategy to target various malignant cellular populations, then monitoring clonal responses to treatment. MRD monitoring could be much more comprehensive with single-cell omics, without the any limitation on the number of markers used, to identify early leukaemic clones which are an indicator of relapse13,14. Single-cell approaches present an opportunity for genomics services to revolutionise the haematology clinic; whether this will be translatable into routine use only time will tell, although this technology is already beginning to enter clinical trials.
The Future Diagnostic Service
What does the future hold for diagnostic services? It is crucial to recognise the strengths and limitations of genomics to facilitate its integration into modern healthcare, and mediate future developments. The haematology and transfusion laboratory is not under threat as it provides an essential service ideal for acute and primary care settings, however genomics can revolutionise patient care in a specialised setting, especially in the context of oncology. The haematology clinic should embrace the new opportunities provided by genomics and readily integrate them into patient care, encouraging a synergistic diagnostic service with existing laboratories.
References
- Osorio FG, Rosendahl Huber A, Oka R, Verheul M, Patel SH, Hasaart K, et al. Somatic Mutations Reveal Lineage Relationships and Age-Related Mutagenesis in Human Hematopoiesis. Cell Rep. 2018;25(9):2308-16 e4.
- van Loon J, Dehghan A, Weihong T, Trompet S, McArdle WL, Asselbergs FW, et al. Genome-wide association studies identify genetic loci for low von Willebrand factor levels. Eur J Hum Genet. 2016;24(7):1035-40.
- Duncavage EJ, Schroeder MC, O'Laughlin M, Wilson R, MacMillan S, Bohannon A, et al. Genome Sequencing as an Alternative to Cytogenetic Analysis in Myeloid Cancers. N Engl J Med. 2021;384(10):924-35.
- Alexandrov LB, Kim J, Haradhvala NJ, Huang MN, Tian Ng AW, Wu Y, et al. The repertoire of mutational signatures in human cancer. Nature. 2020;578(7793):94-101.
- Hoang PH, Cornish AJ, Dobbins SE, Kaiser M, Houlston RS. Mutational processes contributing to the development of multiple myeloma. Blood Cancer J. 2019;9(8):60.
- Robbe P, Ridout K, Becq J, He M, Clifford R, Alsolami R, et al. Identifying High-Risk CLL to Predict Early Relapse after FCR Based Treatment Using Whole Genome Sequencing: First Results from the Genomics England CLL Pilot. Blood. 2016;128(22):2022-.
- Subhash VV, Huang L, Kamili A, Wong M, Chen D, Venn NC, et al. Whole-genome sequencing facilitates patient-specific quantitative PCR-based minimal residual disease monitoring in acute lymphoblastic leukaemia, neuroblastoma and Ewing sarcoma. Br J Cancer. 2021.
- Seltsam A, Hallensleben M, Kollmann A, Blasczyk R. The nature of diversity and diversification at the ABO locus. Blood. 2003;102(8):3035-42.
- Matzhold EM, Drexler C, Wagner A, Bernecker C, Pessentheiner A, Bogner-Strauss JG, et al. A 24-base pair deletion in the ABO gene causes a hereditary splice site defect: a novel mechanism underlying ABO blood group O. Transfusion. 2020;60(7):1564-72.
- Spicer J, Papanikitas A. Ethical, social and legal implications of genomics for primary care. InnovAiT. 2021;14(2):124-30.
- Rio-Machin A, Casado-Izquierdo P, Miettinen J, Bewicke-Copley F, Khan N, Demeulemeester J, et al. Integration of Deep Multi-Omics Profiling Veals New Insights into the Biology of Poor-Risk Acute Myeloid Leukemia. Blood. 2020;136(Supplement 1):39-40.
- van Galen P, Hovestadt V, Wadsworth Ii MH, Hughes TK, Griffin GK, Battaglia S, et al. Single-Cell RNA-Seq Reveals AML Hierarchies Relevant to Disease Progression and Immunity. Cell. 2019;176(6):1265-81 e24.
- Pellegrino M, Sciambi A, Treusch S, Durruthy-Durruthy R, Gokhale K, Jacob J, et al. High-throughput single-cell DNA sequencing of acute myeloid leukemia tumors with droplet microfluidics. Genome Res. 2018;28(9):1345-52.
- Ediriwickrema A, Aleshin A, Reiter JG, Corces MR, Kohnke T, Stafford M, et al. Single-cell mutational profiling enhances the clinical evaluation of AML MRD. Blood Adv. 2020;4(5):943-52.
Genomics, the study of the complete genetic sequence of an organism, is playing an increasing role in clinical medicine1. This change has been enabled by the rapid development of new technologies, which can sequence a human genome within a day at a cost of less than £7001. Initially the integration of genomics into healthcare has focused on the diagnosis of rare inherited diseases and the management of cancer1,2. However, it is hoped that genomic medicine will expand into other fields, meaning all specialties will need to adapt. It is important to consider what changes will be necessary, so we can begin developing new services and training programmes. For example, will the next generation of haematologists need to learn how to analyse blood films, or would the time be better spent learning the coding languages necessary for genomic analysis? Indeed, will any of the current techniques utilised in the haematology and transfusion laboratory survive the genomic revolution? Or will we come to rely entirely on genomics for all diagnostic, prognostic and therapeutic queries?
For genomics to supersede the traditional haematological techniques the following conditions must be met. Firstly, genomics must be superior, or at the very least non-inferior, to currently used tests. This discussion will comprise the bulk of the essay, and include an outline of current haematological tests, the genomic techniques which may replace them and the advantages and disadvantages of the latter. Secondly, the transition to genomic medicine would have to be cost effective, environmentally sustainable and allow equitable healthcare.
The Current State of Affairs
Before discussing genomics, it is important to establish the scope of the tests that would be replaced. Consider a patient with chronic myeloid leukaemia (CML). The first clue to the diagnosis may be leucocytosis on the full blood count, which microscopic analysis of the blood film would reveal to be mostly mature granulocytes3. From here, the key test would be RT-PCR or fluorescent in-situ hybridisation to establish the presence of the BCR-ABL oncogene which is pathognomonic for CML3,4. Further genetic testing aids in determining prognosis, namely obtaining a bone marrow aspirate for karyotyping to assess for the presence of cytogenetic changes associated with progression to the blast phase4. If this patient required a blood transfusion, serological tests would be used to determine their ABO and RhD groups5,6. The above journey is reflective of other haematological malignancies, many of whom have characteristic mutations with diagnostic and prognostic value7. Similarly, genetic testing is also utilised in the diagnosis of inherited haematological conditions, such as sickle cell anaemia8.
Genomics: An Overview
For genomics the patient journey would begin similarly, with the collection of blood or other tissue sample. These samples need to be fresh frozen to allow extraction of high quality DNA1, which can then be amplified and sequenced using next generation sequencing (NGS) technologies2,9. NGS involves sequencing multiple fragments of DNA simultaneously, the overlap between these fragments is then used to generate a complete genome sequence9. Once a sequence is obtained, the extensive process of analysis can begin. For patients with cancer, this would involve comparing the genomes of cancerous and healthy cells to identify mutations known or predicted to have an effect on prognosis and treatment response1.
It is worth acknowledging that genomics is only one branch of functional genomics. The latter field is devoted to the study of how genes and their products function to produce a phenotype in a cell or organism2. It comprises many different “-omics”, including transcriptomics, metabolomics and proteomics. Given these other“-omics” require additional techniques and are less well established clinically, for the purpose of this essay the capacity of genomics alone to replace the haematology and transfusion laboratory shall be considered.
Advantages of Genomics
The principal advantage of genomics is the sheer amount of information that can be obtained. For example, malignant cells typically have a large number of mutations, some of which determine diagnosis, prognosis and response to treatment. This is exemplified by Ph-like acute lymphoblastic leukaemia (ALL). Unlike many other subgroups of ALL, Ph-like ALL is not defined by a founding chromosomal rearrangement. Instead, the genome is peppered with genetic alterations in cytokine receptors and tyrosine kinases10. Whilst this subgroup currently has an overall poor prognosis, these multiple mutations allow for the possibility of targeted therapy10. Thus, genomic assessment of patients with possible ALL could give a rapid and accurate diagnosis of Ph-like ALL and other subgroups, personalised prognostication and targeted therapy in a single test. As a result, genetic tests currently used in the haematology laboratory, such as PCR and karyotyping, may become obsolete.
Another advantage of genomics is that it may be used to assess molecular heterogeneity. It is well-established that an individual’s cancer will consist of multiple subclones, derived from a founding clone but with divergent mutations10. These clonal populations can be precisely defined using genomics coupled with deep sequencing or single-cell genomics. Utilising these techniques early in treatment may identify clones which are naturally more resistant to therapy and are thus likely to become the predominant clone at relapse10. As cancer is a dynamic disease, serial genomic sampling may also demonstrate shifts in clonal populations and the development of new mutations, thereby enabling changes in treatment regimens before a new clone becomes established.
The advent of the genomic era is also likely to accelerate research. It is envisioned that the data generated from genomic sequencing, along with detailed clinical information, will be available to researchers2. Analysis of such a database may reveal new patterns of mutations associated with disease outcome or pathogenesis. For example, the genetic basis of ALL remains undetermined in a significant minority of patients10. Defining the characteristic mutations in these cases could lead to the identification of new treatment targets and subsequently improved survival rates. Another exciting area of research is using genomic sequencing of cell-free DNA in the bloodstream to identify circulating tumour DNA (ctDNA) as a method to monitor response to treatment or screen for malignancy. For example, in a 2019 study whole genome sequencing (WGS) of cell-free DNA of 1002 individuals identified four cases of lymphoma and one of myelodysplastic syndrome with excess blasts11. In the future, cancers may be diagnosed, monitored and treatment decisions made using genomic analysis of ctDNA alone11, reducing the need for more invasive testing.
Another area in which genomics is already proving to be useful is in the identification of disease-causing mutations in rare diseases2. This application would be valuable in the management of haematological diseases thought to be the result of as yet unidentified mutations. For example, 2% of patients with a bleeding disorder do not show abnormalities on laboratory testing12. It is thought that these patients are likely to have defects in their platelets or vessel walls12, which may be identified with genomic testing. Thus, genomics may supersede current clotting and platelet function tests by identifying both established and novel disease-causing mutations in patients with bleeding disorders.
There are additional advantages to using genomics over specific genetic tests in the diagnosis of inherited haematological conditions. For example, WGS in a patient with possible sickle cell anaemia could not only identify the disease-causing mutation, but may also be used to screen for genetic variations which render an individual more susceptible to specific complications such as osteomyelitis13. Similarly, genomes can be screened for markers which indicate the effectiveness of medications and the susceptibility to side effects. This includes genetic variants associated with opioid sensitivity14, potentially allowing more effective analgesia in sickle cell crises or haematological malignancies. The hope would be to produce more tailored care, resulting in more efficient allocation of resources and reducing unnecessary suffering.
Regarding transfusions, genetics is already an integral part of blood grouping and is replacing traditional serological tests in some institutions5,15. Indeed, genotyping may be superior as it can identify antigens expressed at a level too low to be detected by a serological test, or for which such an assay is not available5,15. Therefore, genetic testing enables extended grouping, allowing for more accurate donor-recipient matching to prevent alloimmunisation. In turn, these genetic tests may be superseded by WGS, which could be used to group all red blood cell (RBC) antigens.
Disadvantages of Genomics
The most significant limitation of genomic testing is that not all diseases are genetically determined. Whilst some, such as sickle cell anaemia, are determined by a single inherited gene mutation8, others are purely environmental, for example anaemia resulting from dietary B12 deficiency. In reality most diseases fall somewhere between these extremes, with genetic and environmental factors influencing development and severity of disease. Thus, whilst genomics may be used to identify those at increased risk of a specific condition, proving that an individual is currently affected will likely require additional testing. For example, to diagnose an individual with autoimmune haemolytic anaemia (AIHA), a test is needed to demonstrate the presence of antibodies directed against a patient’s own RBCs16. NGS techniques can be used to examine the antibody repertoire, however this is an incredibly complicated process. Antibody production requires B cells to rearrange and self-mutate their own genomes. As a result, each group of B cell clones has a unique genome producing a unique antibody. Assessing such incredible diversity typically requires a mix of both bulk and single cell sequencing17. Even then, genomic sequences of B cells in peripheral blood are not a perfect representation of antibodies present in sera17. Thus the current test of AIHA, the direct antiglobulin test16, is both simpler and more sensitive than genomic sequencing.
The limited capacity for genomics to replace antibody-based tests also has significant implications for transfusions. As discussed above, genomics could allow for more accurate matching for blood transfusions. However, given the numerous RBC antigens and limited blood supply5, it will be necessary to transfuse blood where there is donor-recipient mismatch for more minor RBC antigens, including those with alloimmunisation potential6. Whilst only a minority of patients will develop detectable alloantibodies following transfusion, these can cause delayed, or more rarely acute, haemolytic reactions6. Therefore, serological testing to identify the development of alloantibodies following transfusion will continue to be required.
Another significant disadvantage of genomic testing is speed. WGS is significantly faster than two decades ago1, however it still takes approximately a day to obtain a sequence and this does not include time for sample collection, DNA extraction and analysis. As a result, WGS may not be appropriate for emergency situations. For example, blood is often required urgently and whilst O-negative blood can be safely given, it is a valuable resource with limited supply. In such cases, it seems likely that there will be a continuing need for crossmatching, which can be in performed in under an hour.
Furthermore, whilst information gathering is one advantage of genomic testing, it is not without its drawbacks. Haematologists risk being overwhelmed by data of unclear clinical utility, a limitation demonstrated most clearly by variants of unknown significance (VUS)18. When genetic variations are identified in the genome they are classified as benign or pathogenic based on evidence such as the predicted effect of the mutation or whether it has been seen previously in individuals with the disease. However, this classification is often ambiguous, resulting in VUS – variants which cannot be confirmed as benign or pathogenic18. These are a considerable source of frustration for both patients and doctors. Patients may worry unnecessarily about variants which are in fact harmless. For doctors, ignoring a VUS may mean missing a mutation which is key to pathogenesis, alternatively further investigations may waste considerable time and resources determining that a variant is benign.
Similarly, the untargeted nature of genomics means that incidental, or secondary, findings will be commonplace. These are variants which have no impact on the primary diagnosis, but may, for example, indicate an increased risk of developing an unrelated condition2. Proponents argue these findings are beneficial as they could allow for preventative therapy. However, this relies on such treatment being available and efficacious in patients identified as at-risk based on genetic profile. Moreover, some patients may not want to be informed of secondary findings, which raises serious ethical and practical questions as to how we can ensure that their data will remain secure and when a disclosure should be made.
Other Considerations: Sustainability and Equality
The switch to genomic medicine will require significant financial investment. In addition to the cost of the sequencing itself, new infrastructure will be needed to transport and process clinical samples, analyse and store data, and train healthcare professionals1. Moreover, in a world increasingly affected by climate change, it is imperative that we consider the environmental impact of a switch to genomics. Genomic data is not a carbon neutral asset. Storing and processing such vast quantities of information requires a large and constant supply of energy which typically comes from non-renewable sources19.
In cases where genomics can be used to save lives, such as enabling personalised cancer therapy, the environmental and financial cost seems justifiable. Indeed, it may be more cost-effective as genomics could reduce time to diagnosis and prevent unnecessary treatment by providing targeted therapy. Conversely, in other cases the benefits may be more marginal and we need to consider whether this is a worthwhile allocation of limited resources. For example, as discussed above, a pharmacogenomic profile could be used to inform pain management during a sickle cell crisis. However, genetically determined sensitivity to analgesics and their side effects is only one factor which will influence patient response, environmental and developmental factors will also play a role. As such, it may be more effective to invest in additional specialist nursing staff to allow regular monitoring of pain, timely delivery of analgesia and assessment of side effects.
Another key factor to consider is whether genomic medicine will be equitable. A major problem with the current genomic databases is that they are dominated by data from individuals of European descent2,20. Therefore, it is more difficult to determine the significance of genetic variations and the risk of developing particular diseases in non-white individuals. Until these problems are addressed, relying on genomic medicine may result in a poorer quality of care for non-white populations and thus further entrench existing inequalities.
Conclusions
Overall, genomics is a tool to be incorporated into, not replace, the existing haematology and transfusion laboratory. There are clearly significant advantages to WGS in certain contexts, particularly for cancer where the ability to rapidly screen the complete genome in a single test will likely replace many of the current genetic tests. However, we need to be cautious not to generate excess genomic information unnecessarily. This is not only environmentally and financially costly, but risks wasting limited clinical resources on VUS or the identification of unwanted secondary findings. Moreover, we are more than the product of our genomes and most diseases arise due to complex interactions between our environments and genetics. Thus, the haematologist will continue to rely upon traditional genetic and non-genetic tests. Ultimately, WGS is like any other investigation, in that it should be used selectively in the right patients in the appropriate clinical scenario.
Bibliography
- Turnbull, C. et al. The 100 000 Genomes Project: bringing whole genome sequencing to the NHS. BMJ 361, k1687 (2018).
- Genome UK: the future of healthcare. (2020).
- Eden, R. E. & Coviello, J. M. Chronic Myelogenous Leukemia. StatPearls (2021).
- Smith, G. et al. A British Society for Haematology Guideline on the diagnosis and management of chronic myeloid leukaemia. Br. J. Haematol. 191, 171–193 (2020).
- Casas, J. et al. Changing practice: red blood cell typing by molecular methods for patients with sickle cell disease. Transfusion 55, 1388–1393 (2015).
- Tormey, C. A. & Hendrickson, J. E. Transfusion-related red blood cell alloantibodies: induction and consequences. Blood 133, 1821–1830 (2019).
- Taylor, J., Xiao, W. & Abdel-Wahab, O. Diagnosis and classification of hematologic malignancies on the basis of genetics. Blood 130, 410–423 (2017).
- Lanzkron, S. Sickle cell anaemia - Symptoms, diagnosis and treatment. BMJ Best Pract. (2021).
- Giani, A. M., Gallo, G. R., Gianfranceschi, L. & Formenti, G. Long walk to genomics: History and current approaches to genome sequencing and assembly. Comput. Struct. Biotechnol. J. 18, 9–19 (2020).
- Roberts, K. G. & Mullighan, C. G. Genomics in acute lymphoblastic leukaemia: insights and treatment implications. Nat. Rev. Clin. Oncol. 12, 344–57 (2015).
- Ogawa, M., Yokoyama, K., Imoto, S. & Tojo, A. Role of Circulating Tumor DNA in Hematological Malignancy. Cancers (Basel). 13, (2021).
- Sivapalaratnam, S., Collins, J. & Gomez, K. Diagnosis of inherited bleeding disorders in the genomic era. Br. J. Haematol. 179, 363–376 (2017).
- Xie, X. et al. Genetic Determinants for Bacterial Osteomyelitis: A Focused Systematic Review of Published Literature. Front. Genet. 12, 904 (2021).
- Smith, A. H. et al. Genome-wide association study of therapeutic opioid dosing identifies a novel locus upstream of OPRM1. Mol. Psychiatry 22, 346–352 (2017).
- Bakanay, S. M. et al. Blood group genotyping in multi-transfused patients. Transfus. Apher. Sci. 48, 257–261 (2013).
- Hill, A. & Hill, Q. A. Autoimmune hemolytic anemia. Hematology 2018, 382–389 (2018).
- Kim, D. & Park, D. Deep sequencing of B cell receptor repertoire. BMB Rep. 52, 540–547 (2019).
- Hoffman-Andrews, L. The known unknown: the challenges of genetic variants of uncertain significance in clinical practice. J. Law Biosci. 4, 648–657 (2017).
- Lucivero, F. Big Data, Big Waste? A Reflection on the Environmental Sustainability of Big Data Initiatives. Sci. Eng. Ethics 26, 1009–1030 (2020).
- McGuire, A. L. et al. The road ahead in genetics and genomics. Nat. Rev. Genet. 21, 581–596 (2020).
In 2021 the essay title was 'Will we have to see haematology patients face-to-face in the future:'. The winner was Claire Otasowie from the University of Oxford and the runner-up Oliver Marwood from University College London.
INTRODUCTION
Slowly then all at once, the nation’s ever-changing clinical landscape has boldly welcomed its newest dawn: the widespread use of remote healthcare provision (telemedicine). Its rise represented years of advancement played out over a matter of mere weeks, catalysed in no small part by the current COVID-19 pandemic1. What was at first an unprecedented move is now feeding transformative healthcare reform, even fuelling questions over the need to see patients face-to-face at all. This timely question bears particular relevance to haematology, not least as a field given to operating at the vanguard, but also as one whose patients may uniquely suit and benefit from long-distance care via telephone and video consultations.
THE ARGUMENTS ‘FOR’
Convenience is key
A significant advantage of telemedicine lies in its convenience. For the patient, remote consulting removes the need to arrange time off work, childcare or respite to attend an appointment. In addition to the time and expense saved on hospital car parking, telemedicine alleviates a significant financial burden that may eventually promote more equal access to healthcare1, and potentially carries more immediate positive effects on patient expectations.
It is an unfortunate truth universally acknowledged that when one goes to see the doctor, one does not tend to see the doctor on time, for punctuality always bows to patient needs. As such, attending a face-to-face appointment is often preceded by a long wait due to unexpected emergencies that interrupt the appointment schedule. Some patients accept the disruption with steadfast restraint, but others react angrily, venting their frustrations at reception staff and clinicians, and disturbing other waiting patients in the process. When consulting from the comfort of their homes, however, it is reasonable to assume that patients are less inconvenienced by delays. This is a possibility certainly worth exploring, given that in clinics, delays may be inadvertently worsened by resultant effects on clinician behaviour: compassion may drive the conscientious haematologist to extend an otherwise brief outpatient appointment, in order to compensate for the long wait time and restore patient satisfaction. Consistent with this, it is recognised that for the patient, the sacrificed time and effort of attending a traditional clinic comes with the expectation of a ‘reasonable’ time spent in the consultation room, almost irrespective of the complexity (or indeed simplicity) of the problem, yet brief teleconsultations are acceptable for similar presenting complaints2. The same will likely be true of uncomplicated video consultations, making them shorter and possibly saving clinician time2.
Thus, telemedicine affords clinicians more flexibility. Plus, unlike their in-person equivalent, telemedicine clinics are not restricted by the number of available consulting rooms. Ordinarily, this capacity constraint limits how many patient-facing tasks may otherwise be delegated to advanced nurse practitioners, for example, as opposed to clinicians.
Patients safety is paramount
Looking at the distinctive characteristics of a typical haematology patient reveals further benefits of a move to telemedicine. Notably, many with haematological conditions are immunocompromised, either by their condition or as a consequence of its often immunosuppressive treatment3: consider the auto-splenectomised patient with sickle cell disease, the pancytopenic patient with acute myeloid leukaemia, or the immune-ablated bone marrow transplant recipient. Besides careful antimicrobial (such as penicillin V in sickle cell disease) and immune prophylaxis (vaccination; immune globulin), haematologists stress the need to prevent patient exposure to potential sources of infection4. Herein lies another advantage of abandoning the physical waiting room: the significant infection risks of bringing immunocompromised patients together in waiting areas for phlebotomy clinics, for example, are now avoidable thanks to innovative solutions such as the establishment of off-site, drive-through blood collection facilities4.
Arguably, the COVID-19 pandemic has prompted a much-needed re-evaluation of haematology care. Beyond being a temporary measure during the ongoing crisis, replacing face-to-face appointments for haematology patients with telemedicine, where possible, might better protect this vulnerable patient group. It is therefore helpful that many haematological diseases, of which myeloproliferative neoplasms and low-grade non-Hodgkin lymphoma are pertinent examples, are characterised by a chronic course and outpatient care5, both of which lend themselves to a transition to telemedicine. It goes without saying that in-person provision must remain for both new and existing haematology patients who present acutely with potentially serious illness.
Coincident with the above, being able to ‘see’ patients in their home environment via video consultation could provide important contextual information that a clinician might not otherwise gain from traditional clinic-based appointments. The benefit of this is twofold. Firstly, this unfiltered interaction humbly reminds the haematologist to view things as the patient does: haematological disease is but a part of life to be balanced alongside other priorities such as family, relationships and work, all of which are impacted by the illness6. Haematologists must not lose sight of this holistic perspective, especially when managing patients with chronic disease. Secondly, candid video consultation may give a better impression of how the patient is coping at home, providing the chance for delicate conversations into what additional support a patient may need but not otherwise ask for.
Sustainability is a welcome bonus
Another argument in favour of telemedicine is its wider impact on the environment. For one, removing the need for patients to travel to hospital appointments has the potential to offset the sizable carbon footprint generated by motorised vehicles. The resulting decrease in transport-associated greenhouse gas emission and fossil fuel consumption naturally leads to improved air quality, as witnessed through stunning images captured around the globe during the earlier days of the natural experiment that is a modern-day pandemic7. Plus, it is logical to assume that with patients and clinicians eating at home rather than at hospital cafes and canteens, single-use plastic consumption would fall too. Coupled with paperless electronic prescriptions, healthcare services are moving towards more climate-friendly operations.
Yet, a more complex picture is emerging. Perhaps working from home was sustainable in the summer months when days were longer and temperatures more amiable, but with winter comes the considerable cost of heating and, by extension, lighting, countless individual homes instead of hospital clinics8. This does not even take into account the hugely power-intensive data centres required to securely handle the ever-increasing data flow between patients and clinicians consulting remotely7. Telemedicine’s true environmental impact is therefore yet to be elucidated.
THE CASE ‘AGAINST’
Implications for patient diagnosis
Current knowledge does, however, indicate that not seeing haematology patients face-to-face will impede diagnosis.
Without question, haematology has long and warmly embraced modern genetics for the diagnosis, classification, prognostication and treatment of disease: immunophenotyping, cytogenetics and molecular analysis of peripheral blood samples and bone and lymph node biopsies have become as important as the traditional morphological studies9. Moreover, this contemporary genomic approach has opened the door to ground-breaking targeted [immuno] therapies and personalised treatment of haematological disease. Taking chronic lymphocytic leukaemia (CLL), a common leukaemia characterised by marked clonal B-cell lymphocytosis, as an example: NICE recently approved a chemotherapy-free regimen for untreated CLL patients carrying a 17p deletion or TP53 mutation, who will now be offered venetoclax (potent BCL2 inhibitor that leads to apoptosis of CLL cells) plus obinutuzumab (targets CD20 on CLL cells, also causing their apoptosis) as an alternative to standard chemotherapy10. Indeed, precise diagnosis is perhaps the biggest single determinant of the most appropriate treatment for haematological malignancies.
Be that as it may, blood tests and laboratory investigations must be supplemented by a thorough patient history and informed by clinical findings. While the former can be suitably achieved remotely, the latter simply cannot. Physical examination, if only to take blood pressure and palpate the spleen and lymph nodes, is important both when indicated for clinical reasons and when not, because therein lies the opportunity to detect chance findings such as an indolent haematological malignancy.
Critics may propose this physical examination be replaced by non-invasive imaging. Yet, given that X-ray based modalities are the most commonly used, this screening approach unnecessarily exposes patients to radiation11 when palpation, even if preliminary, is surely favourable as the quicker, more cost effective and convenient action. Thus, the greatest limitation and indeed, danger, of telemedicine is the lost physical component.
Implications for the patient-doctor relationship
The patient-doctor relationship is similarly impacted by this physical distance. Consistent with existing literature on teleconsultation as an alternative to face-to-face consultation12, a recent study of video consultation through the Attend Anywhere platform in primary care reported that both telephone and video modalities entailed significantly shorter consultations that were more clinician-led and transactional than face-to-face encounters2. The authors characterised these remote consultations as orderly and continuous12, perhaps because the pauses natural to face-to-face interactions become alien and uncomfortable in the virtual context. Online, even the most perceptive and sociable of clinicians could not replicate the easy periods of silence as the patient redresses after examination or the doctor writes up a prescription. Yet, these crucial pauses lend themselves to the social small talk necessary for building rapport12 and eventually, trust between patient and clinician. Without this foundation, it follows that patients may feel less inclined to share their true ideas, concerns and expectations, particularly the telling ‘while I am here, can I ask you about…’ disclosures. Conceivably, this issue may be compounded by the fact that telemedicine makes it easier for patients to hide their illness from family and friends: for the patient struggling to come to terms with their diagnosis, it is much easier to explain away a brief phone call or video consultation, than a longer hospital visit. Needless to say, breaking bad news online is far from ideal; it then becomes all the more important that patients have adequate support13.
Nonetheless, a key limitation of this study2 lies in its lack of randomisation since patients self-selected their mode of consultation. Those who agreed to video consultation were younger (so possibly healthier) and discussed fewer problems than those who attended face-to-face appointments2. It is therefore unclear whether those who opted for face-to-face did so knowing they had multiple problems to discuss, or whether the modality itself better facilitates eliciting additional concerns. Although interestingly, patients presented with similar problem types across face-to-face, telephone and video appointments2. The age difference may then indicate that remote consultation suits a working, technically informed young population but is perhaps a step too far into the unfamiliar for their older counterparts. Rightly, GMC guidance cautions that telemedicine is not suitable for all clinical situations, much less for all patients14.
Of course, findings from general practice must be interpreted with caution as it is yet to be seen whether the same trends are seen in secondary care clinics, in view of the differing patient requirements and expectations after specialist referral. Ironically, an early indication of how this may play out comes once again from our Italian colleagues: researchers conducting a telemedicine study among haematology patients at the Bologna University Hospital found that though patient satisfaction was retained with a switch from in-person to telephone appointments during the pandemic, patients did perceive a lack of physical interaction5. This further emphasises how much haematological care goes beyond the technical diagnostics, instead involving a strong patient-doctor relationship that telemedicine cannot replace. Indeed, the prefix ‘tele’ comes from the Ancient Greek meaning at a distance or far away: it is unsurprising then, that this distance can be felt by both patient and clinician.
Implications for clinicians
Finally, no essay evaluating the merits of telemedicine for haematology patients would be complete without due consideration of its impacts on their clinicians. Not seeing patients face-to-face is a far cry from traditional practice; medics will undoubtedly miss the personal experience of seeing patients face-to-face. Telemedicine imposes a personal and professional isolation that may be particularly challenging in a discipline as sociable as haematology, where clinicians are used to regular interaction with their long-term patients, and with other specialists in the hospital, who rely on haematologists for advice on blood results or clotting problems15.
What is more, care must be taken that clinicians do not suffer from the physical demands of their new role with fatigue, posture issues and repetitive strain injury or neck issues from using landlines all day being notable concerns16.
FUTURE DIRECTIONS
Alongside the above, pressing issues remain concerning the future wider impacts of telemedicine. For one, it is worth considering how a move away from seeing patients face-to-face will impact clinical training at all stages. Telemedicine costs medical students vital early opportunities to build confidence in their clinical skills by replacing what was a chance to consider a case and examine the patient under the supervision and guidance of a senior clinician1, with sometimes little more than an observer’s role. While telemedicine might permit medical students the flexibility to sit in on a wider variety of virtual specialty clinics, the less interactive experience may have bearing on their choice of specialisation.
Moreover, telemedicine threatens to transform haematology specialist training. Unlike in North America and mainland Europe, the holistic UK training programme combines both laboratory and clinical aspects to produce haematologists who are both competent diagnosticians and astute clinicians. This dual training approach is undoubtedly a real strength of the UK system, not to be lost17. As such, it is crucial that a move away from hospital-based care does not inadvertently encourage a clearer dichotomy between the lab-based and patient-facing disciplines by making it tougher for haematologists to continue uniquely traversing both.
Yet in spite of these caveats, telemedicine may bring us tangibly close to multidisciplinary consultations for haematology patients, which would particularly revolutionise the care of those with complex health pictures: a patient with sickle cell disease with pulmonary hypertension and kidney involvement requiring input from haematology, cardiology and nephrology, for example. Multidisciplinary team intervention is known to significantly reduce emergency hospital admission for these complex patients18; how much more so if patients could be brought into the room where it happens. Though arranging for all specialists to be available at the same time will remain tricky, holding the consultation remotely makes this immeasurably more feasible.
CONCLUSION
Telemedicine represents a true paradigm shift in healthcare, offering much improved convenience, patient safety, and environmental sustainability. The advantages of its advent are clear, though increasingly obscured by significant caveats that are perhaps only coming to light as the heady excitement of the laudable technological triumph gives way to the realities. Having surely reached a tipping point, it would appear the question is no longer of returning to previous pre-pandemic practices, but embracing new ones and deciding which elements will remain: perhaps the most seminal being the unprecedented opportunity for multidisciplinary consultations, and a reconsideration of the risks deemed suitable for vulnerable haematology patients to take in order to access their care. Aside from the technicalities, further study will be necessary to elucidate the longer-term implications of a move away from in-person medicine for the patient-doctor relationship, and its personal toll on clinicians. The current evidence does, however, make emphatically clear that telemedicine must supplement, not substitute face-to-face care for haematology patients, not least in the absence of other safe and cost-effective measures to ensure that none fall through the net.
REFERENCES
- Stokel-Walker, C. Why telemedicine is here to stay.BMJ 371, m3603 (2020).
- Hammersley, V. et al. Comparing the content and quality of video, telephone, and face-to-face consultations: a non-randomised, quasi-experimental, exploratory study in UK primary care.Br J Gen Pract 69, e595-e604 (2019).
- British Society for Haematology. COVID-19 Vaccine in patients with haematological disorders. https://b-s-h.org.uk/media/19195/haematology-covid-19-v10-vaccination-statement-231220.pdf (Accessed on 28th January 2021).
- Willan, J., King, A. J., Hayes, S., Collins, G. P. & Peniket, A. Care of haematology patients in a COVID-19 epidemic.Br J Haematol 189, 241-243 (2020).
- Palandri, F. et al. Telemedicine in patients with haematological diseases during the coronavirus disease 2019 (COVID-19) pandemic: selection criteria and patients' satisfaction.Br J Haematol 192, e48-e51 (2021).
- Royal College of Physicians. Specialty spotlight - haematology. https://www.youtube.com/watch?v=TWtNWDs-lh0 (Accessed on 12th January 2021).
- Specht, D. Coronavirus: is working from home really better for the environment. https://geographical.co.uk/opinion/item/3653-is-working-from-home-really-better-for-the-environment-doug-specht (Accessed on 26th January 2021).
- Turits, M. Why working from home might be less sustainable. https://www.bbc.com/worklife/article/20200218-why-working-from-home-might-be-less-sustainable (Accessed on 26th January 2021).
- Taylor, J., Xiao, W. & Abdel-Wahab, O. Diagnosis and classification of hematologic malignancies on the basis of genetics.Blood 130, 410-423 (2017).
- Targeted leukaemis therapy: a chemotherapy-free future. The Lancet Haematology, 8, e1. (2021).
- Brenner, D. J. & Hall, E. J. Computed tomography--an increasing source of radiation exposure.N Engl J Med 357, 2277-2284 (2007).
- Hewitt, H., Gafaranga, J. & McKinstry, B. Comparison of face-to-face and telephone consultations in primary care: qualitative analysis.Br J Gen Pract 60, e201-12 (2010).
- Rimmer, A. How can I break bad news remotely. BMJ 369, m1876 (2020).
- General Medical Council. Remote consultations. https://www.gmc-uk.org/ethical-guidance/ethical-hub/remote-consultations (Accessed on 17th January 2021).
- Wise, J. Life as a haematologist. BMJ 368, m248 (2020).
- British Society of Gastroenterology. Rebooting gastroenterology and hepatology outpatients in the wake of COVID-19: renewal, redesign and establishing the “New Norm”. https://www.bsg.org.uk/wp-content/uploads/2020/07/Outpatient-services-restart-and-redesign-Ver-Final-2.pdf (Accessed on 22nd January 2021).
- Shlebak, A. A. & Bain, B. J. Training future haematologists, a privilege or a burden? "A trainer's view".Br J Haematol 178, 501-507 (2017).
- Powell, R. E. et al. A Multidisciplinary Approach to Impact Acute Care Utilization in Sickle Cell Disease. Am J Med Qual 33, 127-131 (2018).
When a novel pneumonia of unknown aetiology was announced in Wuhan, China on 31 December 20191 few could have imagined the scale of forthcoming change to many aspects of everyday life, including medical care. The coronavirus (SARS-CoV-2) pandemic has spurred several revolutions in modern medicine, perhaps one of the most significant being the large increase in the number of consultations delivered remotely, such that virtual interactions have now become the norm versus face-to-face appointments. Whilst initially this change was implemented in a matter of days in order to protect patients from community transmission of the virus causing COVID-19, it is likely to continue in some form in the future2. However, going forward there is a balance to be struck between safety, optimising patient care and increasing efficiency. This essay will discuss how the rapid transformation in service delivery has paved the way for a significant change in the specialty of haematology. It will firstly present a definition of telemedicine and how the pandemic has prompted its rapid incorporation into NHS care, explaining how this “jolt” catalysed a transformation already subtly in motion. Then, by explaining the shortfalls of telemedicine it will argue that there will continue to be a need to see at least some haematology patients face-to-face in the future, namely for inpatient care, breaking bad news and safeguarding.
Telemedicine or “healing at a distance” is a word often used to describe the practice of medicine where the patient and the practitioner are not in the same room. Although there is no official definition, the World Health Organisation3 describes it as:
“The delivery of health care services, where distance is a critical factor, by all health care professionals using … technologies for the exchange of valid information for diagnosis, treatment and prevention of disease and injuries, research and evaluation, … all in the interests of advancing the health of individuals and their communities”
In practice, telemedicine is often as simple as talking to a patient over the phone but can include video conferencing via software such as Attend Anywhere, Zoom or Facetime. For the purposes of this essay the term will be used to refer to synchronous interactions with a healthcare practitioner but some sources use it to refer to asynchronous advice sources, AI-based text messaging services or digital home monitoring4. Although not yet widely used in the UK, tools such as digital stethoscopes and remote vital sign monitoring devices allow a semblance of patient examination, promoting further devolution of healthcare from established locations to the patient’s own home5.
Pre-COVID systematic reviews of the palatability of telemedicine for patients and caregivers have found generally high levels of satisfaction, especially for specialist care. However, there is a lack of clarity on the definition and metrics of approval6. In addition, considering that before the pandemic the vast majority of people using telemedicine lived in rural locations (where there is often a dearth of specialist staff), the threshold for satisfaction is likely to be lower given that remote consultations avoid the often-significant costs and inconvenience associated with travelling long distances in order to access care. However, other reviews have found the methodology of most telemedicine studies to be low quality, which limits generalisability and applicability of findings7.
Before 2020, many healthcare providers were slow to embrace existing digital technologies, either due to lack of funds, regulatory restrictions or risk aversion8. However, social distancing measures and stay at home orders rendered traditional service delivery methods impossible and there was a vertiginous rise in the use of telemedicine. On 19 March 2020, NHS England issued a directive encouraging all care providers to “support provision of digital or telephone-based consultations wherever possible” and that face-to-face appointments should only occur when absolutely necessary5. Because the risk of patients contracting the virus in the clinical environment vastly overshadowed any potential pitfalls of remote consultations that would previously have been deemed unacceptable, the pandemic has provided an unprecedented opportunity for a radical shift in service provision. For haematology patients in particular, many of whom are considered clinically extremely vulnerable to COVID-19, there is a significant incentive to reduce attendances as much as possible9.
In the immediate future, it is likely that the primarily remote outpatient model will continue. Phase 3 of NHS England’s pandemic response specifically highlights how keeping outpatient services remote will allow footfall at clinical sites to remain reduced and protect other services that cannot be performed remotely2. Technology with proven value for distant communities is now significantly more relevant in an era of government-enforced social distancing.
As a medical speciality, certain aspects of haematology are particularly well suited to remote consultations. Many patients live with long-term, but stable conditions (e.g., chronic myeloid leukaemia) that never truly resolve, and they require regular check-ups and monitoring of their circumstances. Rather than having to arrange transport, pay for parking or have the potential for exposure to a communicable disease in the waiting room, remote consultations for these particular types of interactions are an efficient restructuring that should outlast the pandemic. The specialty also treats many younger patients living with long-term conditions such as haemoglobinopathies, haemophilia and thrombotic disease who are usually exceedingly technologically literate.
The 2019 Topol Review on the digital future of the NHS highlighted the benefits of information technologies for empowering patients to feel more involved in their own care4. It found significant evidence that appropriate use of pre-existing technology such as remote home monitoring and digital means of communicating with community teams can reduce hospital admissions and provide better outcomes for patients through early detection of deteriorating conditions. There are now a wide variety of apps available that can be used for reassurance, together with reminders to increase medication compliance in sickle cell disease10; as well as eDiary apps that have been shown to improve vital symptom tracking and record keeping in patients living with haemophilia by 23%11. Systematic use of these technologies across the healthcare sector should spur further research and development as well as reduce the number of inpatient stays.
However, even with recent advancements in digital technology, there are many areas of haematology that cannot be executed remotely. These include the majority of inpatient care, investigative procedures such as bone marrow aspirates and trephines, some chemotherapy treatment and blood transfusions. Even in an outpatient setting there are myriad things that become impossible in a telephone consultation, for example a comprehensive physical examination and a (sometimes subconscious) assessment of the patient’s overall wellbeing. It is difficult to replicate the subliminal intuitive clinical judgement practitioners develop from their years of experience seeing patients face-to-face. Non-verbal cues such as eye contact, body position and engagements, as well as signs such as pallor, bruising and weight loss, are almost entirely eliminated in telephone consultations and these subtleties contribute greatly to the overall clinical picture. The addition of video is not a panacea; it is often clumsy, with poor image quality and adds little to a consultation that would probably benefit from being face-to-face. Indeed, telemedicine fundamentally changes the tacit aspects of the doctor-patient relationship.
“Gestalt” is the term used to describe the ability of clinicians to build a comprehensive clinical picture that is greater than the sum of its constituent limited sources of information, i.e. history, examination and investigations12. Practitioners’ clinical skills and judgement are honed over many years of experience of providing in-person care and knowledge is handed down over generations through education. The rapid shift to remote outpatient consultations in 2020 renders experience developed over the previous centuries of practising medicine “traditionally” somewhat useless, as well as reducing opportunities for younger staff to develop their own clinical reasoning skills.
Speaking more practically, it is difficult to establish rapport – even over video conferencing – as patients also subconsciously pick up on non-verbal cues. It is vital to remember that remote consultations (and their pitfalls) are currently tolerated due to the pandemic, but there is little evidence as to how patients perceive “the new normal”. The literature already mentions the difficulties of breaking bad news in a remote consultation13, with poor connections making it difficult to appear empathic and communicate clearly, which are essential qualities in holistic care. Another significant issue with reducing the number of face-to-face patient interactions in haematology is diminished opportunities for safeguarding vulnerable individuals. Recorded rates of neglect, family violence and abuse have skyrocketed during periods of social-distancing measures14 and medical appointments are often a safe space where individuals can talk freely, seek advice and access necessary services. In short, it is much trickier for professionals to utilise their “soft skills” with telemedicine.
Perhaps the largest stumbling block to never seeing haematology patients face-to-face again is the issue of access to technology. Although four out of five adults in the UK own a smartphone8, not all patients have access or a working knowledge of the necessary devices, sufficient data allowances or quiet, private space. This is often described as digital poverty and is more prevalent in lower socio-economic groups15, which are already more likely to have poor health outcomes16. Therefore, it is essential that we strive for equity in healthcare delivery so as not to exacerbate pre-existing health inequalities due to socioeconomic status. Confidentiality is also a significant issue. Not only should patients not be overheard within their own household, but they should feel that their personal information sent over the internet is secure. There are also serious ethical questions to be asked about collection of patient data by for-profit organisations. Many NHS trusts relaxed information governance rules temporarily at the beginning of the pandemic in order to maximise the speed of transition to remote working5, and a comprehensive solution to multifaceted privacy issues is yet to be found4.
Finally, if remote consultations become the norm across the whole NHS, work must be done to reduce the number of late presentations of serious illness. Although some can be attributed to patients’ fears of contracting COVID-19 whilst seeking care, two-week-wait cancer referrals fell by 84% in April 2020 and models predict significant reductions in ten year survival from just a three-month delay in treatment17. Anecdotal evidence suggests this is partly due to GP’s reduced ability to get a clear picture of a patient’s condition but also due to deferral of routine appointments due to social distancing2. What is certain however, is that the impacts of these decisions will only become more apparent with time. If seeing haematology patients face-to-face in the future is reduced, more evidence is needed on how to best detect subtle clinical information.
The opportunities possible from sustained investment of time and resources into effective telemedicine systems are almost infinite. One particularly of relevance to the NHS is a blurring of the boundaries between primary and secondary care, allowing GPs and other allied health professionals to manage long-term conditions in the community. Fogel and colleagues use the term “e-consult” to refer to practitioner-practitioner digital interactions used to seek advice on how to best assist a particular patient. Studies have shown that effective use of e-consults in primary care can reduce unnecessary in-person appointments, with one in particular suggesting 66% of haematology e-consults could be adequately managed without the patient needing a face-to-face meeting with a specialist18. E-consults can also be used within hospitals to allow remote specialist input on a particular patient’s case. Even if complex restructuring remains difficult to achieve at present, simpler measures such as allowing patients to have their blood tests performed at their local practice, with the results sent electronically to their hospital consultant are an efficient, patient-centred reallocation of resources. In the same way that NHS trusts provide transport to allow patients to reach hospital, it is not difficult to imagine community outreach teams to ensure that all patients have access to confidential facilities or the necessary technology to effectively utilise telemedicine services.
However, given the polemic nature of wider structural reforms to the NHS in recent years 19, it is essential that decisions are made with patients’ (rather than financial) interests at the forefront. Sacrifices in quality of patient care mentioned previously that were deemed necessary during periods of high coronavirus transmission should not become normalised when the opportunity to resume face-to-face interactions returns. There are many private companies seeking to benefit from this rapidly expanding marketplace who expound the advantages of telemedicine8, but something more substantial than marketing material is needed to make informed commissioning choices. A general trend in the literature is the lack of empirical data to make evidence-based decisions about the use of telemedicine and how to design systems effectively20. It is impossible and foolhardy to make rational decisions in the eye of the storm.
The COVID-19 pandemic has called fresh attention to the stark health inequalities that exist within the UK2, i.e. the systematic and avoidable differences in health and wellbeing that arise due to lifestyle factors beyond one’s control. Marginalised groups – particularly those with BAME heritage – have been disproportionately affected by the health, economic and social consequences of almost a year of restrictions and are more likely to live and work in conditions that increase exposure and vulnerability to SARS-CoV-2. The importance of an intersectional approach that prioritises inclusivity, fairness and innovation cannot be overstated. Sustainable redesign of the health service in the aftermath of the coronavirus’ devastating effects needs to take an equitable approach towards disenfranchised communities, particularly those without smartphone or internet access, who will suffer under a model that restricts access to face-to-face care. Importantly, there is significant evidence to suggest that sustained (albeit costly) investment in improved education, employment and housing notably decreases unfair and avoidable inequalities which would lower long-term costs to the NHS through improved health outcomes16.
In conclusion, the COVID-19 pandemic has revolutionised delivery of healthcare across the globe, such that face-to-face interactions with patients are far less common. It is likely that widespread use of telemedicine is here to stay for the foreseeable future. However, there are serious shortcomings to remote consulting including hampered empathetic communication, reduced opportunities for safeguarding vulnerable individuals and diminished ability to utilise clinical gestalt developed through years of experience and training. With an ethos of patient-centred and equitable provision, post-COVID restructuring could lead to improved experience for patients and enhanced health outcomes. Increased and systematic use of home-monitoring technologies and apps across the healthcare sector should also increase technology literacy rates, potentially allowing increased patient education and improving patient-centred care. Better patient engagement in their own care via accessible and existing technology could even result in fewer face-to-face interactions if hospital admissions can be avoided with pre-emptive management in the community.
The pandemic has catalysed a fundamental change to the centuries-old idea of “going to the doctor” Although the current situation may appear bleak, we are at a crossroads where there is a chance to use the last year as an impetus for viable, sustainable change in order to build an equitable health service for future generations, with patient-centred care at the heart of all decisions.
References:
- WHO. Pneumonia of unknown cause - China. (2020). Available at: https://www.who.int/csr/don/05-january-2020-pneumonia-of-unkown-cause-china/en/. (Accessed: 29th December 2020)
- NHS England. Implementing phase 3 of the NHS response to the COVID-19 pandemic. (2020).
- WHO. Telemedicine: Opportunities and Developments in Member States. (2010).
- Topol, E. Preparing the healthcare workforce to deliver the digital future . (2019).
- Fisk, M., Livingstone, A. & Pit, S. W. Telehealth in the context of COVID-19: Changing perspectives in Australia, the United Kingdom, and the United States. J. Med. Internet Res. 22, (2020).
- Orlando, J. F., Beard, M. & Kumar, S. Systematic review of patient and caregivers’ satisfaction with telehealth videoconferencing as a mode of service delivery in managing patients’ health. PLoS One 14, (2019).
- Eze, N. D., Mateus, C. & Hashiguchi, T. C. O. Telemedicine in the OECD: An umbrella review of clinical and cost-effectiveness, patient experience and implementation. PLoS ONE 15, (2020).
- EY. Embracing digital in health and social services. (2021). Available at: https://www.ey.com/en_gl/government-public-sector/embracing-digital-is-covid-19-the-catalyst-for-lasting-change. (Accessed: 30th January 2021)
- Weinkove, R. et al. Managing haematology and oncology patients during the COVID-19 pandemic: interim consensus guidance. Med. J. Aust. 212, 481–489 (2020).
- Hankins, J. S. & Shah, N. Tackling adherence in sickle cell disease with mHealth. Lancet Haematol. 7, e713–e714 (2020).
- Banchev, A. et al. Impact of Telemedicine Tools on Record Keeping and Compliance in Haemophilia Care. Hamostaseologie 39, 347–354 (2019).
- Kienle, G. S. & Kiene, H. Clinical judgement and the medical profession. J. Eval. Clin. Pract. 17, 621–627 (2011).
- Wolf, I., Waissengrin, B. & Pelles, S. Breaking Bad News via Telemedicine: A New Challenge at Times of an Epidemic. Oncologist 25, e879 (2020).
- Usher, K., Bhullar, N., Durkin, J., Gyamfi, N. & Jackson, D. Family violence and COVID-19: Increased vulnerability and reduced options for support. International Journal of Mental Health Nursing 29, 549–552 (2020).
- Watts, G. COVID-19 and the digital divide in the UK. Lancet Digit. Heal. 2, e395–e396 (2020).
- Marmot, M. & Wilkinson, R. G. Social determinants of health. (Oxford University Press, 2006).
- Sud, A. et al. Effect of delays in the 2-week-wait cancer referral pathway during the COVID-19 pandemic on cancer survival in the UK: a modelling study. Lancet Oncol. 21, 1035–1044 (2020).
- Fogel, A., Khamisa, K., Afkham, A., Liddy, C. & Keely, E. Ask the eConsultant: Improving access to haematology expertise using an asynchronous eConsult system. J. Telemed. Telecare 23, 421–427 (2017).
- Furse, J. The NHS Dismantled. London Review of Books (2019). Available at: https://www.lrb.co.uk/the-paper/v41/n21/john-furse/the-nhs-dismantled. (Accessed: 29th December 2020)
- Fouquet, S. D. & Miranda, A. T. Asking the Right Questions—Human Factors Considerations for Telemedicine Design. Current Allergy and Asthma Reports 20, (2020).
In 2020 the essay title was 'How are you inspired by haematology and how will you inspire others to become haematologists?'. The winner was Louis-Pierre Girard from the University of Aberdeen and the runner-up Joanna Parsons from Barts and the London School of Medicine and Dentistry.
‘Do you know what specialty you want to do?’
This is a question that all medical students and junior trainees are accustomed to being asked on a regular basis. Inquiring parties include family members, friends, members of the public, as well as members of the healthcare profession at all levels. When I am asked, I always reply ‘I would quite like to do haematology’. Non-medical individuals are almost always baffled and befuddled, whilst my peers are either awestruck or horrified. Meanwhile, medical personnel frequently appear blindsided, and usually quite surprised - amusingly, this also often includes haematologists themselves. Without fail, I am asked either what haematology is, or why I want to do it. The former is easy to answer, but offering a succinct answer to the latter is a much trickier challenge.
A Fluid Harmony: Intellectual Elegance
Medicine can be a complex and self-contradictory subject, to the great chagrin of all medical students. The fundamental principles of haematology, meanwhile, are refreshingly simple, and are wonderfully easy to grasp when approached with a clear mind. Blood has four principal components: red cells, white cells, platelets and plasma. In turn, these components serve three simple functions: transportation, self-defence, and maintenance of vascular integrity. In health, these components are created and destroyed effectively and harmoniously. In illness, they can either increase, decrease, or suffer impaired function - invariably due to errors or imbalances in their creation and destruction.
This knowledge, complemented by a thorough history and physical examination, and supplemented by a basic grasp of cell lineage and cell function, can be used to successfully tackle the vast majority of basic haematological problems. This begins with the successful interpretation of full blood counts and blood films, continues thereafter with the choosing of further appropriate investigations, and culminates in the implementation of the treatment required to address the patient’s fundamental problem. The simple and elegant logic which underpins all of haematology allows the subject to almost entirely unfold itself before a novice’s eyes and blossom into a very natural, fundamental understanding of blood, including its various forms, functions and modes of failure.
This is perhaps contrasted against the deeper waters of haematology, where classifications of diseases and their various subtypes seem, at first, to multiply outwards at an exponential rate, and for which the treatments can vary significantly based on extremely specific criteria. Simultaneously, we are introduced to the multitudes of otherwise unusual tests that proliferate within haematology, including immunophenotyping, immunohistochemistry, electrophoresis, karyotyping, in addition to tests for various specific mutations. These techniques are all much more familiar to academic and biomedical scientists, with whom clinical haematologists intimately share their professional practice. After spending many years learning the language of medicine, it is not surprising that so many find the haematological dialect jarring and alienating: We speak of CD numbers, minimal residual disease, T cell subsets and double-hit lymphomas. These are not things we are acquainted with during medical school.
This intellectual mist, as perceived by medical students and other medical professionals, highlights two essential aspects of haematology. Firstly, that few other specialists are so closely knitted to the scientific process as the practicing haematologist, for whom the clinical playing field is in a near-constant state of flux. Secondly, it reflects the differences in thought and approach of the practicing haematologist, when compared to his or her general medical colleagues. Where respiratory physicians might sling a stethoscope, or where neurologists tender their tendon hammer, the haematologist will return always to their microscope. Where other specialists make brilliant inferences, the haematologist simply bears witness to the cells beneath their gaze.
These essential differences cause haematology to stand apart from the many medical specialties which chart the course of medical students’ developing powers of clinical reasoning. Navigating haematology’s choppy sea requires different bearings. Though others may feel nervous when faced with the idea of learning a ‘new’ mindset for unfamiliar diseases, admittedly having already devoted many years to learning the topsy-turvy world of clinical medicine, it need not be so. Many trainees who are introduced to haematology, and given time by their superiors to test its waters, are inspired to set sail in this new direction they have discovered. This is evidenced by the number of consultants who, when asked what drew them to take this path, all recount the same tale of being charmed by the innate and graceful logic which suffuses the academic study of blood (Bowles, K 2015).
From the Front to the Frontiers: A Pioneering Spirit
Blood has been a source of inspiration and intrigue to humanity since the dawn of our time. Perhaps the reason for this special regard is because blood is omnipresent in the human body, and thus is easily revealed and sampled. And yet, despite the ease with which it can be obtained, the scientific study of blood remains a young subject. The first haematologists to differentiate themselves from their general physician and pathologist colleagues did so in 1931, in France, with the formation of the French Society of Haematology (McCann, 2016). Across the English Channel, our own British Society for Haematology was established later, in 1960 (McCann, 2016). Since these times, the practice of haematology and its impact on all of medicine has been colossal and revolutionary.
At the time of writing, there is enormous global hubbub in the private sector about the industrial upscaling of CAR-T cell therapy, and the subsequent cellular therapy revolution that this would supposedly herald (Nam, Smith and Yang, 2019). This might give the impression to otherwise uninformed parties that cellular therapy is a new and modern breakthrough, which is false. The first large scale, therapeutic use of cells came in the form of blood transfusion, whose widespread use was implemented and codified in the vicious Spanish Civil War (1936 – 1939) by doctors Norman Bethune and Frederick Durán-Jordá (McCann, 2016). Whilst blood transfusion was largely neglected in the preceding First World War (1914 – 1918), the Second World War (1939 – 1945) became the proving ground on which the mass use of this novel therapeutic procedure was consolidated, subsequently saving countless lives (King, 1965).
Thereafter, the therapeutic use of cells marched onwards with the leap from transfusion to transplantation, beginning with clinical observations of acute radiation sickness in atomic bomb survivors in the Japanese city of Hiroshima (DeCoursey, 1948). Therapeutically harnessing the myeloablative and cytotoxic properties of ionizing radiation, American research teams devised and advanced bone marrow transplantation as a process to rescue patients from otherwise irreversible and lethal bone marrow failure (Blume and Thomas, 2016). Only in 1988 did haematologists identify the graft vs leukaemia effect propagated by bone marrow or haematopoietic stem cell transplant, which we now identify as the key process underlying successful transplantation, and the cure from cancer it entails (Horowitz et al., 1990). Thus, rather inadvertently, bone marrow transplantation has been the progenitor of cell-based immunotherapies, and the technologies and discoveries pioneered by its practitioners have paved the way for the current cell-therapy revolution we all eagerly anticipate.
The therapeutic use of cells, however, is only one aspect of the multifaceted approach taken by haematologists towards the goal of thwarting blood disease. Though cytotoxic chemotherapy has its origins in the antimicrobial use of arsenic, it has since evolved to include antineoplastic therapies. Careful observation of the myeloablative properties of mustard gas, weaponised in the First World War led to the creation of cyclophosphamide - a drug which continues to be a cornerstone in the treatment of aggressive lymphoma (Pfreundschuh et al., 2006). In recent years, it has enjoyed a renaissance in stem cell transplantation, due to its powerful negation of potentially lethal graft versus host disease (Kanakry, Fuchs and Luznik, 2016). Also of note, the specific development of the folate antagonist methotrexate for use in childhood leukaemia marked the first instance of intelligent drug design (Bryan, 2012).
The ability of haematologists to harness and repurpose the world around them, based on insightful and clear-minded observations, has continued with numerous momentous advancements in recent history: The identification of all-trans retinoic acid as a near-curative therapy for acute promyelocytic leukaemia (Huang et al., 1988), the propagation of targeted inhibitors such as imatinib which have more than doubled the survival of patients with chronic myeloid leukaemia (O’Brien et al., 2003), and the grand success of monoclonal antibody therapy, embodied by rituximab (Pfreundschuh et al., 2006). Most recently, the United States Food and Drug Administration granted approval to voxelotor - the first haemoglobin oxygen-affinity modulator – for use in patients with sickle cell disease (FDA, 2019).
The success of these advancements has not only revolutionised and transformed the lives of haematological patients, but have also been either directly transferred for use in patients with non-haematological diseases, or have otherwise helped lay the foundations on which subsequent therapies for these patients have been developed. In these ways, due to the nature of blood and the inquisitive personalities of those who study it, haematology has helped to pioneer revolutions not only in the treatment of blood disease, but for all of medicine. As a student, learning about this rich history, often from individuals who directly witnessed or propagated these events, is thrilling and motivating, and should encourage everyone to reflect and consider what they can contribute to this still blooming field.
Through the Mist: Engendering Inspiration
When inspiring young students, scientists and doctors, I believe the greatest challenge is helping them ford the chasm which appears to separate the fundamental principles of haematology from the higher practice of haematology. One of the least inspiring responses I have regularly heard from haematologists, when I have been attempting to make this crossing, is that ‘It’s unnecessary for medical students to know this’. I have heard the same uttered by oncologists. I firmly believe this to be completely false. I have been most fortunate and grateful to be tutored by a number of haematologists, all fantastic educators, who succeeded precisely because they demanded I learn more than required, and because they actively supported this learning. Indeed, all the knowledge I have accrued under their tutelage will certainly never be tested in any medical school exam, but without it, I would never have grasped my current full-blooded understanding of the processes which underlie the diagnosis, classification and management of the multitudinous haematological malignancies, nor the practical subtleties of clotting and thrombosis.
The demystification of our subject is, and will continue to be, the bedrock of my approach to inspiring others towards haematology, and I have been greatly satisfied by my experiences in educating and inspiring young clinical research interns from polytechnic schools, as well as helping peers now working as junior doctors in haematology units. To cite a recent example, I was all too pleased to discuss with a freshly graduated colleague - now working in a haematology unit - why a patient with chronic low blood counts in the context of longstanding myelodysplastic syndrome might be handled with an altogether different level of urgency, when compared to another patient receiving very intensive induction chemotherapy for the treatment of acute leukaemia. Our discussion was a fruitful and satisfying experience for both parties. This particular colleague had historically always found haematology to be an inscrutable subject.
Building on this demystification, my next approach is to encourage young student doctors or scientists to spend dedicated time in haematology units and labs. As students we devote justifiably significant amounts of time on such subjects as internal medicine, general surgery and general practice. Other specialty subjects, such as haematology, oncology, obstetrics, gynaecology, radiology and orthopaedics, do not benefit from as much dedicated time (Mandan, Sidhu and Mahmood, 2016). For the most part, this can be overcome through study. Not so for haematology - bones and babies are all better grasped than bone marrow. Over the course of my medical studies, I have been fortunate to spend twenty weeks on haematological placements, largely at the expense of my personal time. The understanding of haematology I may have gleaned over that of my colleagues is borne out of the time I have spent on these placements.
Despite my overtures to them, my colleagues never heeded my suggestion to directly inquire to haematologists about extra-curricular attendance at clinics. Perhaps, therefore, the ideal time to light the fires of haematology in young staff is when they pass through haematology units and laboratories on rotation (Mandan, Sidhu and Mahmood, 2016). At these key junctions, they have the opportunity to gain the experience required to gather a solid grasp on the daily practice of haematology, including the unique vocabulary and methodology that accompany it. The vast majority of haematologists I have encountered have also tended to be very friendly, and enjoy the instruction of their subject. They are perfectly positioned to educate rotating junior doctors, and help them cross the perceived gap between the basic principles and the daily practice of haematology.
Furthermore, acquainting junior doctors with common haematological diagnostic tools, and encouraging them to learn and practice the basic interpretation of the generated results would be effective at narrowing the perceived mystery that normally surrounds them (Di Carlofelice and Cope, 2016). I am reminded of non-medical friends peeping over my shoulder as I revise chest x-ray interpretation: despite their lack of knowledge of chest anatomy and pathology, their perceived familiarity with chest x-rays meant that they were quite happy to proffer their guesses at the diagnosis. This confidence is not replicated when I am revising blood films or reading about the immunophenotyping of indolent non-Hodgkin lymphomas. But by outlining a basic approach to such things, as it was outlined to me by my teachers, their confidence is quickly restored, and they return once more to their diagnostic conjecture.
The final key aspect of haematology, to which all trainees can relate, is the patients. The close bonds developed between haematologists and their patients, over the course of long and arduous courses of treatment, including prolonged stays in hospital, can only be experienced by spending significant time on a haematology unit. This aspect, in combination with the willingness of patients and consultants to soldier on against the terrible illnesses they are facing, has been deeply moving for many junior doctors I have shadowed. Life for patients and doctors on haematological units makes for a dramatic landscape, both punishing and rewarding in its emotional demands. At its highest peak, there is the clean air of cure, and at its lowest trough is the indignity of death. That haematologists should be so determined in their attempts to shepherd their patients towards a cure can only be admirable, and is justified both in respect towards patients’ autonomy, and in respect to the ever-rising rates of cure which we are achieving for these patients (McDonald et al., 2020).
Conclusion
For myself, I have been inspired by a tirelessly committed, and highly talented community who, through decades of devoted and determined toil, have instigated a medical revolution that has barely begun, by drawing on a well of imagination and observation, whose source has a reach as wide as two World Wars, and as deep as the DNA that underpins life itself. If we wish to inspire others to join us on this course, I believe we need only to show them the way.
Bibliography
Blume, K.G. & Thomas, E.D. 2016, "A History of Allogeneic and Autologous Hematopoietic Cell Transplantation" in Thomas' Hematopoietic Cell Transplantation, eds. J.S. Forman, R.S. Negrin, J.H. Antin & F.R. Appelbaum, 5th edn, John Wiley & Sons, Chichester, pp. 1.
Bowles, K. 2015, June 1st-last update, Specialty spotlight – haematology [Homepage of Royal College of Physicians], [Online]. Available: https://www.rcplondon.ac.uk/education-practice/advice/specialty-spotlight-haematology [2020, 02/01].
Bryan, J. 2012, "From cancer to rheumatoid arthritis treatment: the story of methotrexate", The Pharmaceutical Journal, vol. 289, no. 303.
DeCoursey, E. 1948, "Human pathologic anatomy of ionizing radiation effects of the atomic bomb explosions.", The Military Surgeon, vol. 102, no. 6, pp. 427.
Di Carlofelice, M.A. & Cope, J.F. 2016, "Undergraduate hematology - a more integrated approach.", Advances in Medical Education and Practice, vol. 7, pp. 643.
Horowitz, M.M., Gale, R.P., Sondel, P.M., Goldman, J.M., Kersey, J., Kolb, H.J., Rimm, A.A., Ringden, O., Rozman, C. & Speck, B. 1990, "Graft-versus-leukemia reactions after bone marrow transplantation. ", Blood, vol. 75, no. 3, pp. 555.
Huang, M.E., Ye, Y.C., Chen, S.R., Chai, J.R., Lu, J.X., Zhoa, L., Gu, L.J. & Wang, Z.Y. 1988, "Use of all-trans retinoic acid in the treatment of acute promyelocytic leukemia. ", Blood, vol. 72, no. 2, pp. 567.
Kanakry, C.G., Fuchs, E.J. & Luznik, L. 2016, "Modern approaches to HLA-haploidentical blood or marrow transplantation.", Nature reviews. Clinical Oncology, vol. 13, no. 1, pp. 10.
King, L.S. 1965, "Blood Program in World War II. Medical Department, United States Army", JAMA, vol. 191, no. 11, pp. 954.
Krumbhaar, E.B. & Krumbhaar, H.D. 1919, "The Blood and Bone Marrow in Yelloe Cross Gas (Mustard Gas) Poisoning: Changes produced in the Bone Marrow of Fatal Cases.", Journal of Medical Research, vol. 40, no. 3, pp. 497.
Mandan, J., Sidhu, H.S. & Mahmood, A. 2016, "Should a clinical rotation in hematology be mandatory for undergraduate medical students?", Advances in Medical Education and Practice, vol. 7, pp. 519.
McCann, S.R. 2016, "The Enlightenment and the Unravelling of the Circulation" in A History of Haematology: From Herodotus to HIV, 1st edn, Oxford University Press, Oxford, pp. 17-26.
McCann, S.R. 2016, "The Same Specialty - but Different Approaches!" in A History of Haematology - from Herodotus to HIV, 1st edn, Oxford University Press, Oxforf, pp. 169-171.
McDonald, G.B., Sandmaier, B.M., Mielcarek, M., Sorror, M., Pergam, S.A., Cheng, G.S., Hingorani, S., Boeckh, M., Flowers, M.D., Lee, S.J., Appelbaum, F.R., Storb, R., Martin, P.J., Deeg, H.J., Schoch, G. & Gooley, T.A. 2020, "Survival, Nonrelapse Mortality, and Relapse-Related Mortality After Allogeneic Hematopoietic Cell Transplantation: Comparing 2003-2007 Versus 2013-2017 Cohorts.", Annals of Internal Medicine, , pp. doi: 10.7326/M19-2936.
Nam, S., Smith, J. & Yang, G. 2019, "Driving the Next Wave of Innovation in CAR T-cell Therapies", McKinsey & Company, [Online], vol. December, , pp. 30/01/2020. Available from: https://www.mckinsey.com/industries/pharmaceuticals-and-medical-products/our-insights/driving-the-next-wave-of-innovation-in-car-t-cell-therapies#.
O'Brien, S.G., Guilhot, F., Larson, R.A., Gathmann, I., Baccarani, M., Cervantes, F., Cornelissen, J.J., Fischer, T., Hochhaus, A., Hughes, T., Lechner, K., Nielsen, J.L., Rousselot, P., Reiffers, J., Saglio, G., Shepherd, J., Simonsson, B., Gratwohl, A., Goldman, J.M., Kantarjian, H., Taylor, K., Verhoef, G., Bolton, A.E., Capdeville, R., Druker, B.J. & IRIS Investigators 2003, "Imatinib compared with interferon and low-dose cytarabine for newly diagnosed chronic-phase chronic myeloid leukemia.", New England Journal of Medicine, vol. 348, no. 11, pp. 994.
Pfreundschuh, M., Trümper, L., Osterborg, A., Pettengell, R., Trneny, M., Imrie, K., Ma, D., Gill, D., Walewski, J., Zinzani, P.L., Stahel, R., Kvaloy, S., Shpilberg, O., Jaeger, U., Hansen, M., Lehtinen, T., López-Guillermo, A., Corrado, C., Scheliga, A., Milpied, N., Mendila, M., Rashford, M., Kuhnt, E., Loeffler, M. & MabThera International Trial Group 2006, "CHOP-like chemotherapy plus rituximab versus CHOP-like chemotherapy alone in young patients with good-prognosis diffuse large-B-cell lymphoma: a randomised controlled trial by the MabThera International Trial (MInT) Group.", Lancet Oncology, vol. 7, no. 5, pp. 379.
United States Food and Drug Administration 2019, November 25th, 2019-last update, FDA approves voxelotor for sickle cell disease [Homepage of United States Food and Drug Administration], [Online]. Available: https://www.fda.gov/drugs/resources-information-approved-drugs/fda-approves-voxelotor-sickle-cell-disease [2019, 02/02].
Much like the elusive stem cell, doctors undergo a sequential process of differentiation until they find their niche, their area of medicine they have decided to specialise in. After four, five or six years in medical school and two years as practising foundation doctors, doctors gradually narrow down their options and the direction of their future career, until they are fully committed as a specialist in a particular field of medicine. In the UK there are over 60 recognised specialities to choose from1, so how do you possibly make a choice? And the right choice at that?
Understanding factors that influence decision making is key to understanding why decisions are made. In terms of choosing a medical specialty, factors that influence your choice of specialty can be both practical and emotive. Practical reasons can include your domestic circumstance, the numbers of hours you’re expected to work, the conditions under which you work, the pay and the geographical location of the job. However, studies have consistently demonstrated that the most significant influencing factors for both undergraduates and trainees is exposure and positive experience of a speciality2,3 or academic interest in a speciality, for which exposure is one of the main driving forces4, whilst factors such as lifestyle, financial rewards and location take a backseat and rank as less important3. Further to this, a number of studies have stated that nearly half of all doctors go on to pursue the careers they identified as their primary choice in medical school2,3,5. When considered together, these studies demonstrate the importance of early positive exposures to any given specialty when it comes to influencing future career decisions.
What inspired me?
A career in medicine doesn’t simply start on your first day as a foundation doctor. It starts many years before, when you, most commonly as a naive adolescent, choose a career you want to do for the rest of your life. When you base you’re GCSE and A-level choices on the ones you know you need to get into medical school, when you plan extracurricular activities because you know they’ll be desirable on your application for medical school, when you devote extra hours to studying than all your friends because you know you have to do well to get into medical school. And at this time in your life you say ‘I want to study medicine and I want to become a paediatrician’, or ‘I want to become a cardiologist’, or ‘I want to become a dermatologist or a plastic surgeon’. How rare is it to hear someone of this age tell you they want to become a haematologist? Indeed, questionnaires have proved its rarity, with pathology, the branch under which haematology finds itself, ranking amongst the least popular specialties medical school applicants wish to pursue after medical school6. Of course, there are many different factors at play here, the most obvious being that haematology is a small and relatively little-known speciality compared to the likes of a glamorous career as a cardiothoracic surgeon and its perceived complexity means that it is often not considered.
As a current medical student, I have not even reached fully fledged totipotent stem cell status yet, I remain entirely unqualified and uncommitted to any speciality. However, as a medical student, you are placed in a unique position whereby you are able to observe a wide variety of doctors and healthcare professionals when changing from one specialty to another and switching hospitals every other week. On your best days in hospital or general practice, you come away thinking ‘this is exactly the kind of doctor I want to be’ and on your worst days you have learnt exactly the kind of doctor you don’t want to be. That feeling of wanting to be able to imitate what a great communicator, teacher, scientist or whatever value you recognised in that person you’ve observed, is inspiration and it is exactly what I experienced on a week long haematology placement in my 3rd year at medical school and, I imagine, exactly the positive experience they are talking about in the research into influential factors on speciality choice.
The haematology seed was first planted for me as I observed a Professor of Haematology whilst he ran a paediatric haemophilia clinic at the Royal London hospital. What I valued the most during this afternoon clinic was that in between patients, the Professor took time to explain to me in detail the ins and outs of haemophilia and the trial he was running at the trust, which after some further reading turned out to be a really quite extraordinary study. Perhaps after six months of placements, my expectation for how senior doctors tended to treat medical students on their firms was low, largely owing to the fact that busy doctors working in an often overstretched system rarely had the time or energy to take much notice of a lurking medical student. However, I was so in awe of the research he had been conducting and how he had gone to such lengths to explain this to me in his busy afternoon clinic, that it was then that I first thought perhaps haematology is quite interesting, perhaps I should consider haematology. For many junior doctors and medical students deciding their career pathway, haematology might seem a slightly obscure subject but now when people ask me what kind of doctor I want to be, which they do, a lot, I say ‘Well, I think I want to be haematologist’. Of course, nothing is set in stone and there will be many opportunities for me to change my mind, but this was the first time since I decided I wanted to study medicine that I had been able to give a confident answer to that question.
The research the professor was talking about in his clinic happened to have been recently mentioned in the news in December of that year (2017), it was, of course, the gene therapy trials for severe haemophilia A and the exceptional results it had already achieved, surpassing all original expectations for the study. To begin with, gene therapy for Haemophilia A, rather than B, seemed unattainable, as the factor VIII gene was much bigger than that of factor IX and so, more difficult to package in the small designated vector, adeno-associated virus (AAV) and in fact prior to this study three previous attempts of gene therapies for haemophilia A had already been deemed unsuccessful. The aim for the first in-man haemophilia A gene therapy trial was to achieve a modest activity level of >5 IU per decilitre, however, the phase 1-2 trials with a single infusion of AAV5-hFVIII-SQ resulted in six patients (out of nine) achieving a factor VIII level in, or even over, the normal physiologic range of 50-150 IU per decilitre at one year post-gene transfer7! Now, three years on from when results of the trial of gene therapy were first published, a follow-up study has been made available showing that seven of the original nine had a median factor VIII expression of 20 IU per decilitre but most importantly they still had an average number of annual bleeding events of 0 and an average of 0 infusions of factor concentrate annually8. And so as one inspiring achievement has a tendency to raise the sense of possibility in others, it is hard to ignore the value and the importance of this research as it pioneers the way for future gene therapies and inspires a new generation of haematologists.
Haemophilia treatment is a glittering example of the success of modern medicine, having evolved from a disease that could kill you by your 10th birthday, to one where patients can expect a near normal life expectancy, providing an early diagnosis and adequate prophylactic therapy. And now, gene therapy is offering a different narrative all together as patients are faced with the very real potential for a complete cure in the not so distant future. It is also a disease, however, where past interventions have been fraught with controversy and catastrophe. The 1970s saw the start of what is now thought of as the modern era for haemophilia treatment with the introduction of lyophilised plasma concentrates of coagulation factors VIII and IX9 from pooled plasma which eventually led to a primary prophylactic regimen for haemophiliacs with the aim of preventing the number of spontaneous bleeds and their disabling consequences10. However, the discovery of plasma concentrates to treat haemophilia in the 1970s was superseded and overshadowed by the realisation that these blood products which had been created by pooling thousands of donors’ blood, were contaminated and were the source of viral infections such as the fatal contagion HIV, Hepatitis B and the Hepatitis C virus. Subsequently, it was estimated that between 60-80% of patients who were exposed to factor concentrate between 1979 and 1984 then tested seropositive to HIV11 and according to public health estimates in the UK, 71% of Haemophiliacs infected with the HIV virus are now dead12. It was described as one of “the worst disasters in the history of the NHS” and so it seems only right that in a wonderful twist of karmic fate this population of long-suffering patients now have the potential to access to curative treatments in the near future that may relieve them of haemophilia all together.
Intellectual Stimulation
As demonstrated with the above example of haemophilia, the understanding of the aetiology of haematological conditions and the advancement of treatments is moving at a phenomenal rate and has transformed the practice of haematology over the last few decades. This makes haematology a particularly attractive career for anyone interested in academic medicine. The way in which haematological research has flourished over the years, especially with the advent of molecular medicine has made huge impacts on clinical care. Such advances were demonstrated brilliantly at the beginning of the century when Imatinib was introduced as a treatment for chronic myeloid leukaemia after having discovered a few years earlier that the Philadelphia chromosome was the abnormality responsible for the disease13. Imatinib was established as the first member of a group of cancer drugs called targeted therapies, quickly followed by Rituximab, an anti-CD20 which revolutionised treatment for non-Hodgkin’s lymphoma14. Since then, an explosion of targeted therapies has ensued, with varying degrees of success admittedly, but each opening new avenues for exploration in the treatment of the most complex and resistant of haematological cancers. Such advances have given haematologists the tools to actually alter the course of disease for many – the core purpose of your role as a doctor and the reason why many of us choose to become doctors, no?
Another of the most attractive aspects of a career in medicine generally, is the logical challenge of evaluating, examining and investigating a patient to determine the precise nature and severity of their underlying problem and subsequently deciding on the best course of treatment. In most specialities it would be rare, if not unheard of, to be involved in each step of this process, from clinic, to performing and interpreting diagnostic tests in the laboratory, to clinic again and initiation of treatment, however, haematologists, due to their unique role that allows them to incorporate laboratory medicine with cutting edge clinical medicine, are granted the enviable position of being closely involved in each step, becoming the ultimate medical detectives.
Lastly blood’s essential nature is pervasive, it is a requisite to the healthy function of every single organ and tissue in the human body and it is the liquid life force that maintains us. In short, blood is everywhere, making the role of a haematologist extremely varied and in turn creating many sub-specialties within the context of the wider specialty such as paediatric haematology, obstetric haematology, haemato-oncology and transfusion medicine. If a career in a branch of medicine that is moving at an incredible pace with great opportunity and scope for academic advancement set alongside wide clinical variety doesn’t excite and inspire, than I’m not sure what will.
What can inspire others?
A role model is defined as a person looked upon by others as an example to be imitated. Evident in my own personal experience was the influence of a perceived role model who created the positive exposure to an otherwise obscure speciality choice. Earlier we discussed how evidence shows that this is one of the main drivers behind a doctor’s choice of speciality and additional studies have specifically linked exposure to role models in a particular medical field with an increased odds ratio for choosing the same clinical field for further training15. In that same study, they sought to identify traits that were ranked as most important when selecting a role model. The traits that they found to be most important were personality, clinical skills and competence and teaching ability, whilst interestingly the least important attributes were research achievement and academic position15. So, what can we take away from these studies and apply to real life? This shows that to inspire others to consider your particular field of medicine you don’t necessarily need to be leading a ground-breaking trial in gene therapy for haemophilia but you do need to be kind, patient, a good teacher and most importantly of all, a good doctor to your patients.
To conclude, when people are inspired, they are willing to take a daring leap towards something not previously considered an option or even a possibility. Inspiration is more than appreciation, it propagates a torrent of energy and an awareness of enlarged possibilities and it can happen when you least expect it, where you weren’t looking for it, and in the most menial of places. As doctors, we must recognise that part of our role includes inspiring a future generation of medical professionals and that this can be achieved through portraying the qualities we once found inspiring ourselves.
Bibliography
- General Medical Council. Approve Postgraduate Curricula. (2019). Available at: https://www.gmc-uk.org/education/standards-guidance-and-curricula/curricula. (Accessed: 8th January 2020)
- McNaughton, E., Riches, J., Harrison, G., Mires, G. & MacEwen, C. What factors influenced the choice of medical specialty for doctors surveyed in the final year at medical school and again having entered their specialty training destination? Postgraduate medical journal 94, 312–313 (2018).
- Ko, H., Lee, T. & Leung, Y. Factors influencing career choices made by medical students, residents, and practising physicians. BCMJ 49, 482–9 (2007).
- Yang, Y. et al. Factors influencing subspecialty choice among medical students: a systematic review and meta-analysis Factors influencing subspecialty choice among medical students: a systematic review and meta. BMJ Open 9, 22097 (2019).
- Vaglum, Wiers‐Jenssen & Ekeberg. Motivation for medical school: the relationship to gender and specialty preferences in a nationwide sample. Med. Educ. 33, 236–242 (1999).
- Mcmanus, I. C., Lefford, F., Furnham, A. F., Shahidi, S. & Pincus, T. Career preference and personality differences in medical school applicants. Psychol. Heal. Med. 1, 235–248 (1996).
- Rangarajan, S. et al. AAV5–Factor VIII Gene Transfer in Severe Hemophilia A. N. Engl. J. Med. 377, 2519–2530 (2017).
- Pasi, K. J. et al. Multiyear Follow-up of AAV5-hFVIII-SQ Gene Therapy for Hemophilia A. N. Engl. J. Med. 382, 29–40 (2020).
- Jones, P. The early history of haemophilia treatment: a personal perspective. Br. J. Haematol. 111, 719–725 (2000).
- Nilsson, I. M., Hedner, U. & Ahlberg, Å. Haemophlia Prophylaxis in Sweden. Acta Paediatr. 65, 129–135 (1976).
- Mannucci, P. M. Hemophilia: treatment options in the twenty-first century. J. Thromb. Haemost. 1, 1349–1355 (2003).
- Ellison, J. House of Commons Hansard Written Answers. www.parliament.uk (2013). Available at: https://publications.parliament.uk/pa/cm201314/cmhansrd/cm131024/text/131024w0002.htm#131024w0002.htm_spnew1. (Accessed: 31st January 2020)
- Hochhaus, A. et al. Long-Term Outcomes of Imatinib Treatment for Chronic Myeloid Leukemia. N. Engl. J. Med. 376, 917–927 (2017).
- Pavanello, F., Zucca, E. & Ghielmini, M. Rituximab: 13 open questions after 20 years of clinical use. Cancer Treatment Reviews 53, 38–46 (2017).
- Wright, S., Wong, A. & Newill, C. The Impact of Role Models on Medical Students. J. Gen. Intern. Med. 12, 53–56 (1997).
In 2018 the essay title was 'Patients are accessing their results and records. What are the implications for haematologists?' The winner was Soumya Ojha from the University of Birmingham and the runner-up Alison Gooda from the Oxford Medical School.
Introduction
Since the introduction of the General Data Protection Regulations in May 2018, it is now possible for National Health Service (NHS) patients to request access to their health records, and it is a legal requirement for healthcare professionals to provide this for free, with some exceptions.1 Providing patients with access to their records and results, particularly through online portals, is a growing worldwide phenomenon, with countries including Sweden, Denmark and Estonia already running such programmes.2 While several studies have been conducted which explore the implications of this in general practice and various medical specialties, there is limited evidence for the implications this would have in haematology. As a specialty which is highly reliant on results of investigations, implications may vary from other areas of medicine. This essay will explore the evidence behind providing patients with access to their records, the positive implications, possible limitations, and ways that the limitations can be addressed in the context of haematology.
Positive Implications
One of the main arguments in favour of providing patients with access to their records is to promote patient autonomy and greater health literacy. In order for patients to make their own decisions about their care, they need to be well-informed about their health. This is a central theme emphasised by the Department of Health, in their document “Liberating the NHS: No Decision About Me, Without Me”.3 The vision portrayed in this document is to ensure patients are as involved in making decisions about their care as they wish to be. One way to bring about the principle of shared-decision making is through patient-accessible health records. Providing patients with a way to understand health information invites them to participate in their disease management in a meaningful way. It allows for the fostering of an equal partnership between the doctor and the patient.
A systematic review by de Lusignan et al showed several benefits of providing patients with access to their records. Four studies included in this review showed improvements in medication compliance. 16 studies showed high levels of patient satisfaction. Overall, patients felt more empowered to communicate effectively with their doctors, and reported an improvement in the patient experience.4 A qualitative study by Rexhepi et al explored the attitudes of cancer patients towards online access of their medical records. The study found that this did not cause severe anxiety, and did not increase the number of phone calls to doctors.5 Patients reported that the intervention encouraged them to be involved in their own care, and improved their relationship with their doctor.5 A United States-based study explored the impacts of OpenNotes, a programme which provides patients access to their records. The study involved 19,000 patients across three sites and found that after the 12-month study period 99% of patients would continue to access their results online. None of the doctors involved in the study wanted to stop providing patients with access to their record. Over two-thirds of patients felt they understood their medical condition better and felt more in control of their care.6 This is especially important in chronic disease management, which is very common in haematology.
The management of many haematological diseases requires regular blood tests to monitor cell counts. Leukaemia patients regularly need full blood counts to track the white cell count, and myeloma patients frequently have their paraprotein levels checked as a marker of disease status. After having their blood test, patients may ring a nurse to find out their results the same day or wait for their next appointment, which may be a substantial amount of time later, to find out their results from their haematologist. This waiting time may cause a lot of anxiety for patients. By introducing a method where haematology patients can monitor their own results, the burden on healthcare staff and patient anxiety while awaiting results can be reduced.
The same can be applied to Chronic Lymphocytic Leukaemia (CLL), which is often managed with a “Watch and Wait” approach.7 This can be difficult for patients to understand, as they have been labelled with a diagnosis of a form of “cancer” but are not receiving any treatment for it. Leukaemia Care conducted a study which showed that 53% of patients who were put on a “Watch and Wait” approach reported feelings of worry or concern about being managed in that way.7 By providing patients with a way to monitor their own results and a record of their consultations, patients can feel more involved in the management of their long-term condition. Professor Schuh is a consultant haematologist at the Oxford Cancer Centre, who has been using Patients Know Best, a commercial application that provides patients with access to their records, for patients with CLL.8 74 of her patients currently use the programme, allowing them to view their test results, have an updated medication list and document their symptoms.8 Using this application provides support for patients to manage their condition from home. This has benefits for both the patient and haematologists. For patients, it reduces the inconvenience of having to come to hospital repeatedly to find out their results. For haematologists, there is greater efficiency, as less time is spent in consultations, providing possible economic benefits. A benefit for both patients and haematologists is that these patient portals allow for a more robust clinical record that takes into account the views of both parties.
Some haematological diseases render patients transfusion-dependent, such as myelodysplastic syndrome and thalassaemia. These patients can benefit from records of their haemoglobin levels, and correspond their results to symptom trackers. This data can support the doctor and the patient in setting individualised haemoglobin target levels for the patient. This example highlights how patient-accessible health records can support personalised medicine.
Another potential use of providing patients access to records is in Therapeutic Drug Monitoring (TDM). Ciclosporine can be prescribed as immunosuppressive therapy for patients following stem cell transplant to prevent graft versus host disease (GvHD).9 Patients taking this drug must have their blood ciclosporine levels monitored frequently for toxicity and possibility of GvHD.9 Explaining this to patients, making them aware of their target drug concentration levels, and providing them access to their records, allows patients to actively engage in their care, and may increase adherence to medication.
Many chronic haematological conditions exist in children, and carry on into adulthood, such as thalassaemia, sickle cell anaemia and haemophilia. Transitioning from paediatric to adult services can be difficult, as patients will have to get to know a new team and provide them with detailed information of their medical history. The NICE Guidelines pathway “Transition from Children’s to Adult Services” highlights that allowing patients to be a part of this transition may help build their self-confidence in managing their condition.10 Giving patients access to their records can make this transition smoother, ensuring all parties have all the necessary information to make safe healthcare decisions that the patient is happy with. An important aspect of this transition is the increasing independence the patient may desire in their day-to-day life. Therefore, establishing contingency plans and allowing the patient to be a major director of their own care can help them live the type of life they want.
A study in the Canadian Oncology Nursing Journal surveyed 46 patients with haematological malignancies about electronic records. 89% expressed a desire to access their results. 79% stated they would like educational materials in addition to the results.11 In developing the NICE Guidelines, ‘Improving Outcomes in Haematological Malignancies’, evidence from focus groups by the National Cancer Alliance was used. In these focus groups, patients explained the need for information about effects from treatment, how long they will require treatment, and potential consequences of their disease and treatment. All patients (100%) said they would have liked a copy of their treatment plan.12 In addition, the National Patient Survey highlighted that patients with Non-Hodgkin Lymphoma in particular, had problems with inadequate information. Compared to patients with solid malignancies, they were less likely to understand why tests were conducted (30% vs 17%).12 18% stated that they would have preferred more information on the outcome of their treatment.12 These studies all show that there is a need for greater information for haematology patients, which can be met through providing them with access to their records and results.
From a health-economics perspective, studies that evaluated the potential economic impact of patient access to records have shown it to be cost-effective. The Department of Health published a report in 2012, which estimates that the intervention would cost £1.3 billion, and could save £6.3 billion over the next ten years.13 A 2010 report by the European Commission studied 11 case studies of electronic health records for patients and found that economic benefits are evident within four to nine years of implementation and are sustainable.13 Costs to consider include implementation of the necessary technology systems, integration of new systems with existing technology infrastructure and training staff members on how to use the applications. It is suggested that costs can be saved by achieving earlier diagnosis and a reduction in errors. Taking a macroeconomic point of view, since providing access to records is associated with better self-care, this may allow a greater number of people to continue in the workforce, thereby contributing to positive socioeconomic benefits on a larger scale.13
Limitations
One of the biggest concerns that physicians have about providing patients access to their records and results is the possibility of patients misinterpreting their results. This concern is very relevant in haematology - a specialty which is highly reliant on the interpretation of test results. However, tests are not the only indicator of disease. Haematologists use these tests in conjunction with the patient’s clinical features to diagnose and decide on suitable management options for the patient. For example, a patient who sees an elevated white cell count might worry they have some form of leukaemia, when in reality, it could be reactive to a viral illness. This would be distinguished through a careful history, interpreting the morphology of cells under a microscope, and the clinical examination of the patient.
With access to results, patients may seek online or other resources to interpret results themselves. This is highlighted in Giardina et al’s mixed-methods study, which explored patients’ perceptions of viewing their results through online portals. The study took place across four large healthcare settings in the United States. Of the 95 participants interviewed, half of them tried to find out more information about their results from a source that was not their doctor. 46% found additional information from the internet and 51.6% from discussing it with friends and family. The study quoted one participant saying, “I’ll sit down and google it and see if I can figure it out that way before I bother (doctor’s name) ... Those doctors are swamped”.14 Patients searching the internet to try and interpret their results may approach resources which are not evidence-based and find misleading information. This has negative consequences on patient health literacy, which can have severe consequences on patient outcomes.
Giardina’s study also found that 63% of patients did not receive any information or interpretation at the time of receiving their test results. 55.8% of patients who received abnormal results experienced negative emotions, including frustration, anxiety and confusion. Participants with abnormal results were more likely to call their doctor than those who received normal results,14 thereby highlighting that when not implemented properly, this intervention might actually increase the workload of doctors. The study strengthens the argument that simply providing the result and a “normal” reference range to patients is not always sufficient. What is more useful is for the patient to know if this abnormal value is of any clinical significance or if it puts the patient at risk of anything that requires immediate management. There needs to be adequate information support available for this intervention to be meaningful. Signposting patients to reliable sources of information on the internet should be provided with test results.
A major concern expressed by both patients and doctors is the maintenance of data confidentiality.15 Since the growing trend in providing patients with access to their results is mainly through electronic systems online, there is a risk that patients’ sensitive medical information may be shared with unauthorised parties such as insurance companies and employers.15 The cost of implementing systems which are rigorous enough to protect this data securely is a potential barrier, but initial investment can bring about several benefits in the long term, as discussed above.
Another issue is the variance in computer literacy and access to the internet among patients. This can widen the already existing inequalities in healthcare.2 In studies that have been conducted, it is evident that older patients are less likely to use this service.16 This is relevant in the context of haematology, as the incidence of many haematological disorders increase and peak in older ages.17 Though these limitations are widely established in the literature, there is yet to be a solution to address this issue.
Finally, in previously conducted studies, patient uptake of this service has been low. For example, in a pilot study called the Patient Portal, which provided cancer patients with access to data held about them by the National Cancer Registration Service, uptake of the intervention was only 24% (95% CI 19-29%).18 Similarly, in a trial providing results for rheumatology patients, only 54% of those who had home internet access used the patient portal to view their health record.16 Underlying issues of low uptake include lack of internet access, lack of awareness about the availability of accessing patient records, and limited use amongst healthcare professionals and their encouragement of patients to access their records online. This can be addressed by provision of training services for patients and staff.
Discussion and Conclusion
From the available research, it is clear that many patients want access to their records, and there are several positive implications. In general, it can improve patient engagement and self-care, in some cases it can reduce patient anxiety, and can be convenient for both doctors and patients. Specifically, in haematology, there is evidence from the literature of a clear need, and several examples where it can be effective, including chronic disease management, aiding the transition from paediatric to adult services, in diseases which require a “Wait and Watch” approach and in therapeutic drug monitoring. However, there is insufficient evidence in haematology to make conclusions confidently. There is a need for studies evaluating this intervention, specifically in the speciality, to fully understand the implications for haematologists and their patients. Research needs to explore how much information should be available for patients (full access or partial access), and when this data should be made available to patients (immediately or after review by a healthcare professional). If this is to be implemented, the limitations discussed in this essay should be taken into account, including: the need for adequate information for support, data security, addressing inequalities of provision and training for healthcare professionals and patients.
References
- Medical records access [Internet]. British Medical Association. 2018 [cited 29 December 2018]. Available from: https://www.bma.org.uk/advice/employment/fees/medical-records
- European Union of Medical Specialists. Patient access to the electronic health record [Internet]. European Union of Medical Specialists; 2013.
- Department of Health. Liberating the NHS: No Decision About Me, Without Me. Department of Health; 2012.
- de Lusignan S, Mold F, Sheikh A, Majeed A, Wyatt J, Quinn T et al. Patients' online access to their electronic health records and linked online services: A systematic interpretative review. BMJ Open. 2014;4(9): e006021-e006021.
- Rexhepi H, Åhlfeldt R, Cajander Å, Huvila I. Cancer patients’ attitudes and experiences of online access to their electronic medical records: A qualitative study. Health Informatics Journal. 2016;24(2):115-124.
- Walker J, Meltsner M, Delbanco T. US experience with doctors and patients sharing clinical notes. BMJ. 2015;350.
- Leukaemia Care. Watch and Worry/Wait [Internet]. Leukaemia Care. Available from: https://www.leukaemiacare.org.uk/wp-content/uploads/2018/04/FINAL-Watch-and-Wait-report-Leukaemia-Care-EMBARGOED-16-APRIL.pdf
- Churchill Hospital Oxford University Hospitals [Internet]. Patients Know Best. 2017 [cited 29 December 2018]. Available from: https://www.patientsknowbest.com/oxford.html
- Ram R, Storb R. Pharmacologic prophylaxis regimens for acute graft-versus-host disease: Past, present and future. Leukaemia & Lymphoma. 2013;54(8):1591-1601.
- National Institute for Health and Care Excellence. Transition from children’s to adults’ services. NICE; 2018.
- Wiljer D, Bogomilsky S, Catton P, Murray C, Stewart J, Minden M. Getting results for haematology patients through access to the electronic health record. Canadian Oncology Nursing Journal. 2006;16(3):154-158.
- National Institute for Health and Care Excellence. Improving Outcomes in Haematological Cancers. London: NICE; 2003.
- Parliamentary Office of Science and Technology. Electronic Health Records. London: Parliamentary Office of Science and Technology; 2016.
- Giardina T, Baldwin J, Nystrom D, Sittig D, Singh H. Patient perceptions of receiving test results via online portals: A mixed-methods study. Journal of the American Medical Informatics Association. 2017;25(4):440-446.
- Miller Jr D, Latulipe C, Melius K, Quandt S, Arcury T. Primary care providers’ views of patient portals: Interview study of perceived benefits and consequences. Journal of Medical Internet Research. 2016;18(1): e8.
- van der Vaart R, Drossaert C, Taal E, Drossaers-Bakker K, Vonkeman H, van de Laar M. Impact of patient-accessible electronic medical records in rheumatology: Use, satisfaction and effects on empowerment among patients. BMC Musculoskeletal Disorders. 2014;15(1).
- Bron D, Ades L, Fulop T, Goede V, Stauder R. Aging and blood disorders: New perspectives, new challenges. Haematologica. 2015;100(4):415-417.
- Patient Portal. Patient Portal: Pilot Phase Evaluation [Internet]. Patient Portal; 2015. Available from: https://www.cancerresearchuk.org/sites/default/files/patient_portal-evaluation_final.pdf
Introduction
The past few decades have brought a shift in the physician’s role, from dictatorial to informative, with a patient’s autonomy and wishes being, in theory, paramount. Access to the medical record is a key aspect and symbol of this. Patients have had the theoretical right to access their own medical records since 1988, but the growth of IT services is increasingly facilitating routine access and dividing health professionals in opinion. Their fears centre on patients’ lack of trust in health professionals, confusion around medical jargon and anxiety following reading their medical notes. Despite these doubts, patient portals (as electronic health records with patient access are commonly termed) have the potential to decrease errors, allow closer disease monitoring and facilitate communication.
Haematology is a prime example for the use of such measures as many conditions are chronic and complex and diagnostic methods are inaccessible for the majority of patients. It is important that patient access is used as a tool for promoting doctor-patient communication, understanding and adherence to treatment. In cases where the relationship has broken down, a patient needs more support, rather than being left with the raw data.
The haematologist is not just a scientist, conveying medical results, but an advisor, bound by ethical codes. Here I will consider four key principals: autonomy, beneficence, non-maleficence and justice in order to assess the potential impact on haematologists and their patients.1
Changing attitudes and the role of technology
Following the Access to Health Reports Act of 1988 and the Data Protection Act of 1998, any competent adult may apply for access to their medical records and parents may access their children’s, unless against a competent child’s wishes,2 Despite this, few patients actually accessed their records. Physicians’ attitudes were initially very mixed, with scepticism including frightening and confusing patients and the poor quality of hospital notes. S. Ross et al evaluated the effects on the patient of accessing their records, finding that levels of confusion were reported at between 20% and 60%3. These misgivings are well summarised in a British Medical Journal article of the time,4 but it also states that “proper explanation would have to become the rule in consultations rather than the exception”, something which I think is key to successful implementation of personal health records.
A tenet of the ‘5 Year Forward View’ of 2014 was that by 2019 all patients will have access to electronic medical and care records which they will be able to write into. Although this is unlikely to be realised in full within the next few months, there have been several attempts to achieve this aim. Many GP practices now offer some degree of online access, but countrywide attempts have failed. Now companies offering IT services such as ‘Patient Knows Best’ are offering smaller scale solutions, rather than rolling out across the nation. These patient health records (PHRs) were reviewed by the Royal Society of Physicians,5 using a survey, literature review and case studies to investigate their function, usage and outcomes. They found that they were used sporadically, often for specific patient populations, including type-2 diabetes mellitus and sickle cell disease. The number of respondents to the survey was low, but found the most common reasons to access services were to find out more information about their condition, to order repeat prescriptions, to obtain care information and to record information. Of course, this is a highly selected patient subset and thus their usage may not be an accurate reflection of trend in a larger, more diverse population.
Facilitation of patient empowerment and autonomy
Patient access to medical records is part of a movement towards greater patient autonomy and informed choice. It can be difficult to see the negative implications, but I believe that giving patients information without explanation or counselling is irresponsible. This has been seen in recent years with the rise of private genetic testing companies and home PSA tests, where the NHS can be left to pick up the costs for follow-up consultations and testing that is often unnecessary. This shift has been accompanied by a transition in the weighting of ethical values seen in key medico-legal cases of capacity.6 These considerations apply especially to haematology, where some haematological treatments require long-term cytotoxic drug regimens, which may not be curative. Thus, it is vital that the patient's own concerns are at the centre of treatment. Cooperative access to medical records can be a key way of achieving this.
The implication for haematologists is that they will have a new role in educating patients in taking responsibility for the management of their own healthcare resources, and assessing the extent to which individual patients can manage this. This will require early input from clinicians, but with potentially long-term gains in efficiency and patient outcomes.
In oncology, the use of oral chemotherapy is rising.7 This brings lower costs, no venous access requirement and reduced hospital stay. However, they do still have considerable side-effect profiles and risks of drug interactions. Adherence is also poorer and associated with worse outcomes. This is an area where engaging patients with their own treatment by giving them access to test results and the ability to check their treatment regimens is likely to benefit patients through improved outcomes. Additionally, haematologists will receive updates when patients take their medications and when they experience side effects. This could allow more rapid adaptation of regimens and response to patients’ concerns.
Medical outcomes: Beneficence
One of the first areas in which patient responsibility for and access to medical records was adopted was in obstetrics. The maternity record gives women constant access to their pregnancy health records and women involved in the pilot studies reported feeling more able to discuss issues with healthcare professionals.8
As well as having psychological benefits for patients, opening up the records to a critical pair of eyes will mean notes will have to be legible and logically written and also that inaccuracies are more likely to be spotted. S. Bogardus et al9 found a lack of consistency between impaired function documented in the notes and that found at interview. Functional status is a really useful predictor of outcomes such as length of stay and if physicians are failing to recognise this, it could lead to lost opportunities for intervention. A study of residents from a Michigan teaching hospital added to this finding.10 Less than half of cases of medical errors recalled by residents were found documented in the notes, including serious errors, such as pneumonia being missed on chest X-ray, or a young man with an aortic dissection being sent home.
If errors in medical records can be reduced and if patients understand and trust what is in them, they will be more likely to consent to the sharing of their data in the construction of databases for research purposes, such as that attempted by Care.data. The analysis of large datasets will hopefully have a substantial positive impact on haematology. Previously the evidence base for autologous haematopoietic stem cell transplantation in elderly patients was poor, but a Medicare database of patients with multiple myeloma was used by Winn et al to show that median survival of those transplanted patients was significantly higher.11
The Oxford Cancer Centre at the Churchill Hospital has recently begun to use Patient Knows Best (PKB) to allow chronic lymphocytic leukaemia (CLL) patients to view blood test results, share them with different specialists and report on symptoms and side effects.12 Previously, patients would have to frequently attend clinics for monitoring of any complications. Patients may be in the asymptomatic early stages, requiring monitoring for the onset of symptoms. Later stage CLL is treated with a wide array of drugs such as chlorambucil, fludarabine and newer BCR targeting therapies, which all contribute further to the primary immunodeficiency. Systems such as PKB allow patients to update doctors on new symptoms of infections or side effects in real time. The chronicity of CLL lends itself well to an IT reporting system, as patients will often see their specialist for years and thus develop a relationship where face to face communication isn’t always required. Such an approach does require organisation on the patient’s part and so a responsibility will lie with the haematologist to ensure that a patient is able to manage this and the haematologists will have to familiarise themselves with the interface. PKB also allows the patient to share their data with different specialists and so facilitate interdepartmental cooperation. This is really important in patients with comorbidities.13
Preventing adverse events: Non-maleficence
Patient access and IT go hand in hand. Denmark is often seen as a world leader in terms of integrating IT usage into its medical service. A quarter of its population use its national portal to access their medical data.14 However, they have suffered from software fragmentation, with different trusts struggling to exchange information. There are several reports of complex cancer patients’ data being lost, leading to substantial treatment delays. The implications are that, although there has been recent success with small groups of patients accessing medical records, care must be taken that in the long-term integration of care is a priority.
Another source of potential error stems from the patients themselves. D. Solomon et al15 looked at data from the Brigham Rheumatoid Arthritis Sequential Study on patients’ past and current medications and compared these with self-reported data from questionnaires. Agreement for current medications was excellent, but for past medications it was much lower, ranging between 0.13 for methotrexate to 0.74 for aurothioglucose. If we allow patients to not just access but add to medical records, it could lead to contradicting reports. However, outcomes such as this have not been reported to date. In fact, evidence suggests that patient access is more likely to prevent drug errors. A paediatric oncology ‘shuttle sheet’ for families of children with diseases such as acute lymphoblastic leukaemia or Wilms’ tumour was developed to help the management of long, complex cytotoxic drug regimens with organ toxicity.16 Children were often treated at several different centres which resulted in a lack of coherent care. The sheet reduced the large amounts of correspondence between staff and the families and also helped to prevent treatments being missed or duplicated when treatment was shared between centres.
Electronic medical records EMR can be utilised to improve communication between departments. This is excellently demonstrated by St Bart's haematology department who found that when patients with severe haematological conditions presented to ED, their underlying conditions would often not be recognised.17 Following introduction of EMRs there were no clinical incidents in the department (2010-2012). Although in this case they did not give patients access to these records, I feel this would be the next logical step in preventing missed health conditions.
An example of patient access to health records being poorly managed was in the US military, where it was common practice for patients to keep their medical records.18 However, there was little input into optimising this and so records often went missing or were with the patient when results came back. This demonstrates how simply following the principal that patients should have unencumbered access to their records doesn’t automatically lead to improved efficiencies and empowerment.
Healthcare equality and justice
A key consideration is making patient uptake equal across different socio-economic, educational and racial groups. This is an issue encountered with many opt-in medical services; the much-cited example is screening programmes, which tend to be used by wealthier, more educated women. A Personal Genomics (PGen) study by J.S. Roberts et al19 used web surveys to profile users of home genetic testing kits. Compared to the US population, participants were more likely to be female, middle aged and with high levels of education and income. Although these findings cannot be directly carried over, it does imply that alongside implicating mechanisms for patient medical records, uptake and usage should be monitored. Another concern is that those with a greater educational level are both more likely to access and more likely to understand their notes. Such patients are likely to already be engaged in their own healthcare. A literature review of factors affecting patient portal usage by T. Irazarry20 found that conceptual health knowledge, numeracy and computer skills were key factors that contributed to successful patient uptake and use. This again emphasises that rolling out initiatives is only the beginning of giving patients meaningful input and access to their own health care. The responsibility will lie with the haematologist to ensure that their individual patient is able to interact with the system.
Conclusions: Patient portals as a tool
Technological advances and pressure for the patient to be put at the centre of medicine are creating a movement for patients to ‘own their own data’. Recent successful case studies such as the use of PKB in the Churchill hospital haematology department and the integration of electronic medical records by St Bart’s add to the body of earlier evidence that patients should be put at the heart of developing EMR systems. When designing the ideal system, accepted key features include: a secure messaging system, options for reporting symptoms, high levels of security and the ability to review appointments. PKB has gone one step further in making the patient key in deciding which physicians are able to view their records. However, in my opinion an ideal patient portal system should be patient centred but doctor led. Privacy and ownership by the patient should be given a high priority, but the professional role of the haematologist is to evaluate care options and present them to a patient with appropriate advice and support. I believe this is at risk of being lost. Ideally patients should be able to routinely access their medical records only if they are regularly consulting with a doctor to support them. Such a system should not shift the majority of responsibility to the patient, but instead allow them a greater input into healthcare decisions.
Bibliography
- Beauchamp, T. L. & Childress, J. F. Principles of biomedical ethics. (Oxford University Press, 2001).
- Dimond, B. Rights to information access under the Data Protection Act. J. Nurs. 14, 774–776 (2005).
- Ross, S. E. & Lin, C.-T. The effects of promoting patient access to medical records: A review. Am. Med. Informatics Assoc. 10, 129–138 (2003).
- Metcalfe, D. Whose data are they anyway? Med. J. 292, 577–578 (1986).
- Wyatt, J., Sathanandam, S., Rastall, P., Hoogewerf, J. & Wooldridge, D. Personal health record (PHR) landscape review: Final report. (2016).
- Larner, E. & Carter, R. The issue of consent in medical practice. J. Haematol. 172, 300–304 (2016).
- Halfdanarson, T. R. & Jatoi, A. Oral cancer chemotherapy: The critical Iiterplay between patient education and patient Ssfety. Oncol. Rep. 12, 247–252 (2010).
- Young, D. Who should hold the medical record -Provider, parents, or both? Birth 18, 2–4 (1991).
- Bogardus, S. T., Towle, V., Williams, C. S., Desai, M. M. & Inouye, S. K. What does the medical record reveal about functional status? Gen. Intern. Med. 16, 728–736 (2001).
- Rosenthal, M. M., Cornett, P. L., Sutcliffe, K. M. & Lewton, E. Beyond the medical record other modes of error acknowledgment. doi:10.1111/j.1525-1497.2005.0098.x
- Winn, A. N., Shah, G. L., Cohen, J. T., Lin, P.-J. & Parsons, S. K. The real world effectiveness of hematopoietic transplant among elderly individuals with multiple myeloma. JNCI J. Natl. Cancer Inst. 107, (2015).
- The Oxford Cancer Centre works with Patients Know Best to improve care for leukaemia patients. Patient Knows Best (2017). Available at: https://www.patientsknowbest.com/oxford.html. (Accessed: 31st December 2018)
- Tuso, P. et al. Complex case conferences associated with reduced hospital admissions for high-risk patients with multiple comorbidities. J. 18, 38–42 (2014).
- Kierkegaard, P. EHealth in Denmark: A case study. Med. Syst. (2013). doi:10.1007/s10916-013-9991-y
- Solomon, D. H. et al. Agreement between patient report and medical record review for medications used for rheumatoid arthritis: The accuracy of self-reported medication information in patient registries. Arthritis Rheum. 57, 234–239 (2007).
- Stevens, M. M. “Shuttle sheet”: A patient‐held medical record for pediatric oncology families. Pediatr. Oncol. 20, 330–335 (1992).
- DHI News Team. Bloody good show. digital health Available at: https://www.digitalhealth.net/2012/07/bloody-good-show/. (Accessed: 29th December 2018)
- Curtis, M. R. The patient-carried medical record. Healthc. Qual. 11, 34 (1989).
- Roberts, J. S. et al. Direct-to-consumer genetic testing: User motivations, decision making, and perceived utility of Rrsults. Public Health Genomics 20, 36–45 (2017).
- Irizarry, T., DeVito Dabbs, A. & Curran, C. R. Patient portals and patient engagement: A state of the science review. Med. Internet Res. 17, e148 (2015).
In 2017 the essay title was 'Discuss the possible roles of artificial intelligence in the future practice of haematology'. The winner was Marco Narajos from the University of Oxford and the runner-up Karan Dahele from the UCL Medical School.
Introduction
It is said that there are as many definitions of Artificial Intelligence (AI) as there are AI researchers in the field. Many understand the term AI to mean a computing system that behaves as a human mind would. However, many AI systems do not, in fact, aim to mimic the vast complexity of the human mind, let alone that of a haematologist!
In simplest terms, all that computers do is take an Input through a Process to obtain an Output. Machine learning algorithms, for example, can enable computers to learn for themselves, such as by presenting the computer with Inputs and Outputs and prompting it to figure out the Process all by itself, without a human programming it in the first place. We can achieve this so-called ‘deep learning’ in different ways, such as with an artificial neural network (ANN) – a computer system inspired by the rules that govern how neurons in the brain send impulses.
Deep learning with ANNs is only one type of machine learning and machine learning is only one facet of AI. However, we humans, and especially haematologists, pride ourselves on our ability to learn and solve problems. Thus, over the last few years, machine learning has become synonymous with AI. This has not always been the case, as many authors have previously used the term AI to refer to decision support systems that make clinical judgements from complex information like a human clinician would.1
No matter what definition or aspect of AI is in vogue, it is clear that AI will play significant roles in the future practice of medicine. This paper seeks to identify and predict how AI, focusing on machine learning, will interact with diagnostics, prognostics, therapeutics, research, and even management in haematology.
Diagnostics
Haematology’s strength as a specialty lies in its diagnostic capability. Not only is haematology its own valid specialty, it also acts as a clinical support service for primary care and inpatient settings. For this reason, a fast turnover of laboratory results and accurate diagnoses are important, and AI is on its way to doing just that.
In 2009, an Iranian group developed an ANN that they trained to extract features from a dataset of 360 patients and validated it on a sample of 90 others.2 Because of the way ANNs mimic how neurons take on multiple inputs from other neurons, this form of AI has the ability to handle multiple input parameters of different weights or levels of importance. In this case, 11 values from a full blood count (FBC) alone could simultaneously churn out the absence or presence of five diseases – megaloblastic anaemia, thalassaemia, idiopathic thrombocytopaenic purpura, chronic myelogenous leukaemia, and lymphoproliferative disorder – with supposedly near 100% accuracy. There are many flaws in the paper but it is proof of concept that an AI can make a FBC alone be of diagnostic significance. This is especially important in developing countries where there may not be access to other investigations or indeed consultant haematologists.
The holy grail would be the ability to train a computer to report on and even make diagnoses from blood films and bone marrow samples. A Nature Communications paper sparked a media frenzy last year over AIs supposedly outperforming human physicians.3 In fact, the published work was for programming an AI to learn to differentiate between lung adenocarcinoma and squamous cell carcinoma from H&E-stained slide images alone and to extract features from the images that would predict mortality. The media frenzy, no doubt, made sure that AI was the cynosure of all investors’ eyes, but in truth, the AI was trained and validated on images that had unequivocal adenocarcinoma and squamous cell carcinoma features. Pathologists, and haematologists likewise, will see cases on the borderline with artefactual distortions, which means that AI trained on model images would unlikely outperform a clinician. Also, even though we consider the human eye as the gold standard diagnostic test, intraobserver concordance between consultants may not even approach 100%. For this reason, AI still has a long way to go before reporting on blood films and bone marrow samples on behalf of human doctors.
Prognostics
Despite that diagnosis is often an easier task than prognostication, more research exists for using AI in prognostics in haematology, perhaps reflecting haematologists’ eagerness to peer closer into the crystal ball. One 2010 paper examined how well an ANN machine learning algorithm could predict acute graft-versus-host disease (aGVHD) after unrelated donor haematopoietic stem cell transplantation (UD-HSCT) for β-thalassemia major.4 The Italian team compared the ANN with logistic regression. This is a type of classical statistical analysis that can examine how variables determine an outcome, such as the absence or presence of aGVHD. They used 24 variables to train the ANN to analyse the most important factors that associate with aGVHD. Although the ANN did not calculate which factors were most important, it performed significantly better at predicting who would not develop aGVHD, with a specificity of 83% vs 22% for logistic regression. There was no significant difference between the ANN’s sensitivity at picking up future aGVHD, which was high at 90% compared to 81% for logistic regression. This is proof of concept that machine learning can be more effective than traditional statistical methods at predicting complications of haematological procedures.
Therapeutics
Many of our haematological therapies, especially when used in combination, have narrow therapeutic windows: too small a dose and we risk not treating the disease, too large a dose and we risk toxic side effects. Much like any field of clinical medicine, haematologists titrate drug doses based on clinical wisdom and target values.
Decision support systems (DSSs) make titration judgements easier and simpler, and have been around for a relatively long time. An example would be HiruMed’s anticoagulant dosing system (RAID), which does not use machine learning AI. However, the problem with DSSs is that their software has to be reprogrammed with new parameters every time there is new data that could change clinical decisions. Machine learning algorithms solve this issue because each new clinical data point added to the system allows the AI to learn for itself what factors contribute towards better or worse maintenance of a patient’s target value – in this case, the INR. It would be a tool not only for therapeutics but also for research, as so-called ‘supervised learning’ can allow the AI to identify patients who are at high or low risk of adverse events, and therefore, affect clinical management by ensuring the patient is seen or assessed more or less frequently.
A Swedish group tasked themselves with developing a DSS that would do just this, using Bayesian networks (BNs).5 A BN is a type of machine learning that uses Bayesian statistics, which is a more realistic or ‘more human’ way of inferring information from probabilities than traditional ‘frequentist’ statistics commonly taught in schools. Bayesian statistics looks at a priori information, such as a patient’s history of (recent) bleeding, strokes, alcohol consumption, and other variables, to determine a probability. Intuitively, this is how all clinicians think. The beauty in this algorithm is that it can provide a recommendation (such as a 10% dose increase in warfarin) and also a second probability that this recommendation would work to improve a patient’s INR value. The more information we provide about the patient, the more we can increase the algorithm’s confidence in its recommendation because BNs rely on a priori knowledge to adjust their outputs. The team trained the DSS with data from over 3,000 patients on nearly 50,000 hospital appointments. This impressive feat of Scandinavian recordkeeping has allowed this DSS to learn ‘rules of dose titration’, which agreed with hospital clinicians over 75% of the time. Whether the dose titrations that the DSS recommended performed just as well as clinicians is another matter. Interestingly, the DSS’s recommendations on the next follow-up date only agreed with clinicians around half of the time, with the DSS recommending shorter follow-up times.
It is important to emphasise that machine learning is not at all needed to personalise dosing to improve treatment response and safety from side effects. One group in Los Angeles developed a complex statistical model that they call phenotypic personalised medicine (PPM).6 PPM does not involve machine learning, and yet, science news media, including Nature Medicine, have bestowed it with the title of AI.7 PPM is a way of using a patient’s readouts of treatment efficacy and safety to determine the appropriate medication dose using the patient’s own record of how well they have done on a given dose of the drug. It consists of an elegant series of quadratic equations that use mathematical constants that clinicians can calculate individually for each patient. Instead of learning from thousands of patients to give a recommendation of what dose to provide (as in machine learning), PPM uses the patient’s own data (termed ‘n-of-1 medicine’). This makes personalised dosing possible without using machine learning. However, whether PPM is ‘true’ AI is debatable.
The Californian group developed this model for the maintenance therapy of paediatric patients with acute lymphoblastic leukaemia, and in their paper, showed a comparison between a patient whose drugs were dosed clinically compared to one whose drugs were dosed by PPM.8 According to clinical protocol, the clinician could only modulate the dose of 6-mercaptopurine and methotrexate, whereas the PPM was also able to modulate the doses of vincristine and dexamethasone. Clinical modulation led to significant clinical deviations away from the absolute neutrophil count (ANC) target, whereas the PPM kept the patient within the ANC and platelet count targets. Of course, the study is plagued with issues, as it was not a randomised controlled trial, clinician blinding was impossible, and the outcome measures were not patient-centred (e.g. mortality, quality of life, symptomatology). However, it is evidence that something AI-like can aid haematologists in their approach to therapy.
Research
Machine learning AI has the capacity to find patterns in data. In supervised learning, the AI has specific patterns it is taught to look for, whereas unsupervised learning allows the AI to search for features in the data that can be grouped together, even if the developers had not asked the AI to investigate specific variables. Both approaches are incredibly powerful tools in hypothesis-testing and hypothesis-generating research, respectively.
A group from Harvard used a supervised learning approach to direct a piece of machine learning AI to find a gene expression profile in diffuse large B cell lymphoma (DLBCL) at the time of diagnosis that would be predictive of a prognosis, such as cured disease versus fatal or refractory disease.9 From the 58 patients that the researchers separated into cured and fatal/refractory, they found 13 genes that were predictive of the patients’ prognosis.
In contrast, we can take an unsupervised learning approach to classify patients with DLBCL into two groups based on similar gene expression profiles, without directing the AI to specific genes.10 This led to the discovery of two putative types of DLBCL, associated with gene expression profiles and prognosis. This discovery was replicated by another group who used a supervised learning method with an ANN, which improved both diagnosing and prognosing DLBCLs based on the gene expression profile only.11
AI clearly has a major role to play in the future of haematology research, such as in finding markers of subgroups of disease that would aid our diagnostic classifications and prognostic understanding of disease.12 AI could potentially also support research logistics, such as identifying the patients who would be suitable for and amenable to entering a clinical trial.
Management
Management of a clinical haematology department and its various services is an important part of a consultant haematologist’s role. AI’s ability to learn from patterns in data, without the need for programming of rules, could help to streamline management by learning from datapoints such as patients’ addresses, staffing levels, (non-)attendances, numbers of inpatient and GP requests for haematology blood tests and transfusions, and costs of procedures and equipment. The AI could perhaps assist with budgeting, allocation of clinic time, audits, clinical governance, and supply and procurement of new equipment.
No research yet exists on how AI will affect the managerial role of haematologists, but it is a unique opportunity given the hybrid specialty that haematology is – part-laboratory and part-clinical. It is also feasible, given that software is already available to manage a haematology department, such as the DAWN management software package, which even includes its own, tried-and-tested dosing support system.13
Aside from management, there are also various opportunities for AI in haematology such as the development of chatbots that can answer queries from patients (or indeed medical students) about their care, which could improve compliance with medication, clinic attendance, and even medical students’ education.14
Conclusion
Many haematologists will muse about whether they are at risk of losing their jobs; most will assure themselves, rightly, that they are not at risk. All jobs are simply made up of a series of tasks and decisions. At the present moment, AI has such a limited repertoire of tasks that it can perform and decisions that it can make in haematology. For this logistical reason, there is certainly no imminent worry that haematologists will be replaced by AI.15 However, there are other sociocultural reasons that doctors are likely to keep their jobs. As clinicians, we take on a social and legal responsibility by being tasked to diagnose, prognose, and treat a patient. Furthermore, we as clinicians provide much emotional labour; AI will never be able to deliver or replicate empathy, companionship, and the human touch.16
Nevertheless, it is impossible to ignore the relentless rise of machine learning which has come in recent years, powered by the nascent prominence of the trifecta of Big Data,17 greater processing power,18 and state- and industry-led investment.19 In the not-too-distant future, it is likely we will see integration of AI in haematology clinics, laboratories, and even in mobile phone apps. We will probably see AI helping nurses to run clinics, in lieu of haematologists and GPs to reduce workload and address the shortage of doctors.20 AI could also take the form of an app prompting the haematologist with a list of differential diagnoses with the statistical probabilities of those conditions, cementing the role of the haematologist in providing his or her final diagnostic conclusion. Additionally, AI could assist haematologists with continuing to personalise therapies to the patient’s disease as well as ideas, concerns, and expectations of treatment. AI-powered research will also become more prominent in the coming years with the growing number of machine learning developers and their collaboration with haematologists could lead to important discoveries of causes and treatments of haematological disease. Moreover, AI could have a small but not insignificant role to play in managing growing, busy haematology departments to use data to improve organisation, decrease patient and doctor time wasted in clinics, and drive productivity.
All haematologists will need to be aware of AI – its types, mechanisms, benefits, and limitations for the practice of haematology – and how to utilise it to improve patient care, optimise services, and drive efficiency savings in an ever resource-limited society.
References
- Barbieri, B., Gamberoni, G., Lamma, E., Mello, P., Pavesi, P., & Storari, S. (2005). An expert system for the oral anticoagulation treatment. Innovations in Applied Artificial Intelligence, 7-18.
- Payandeh, M., Aeinfar, M., Aeinfar, V., & Hayati, M. (2009). A New Method for Diagnosis and Predicting Blood Disorder and Cancer Using Artificial Intelligence (Artificial Neural Networks). International Journal of Hematology-Oncology and Stem Cell Research, 3(4), 25-33.
- Yu, K. H., Zhang, C., Berry, G. J., Altman, R. B., Ré, C., Rubin, D. L., & Snyder, M. (2016). Predicting non-small cell lung cancer prognosis by fully automated microscopic pathology image features. Nature communications, 7.
- Caocci, G., Baccoli, R., Vacca, A., Mastronuzzi, A., Bertaina, A., Piras, E., ... & La Nasa, G. (2010). Comparison between an artificial neural network and logistic regression in predicting acute graft-vs-host disease after unrelated donor hematopoietic stem cell transplantation in thalassemia patients. Experimental hematology, 38(5), 426-433.
- Yet, B., Bastani, K., Raharjo, H., Lifvergren, S., Marsh, W., & Bergman, B. (2013). Decision support system for Warfarin therapy management using Bayesian networks. Decision Support Systems, 55(2), 488-498.
- Zarrinpar, A., Lee, D. K., Silva, A., Datta, N., Kee, T., Eriksen, C., ... & Wang, S. E. (2016). Individualizing liver transplant immunosuppression using a phenotypic personalized medicine platform. Science translational medicine, 8(333), 333ra49-333ra49.
- Chakradhar, S. (2017). Predictable response: Finding optimal drugs and doses using artificial intelligence. Nature Medicine 23, 1244–1247.
- Lee, D. K., Chang, V. Y., Kee, T., Ho, C. M., & Ho, D. (2017). Optimizing Combination Therapy for Acute Lymphoblastic Leukemia Using a Phenotypic Personalized Medicine Digital Health Platform: Retrospective Optimization Individualizes Patient Regimens to Maximize Efficacy and Safety. SLAS TECHNOLOGY: Translating Life Sciences Innovation, 22(3), 276-288.
- Shipp, M. A., Ross, K. N., Tamayo, P., Weng, A. P., Kutok, J. L., Aguiar, R. C., ... & Ray, T. S. (2002). Diffuse large B-cell lymphoma outcome prediction by gene-expression profiling and supervised machine learning. Nature medicine, 8(1), 68-74.
- Alizadeh, A. A., Eisen, M. B., Davis, R. E., Ma, C., Lossos, I. S., Rosenwald, A., ... & Powell, J. I. (2000). Distinct types of diffuse large B-cell lymphoma identified by gene expression profiling. Nature, 403(6769), 503-511.
- O'Neill, M. C., & Song, L. (2003). Neural network analysis of lymphoma microarray data: prognosis and diagnosis near-perfect. BMC bioinformatics, 4(1), 13.
- Zini, G., & d'Onofrio, G. (2003). Neural network in hematopoietic malignancies. Clinica chimica acta, 333(2), 195-201.
- Poller, L., Keown, M., Ibrahim, S., Lowe, G., Moia, M., Turpie, A. G., ... & Palareti, G. (2009). A multicentre randomised assessment of the DAWN AC computer-assisted oral anticoagulant dosage program. Thromb Haemost, 101(3), 487-494.
- Kerly, A., Hall, P., & Bull, S. (2007). Bringing chatbots into education: Towards natural language negotiation of open learner models. Knowledge-Based Systems, 20(2), 177-185.
- Manyika, J., Chui, M., Miremadi, M., Bughin, J., George, K., Willmott, P., & Dewhurst, M. (2017). A future that works: Automation, employment, and productivity. McKinsey Global Institute, New York, NY.
- Luneski, A., Konstantinidis, E., & Bamidis, P. (2010). Affective medicine: a review of affective computing efforts in medical informatics. Methods of information in medicine, 49(3), 207-218.
- Lohr, S. (2012). The age of big data. New York Times, 11(2012).
- Steinkraus, D., Buck, I., & Simard, P. Y. (2005, August). Using GPUs for machine learning algorithms. In Document Analysis and Recognition, 2005. Proceedings. Eighth International Conference on (pp. 1115-1120). IEEE.
- Chui, M. (2017). Artificial intelligence the next digital frontier?. McKinsey and Company Global Institute, 47.
- Fitzmaurice, D. A., Hobbs, F. R., Murray, E. T., Holder, R. L., Allan, T. F., & Rose, P. E. (2000). Oral anticoagulation management in primary care with the use of computerised decision support and near-patient testing: a randomised, controlled trial. Archives of Internal Medicine, 160(15), 2343-2348.
Artificial Intelligence: the future of haematology?
Artificial intelligence (AI) is broad, often misunderstood term. It refers to the science of simulating the brain’s cognitive ability within a computational system.1 In popular culture, the term is most often associated with robots in a dystopian future. In the media, AI often features in sensationalised headlines about job losses. This representation has fuelled fears of the impact its development will have on society.
The rapid development of AI has facilitated its use to solve a diverse range of problems in recent years, from playing high level strategy games, to understanding natural human speech. AI has huge application in medicine; there is exciting commercial and academic activity in cellular biology, genetics, drug discovery, diagnostics, treatment, and outcome prediction. There is no doubt that AI will play a huge role in healthcare in the future. This essay aims to introduce some of the key principles of AI, present some of the most exciting developments in haematology, and discuss how AI might shape the practice of haematology in the future.
Principles of AI
Machine learning refers to the process by which software takes data, “learns” from it, and produces functions to interpret new data. This is opposed to a traditional “hard-coded” predictive model, where the person designing the software would specify the function producing an output for each input. For example, in designing software to determine the probability of anaemic symptoms based on iron intake, a hard-coded solution might be programmed to increase risk by X%, below a certain value of iron intake. On the other hand, a machine learning solution would tackle the problem by analysing a large data set of relevant patients, and learning itself the nature of the relationship between the two factors. This approach quickly comes into its own when working with large, multi-factorial patient data, where manually finding complex patterns would be difficult.
There are two broad types of machine learning: supervised and unsupervised.
In supervised machine learning, the system is provided with a set of inputs, and a set of outputs. Algorithms aim to produce a function which will map the input to an output. Training refers to the process of feeding the system with data, so that it can learn trends and produce this function. This algorithm can then be applied to real cases, taking inputs and providing predictions. The system has been given data explicitly labelled with inputs and outputs.2
The crucial difference in unsupervised learning, is that the system is not given an explicit outcome variable. The data set used to train the system only has inputs. The model is tasked with finding trends and associations, without a specific outcome to link to; algorithms have free rein to elucidate any interesting patterns.2
In medicine, the most popular machine learning algorithms are currently support vector machines (SVM) and artificial neural networks (ANN).2
SVMs are used to classify a subject into one of two groups. Different traits (inputs) can impact into which group the subject is classified. Each trait is weighted, depending on how much it correlates to each of the two classification groups. Training an SVM involves optimising the values of the weights, so that the model’s predicted classification most correctly matches the actual classification given in the data set.
An ANN is modelled on the organisation of the brain, with artificial neurones connected in layers. Each artificial neurone receives a set of inputs, which are weighted (the “synapse” of the neurone), and produces an output. Each input’s weighting can be optimised through training (akin to an SVM). The layering of neurones allows the relationship between input and output to be broken down into smaller steps, allowing more complex patterns to be identified. These patterns might not be straightforward correlations between variables. A simple neural network might have 3 layers of neurones: an input, hidden, and output layer. Conversely, a deep neural network has many layers of neurones in between the input and output neurones (shown in Figure 1).
Figure 1: Simple and deep neural networks3
Applications
AI has potential uses in every stage of biomedical research and clinical practice.
Clinicians, including haematologists, are involved in both bench-side and clinical research. AI will play a crucial role in biomedical and pharmaceutical research. Artificial neural networks can analyse sparse or noisy data, such as genomic sequences, which would pose a problem for standard statistical methods. They are being used to aid prediction of protein folding based on genetic data,4 which forms the basis of diseases such as sickle cell. AI has also shown potential in designing new diagnostic pathways for clinicians to use. For example, a data mining approach was used to simplify the diagnostic process for Polycythemia Vera. The model produced an accurate diagnostic algorithm for clinicians to use, with four parameters (Hct, Platelet count, Spleen and WBC), in contrast to the existing pathway requiring eight parameters.5
Drug discovery is becoming increasingly expensive and difficult, with drugs frequently failing late clinical trials. Researchers are seeking new higher yield approaches to discover therapeutics. AI is being used to investigate potential drug targets in the treatment of Diamond Blackfan anaemia,6 a rare genetic disorder. Researchers are crossing many different drug compounds with cells of many genotypes (“high-content high-throughput” – around 1000 cell features per cell, with 10 million cells per week), and measuring the outcomes using immunofluorescent imaging. This is then analysed by machine learning algorithms, which suggest the most promising combinations for further investigation. AI is helping make sense of the “data deluge” produced by high-throughput approaches in biomedicine, but still requires highly knowledgeable clinician-scientists to oversee the training of models and to interpret results.
As well as research, AI can play an important role in diagnostics. Automated systems for carrying out full blood counts are common, and have made this investigation relatively rapid and inexpensive. A peripheral blood smear is another frequently used investigation, important for diagnosis of disorders of erythrocytes, leukocytes, and haemoglobin, as well as blood-borne infections. Currently, interpreting blood films to find diagnostic markers is done manually, requiring expert knowledge. There has been considerable progress in using deep neural networks to interpret blood films. Systems have been developed that can diagnose sickle cell disease with high accuracy, and even categorise sickle cells, with the potential to make predictions on severity of the disease.7 Researchers have also trained convolutional neural networks to identify markers of lymphoma in H&E stained histopathology slides of human lymph node tissue. They reported a 38% decrease in residual error over current comparable techniques.8 As well as interpreting images, AI has been successful in incorporating genomic sequence data into diagnosis. IBM Watson diagnosed a patient with a rare form of leukaemia, initially misdiagnosed by clinicians, by cross-referencing the patient’s genome with millions of known mutations learnt from oncology papers. Over the past year, Watson has been used for around 100 patients with haematological diseases at the same hospital.9 However, Watson has still not proved effective at diagnosing other types of cancer, largely due to a lack of accurately labelled, comprehensive patient data to train with.
As well as diagnosis, AI is shaping how haematologists treat patients. One example is the management of anaemia associated with chronic kidney disease (CKD). Damage to the kidneys can cause them to fail to produce enough erythropoietin, which leads to a hypoproliferative anaemia. Anaemia becomes more prevalent as CKD progresses, with almost all end stage CKD patients developing anaemia. Erythropoiesis stimulating agents (ESAs) are commonly used to treat the condition, despite associations with off effects, including cardiovascular events and malignancy. It is important to use the lowest effective dose, which still maintains haemoglobin concentration, whilst minimising dangerous side effects. However, it is difficult to calculate this optimal dose. In 2016, researchers used an artificial neural network to provide better individualised ESA dose recommendations.10 This approach resulted in higher rates of on-target haemoglobin values, less haemoglobin level fluctuation, lower average doses of ESA, and lower transfusion requirement. This study illustrates the role AI can play in personalising treatment.
Humans have inherent biases, which often impact clinical judgement. Doctors are considerably over-optimistic in estimating life expectancies, whilst AI models are much more accurate in estimating prognoses. For example, a Random Forest machine learning model has been used to predict likelihood of relapse of childhood acute lymphoblastic leukaemia with high accuracy.11 Other machine learning models have been used to predict mortality following allogenic hematopoietic stem transplantation, used to treat some patients with haematological malignancies.12 If used clinically, these models, and others like it, would be useful to haematologists to help stratify patients and personalise treatment plans.
Models like these could be considered to diminish clinicians’ autonomy. However, approaches taken to historical advancements in technology, such as imaging, suggest that these models may be integrated into a clinician’s decision-making process, rather than replacing it. It would form one more point of consideration among many other patient factors.
The future of haematology
A haematologist is responsible for the investigation, accurate diagnosis and therapeutic management of blood disorders. Haematologist have a range of roles: working in laboratories to interpret investigations, as well as caring for patients on wards and in outpatient clinics. To what extent will AI replace or aid in these roles?
Figure 2 Supply and demand for clinicians13
As the population ages, and the prevalence of chronic diseases continue to increase, demand on healthcare providers will grow. As shown in Figure 2, even within the next 10 years, demand for clinicians is set to outstrip supply. As well as improving patient outcomes, AI could provide a way of increasing efficiency. It may take over “low-hanging”, repetitive chores, freeing up doctors to spend more time on more challenging tasks. For example, machine vision and deep learning have been used in radiology to analyse images, passing on the most pressing images to the radiologist, while writing reports for unremarkable images itself.14 An example from the past is the implementation of automated blood pressure measurement and automated blood cell counts, which have not lead to deskilling or redundancy, rather clinicians now work on more interesting and complex tasks, with more time to interact with patients.
Haematologists will have more time to train for and carry out more complex procedures, such as bone marrow aspiration and intrathecal drug administration, which are unlikely to be automated. In addition, haematologists may be able to spend more time working on complementary interests, such as teaching or research. AI may aid clinicians in carrying out administrative tasks, currently loathed by many doctors. Systems using speech recognition and natural language processing may be able to listen in to consultations and write automated reports, leading to more comprehensive patient records.15 Developments like these are in their infancy, but show promise in reducing the clerical workload for all healthcare professionals.
In developing countries, healthcare providers with limited resources face the challenge of providing good quality healthcare to a growing population. AI could help meet this demand. For example, researchers have developed a mobile application that uses a smartphone camera to screen for anaemia. The app captures images non-invasively, through the patient’s skin, and then analyses them using a support vector regression model.16 Another example is the recently launched “robo-haematology” service, which uses a natural dialogue system that can explain the results of simple investigations to patients and can answer their questions.17 This type of service may not provide the same level of patient care as a healthcare professional, but in a resource-poor setting, it may prove a valuable addition to the multidisciplinary team.
There are several challenges that need to be addressed before machine learning decision support systems can be widely implemented. Machine learning models are only as accurate as the data they are trained on. There is always a risk of gaps and inaccuracies in data, especially in historic data derived before widespread adoption of electronic records. Furthermore, an estimated 80% of all medical data is unstructured.18 These records present a challenge to interpret, and conversion into machine usable format introduces another potential source of error. Despite attempts to standardise record keeping, such as ICD-10, medical records are still prone to ambiguity. This challenge is exemplified by IBM Watson, which has suffered from failures in oncology diagnosis, chiefly due to a lack of quality patient records to train on. However, this challenge will be overcome in time, as healthcare data is now being produced at a rapid rate; by 2020, estimates suggest that 94% of all medical data available will have been created after 2013.18
Another challenge is the difficulty of understanding how deep learning algorithms come to their decision. The multi-layered neural network is often viewed as a “black box”; there is often little understanding of how the algorithm was derived. The EU’s new General Data Protection Regulation (which will apply from May 2018) states that “Organisations that use ML to make user-impacting decisions must be able to fully explain the data and algorithms that resulted in a particular decision”.19 This is currently not possible in many machine learning systems. As well as the regulatory challenge, this poses an issue of trust; clinicians and patients may not have faith in recommendations from an opaque system which they cannot scrutinise. Furthermore, there is an issue of accountability. In the future, AI may play a part in a poor clinical decision, leading to harm to a patient. In such a circumstance, who is held accountable? The provider producing the model, the provider of the training data set, and the clinician checking the recommendations could all be considered responsible.
Patients with genetic diseases, such as sickle cell disease, haemophilia, and thalassaemia, often require lifelong care, and so can form a strong doctor-patient relationship with their haematologist. This relationship and support is hugely important for many patients, often just as much as the actual medical attention they receive. Some would argue that care dominated by automated systems would cause patients to feel like they are receiving cold, impersonal care. While using AI powered decision support systems, there is a risk of the patient being reduced to their medical history, instead of a unique human being with different preferences, thoughts, and psycho-social background. It is essential that even while using these tools, doctors continue to practise in a way which views patients holistically, treating them as individuals. This consideration means that doctors are unlikely to be entirely replaced, regardless of how medical artificial intelligence advances.
Doctors have a duty to provide the best care to patients. As such, the profession has a responsibility to ensure advancements like AI are used to their full potential, regardless of concerns of employment and clinical autonomy. History provides a positive outlook for haematologists and doctors in general; professions are not often made redundant by technology, instead they undergo redefinition to more human facing roles.20 I am excited to practise a new kind of medicine in the future, focusing on prevention and personalisation, leading to better care and healthier patients.
References
- Zini G. Artificial intelligence in Haematology. Haematology. 2005;10(5):393-400.
- Jiang F, Jiang Y, Zhi H, Dong Y, Li H, Ma S et al. Artificial intelligence in healthcare: past, present and future. Stroke and Vascular Neurology. 2017;2(4):230-243
- Deep Neural Network [Internet]. 2017 [cited 30 December 2017]. Available from: https://www.xenonstack.com/blog/log-analytics-with-deep-learning-and-machine-learning
- Casadio R, Compiani M, Fariselli P, Jacoboni I, Martelli P. Neural Networks Predict Protein Folding and Structure: Artificial Intelligence Faces Biomolecular Complexity. SAR and QSAR in Environmental Research. 2000;11(2):149-182
- Kantardzic M, Djulbegovic B, Hamdan H. A data-mining approach to improving Polycythemia Vera diagnosis. Computers & Industrial Engineering. 2002;43(4):765-773.
- HLS13-04 High-Content High-Throughput Screen for Diamond Blackfan Anemia Treatments | SBIR.gov [Internet]. Sbir.gov. 2017 [cited 28 December 2017]. Available from: https://www.sbir.gov/sbirsearch/detail/1030847
- Xu M, Papageorgiou D, Abidi S, Dao M, Zhao H, Karniadakis G. A deep convolutional neural network for classification of red blood cells in sickle cell anemia. PLOS Computational Biology. 2017;13(10):e1005746.
- Codella N, Moradi M, Matasar M, Sveda-Mahmood T, Smith J. Lymphoma diagnosis in histopathology using a multi-stage visual learning approach. Medical Imaging 2016: Digital Pathology. 2016;.
- Otake T. IBM big data used for rapid diagnosis of rare leukemia case in Japan | The Japan Times [Internet]. The Japan Times. 2017 [cited 29 December 2017]. Available from: https://www.japantimes.co.jp/news/2016/08/11/national/science-health/ibm-big-data-used-for-rapid-diagnosis-of-rare-leukemia-case-in-japan/
- Barbieri C, Molina M, Ponce P, Tothova M, Cattinelli I, Ion Titapiccolo J et al. An international observational study suggests that artificial intelligence for clinical decision support optimises anaemia management in hemodialysis patients. Kidney International. 2016;90(2):422-429.
- Pan L, Liu G, Lin F, Zhong S, Xia H, Sun X et al. Machine learning applications for prediction of relapse in childhood acute lymphoblastic leukaemia. Scientific Reports. 2017;7(1).
- Shouval R, Labopin M, Unger R, Giebel S, Ciceri F, Schmid C et al. Prediction of Hematopoietic Stem Cell Transplantation Related Mortality- Lessons Learned from the In-Silico Approach: A European Society for Blood and Marrow Transplantation Acute Leukaemia Working Party Data Mining Study. PLOS ONE. 2016;11(3):e0150637.
- Collier M, Fu R, Yin L. Artificial Intelligence in Healthcare [Internet]. Accenture.com. 2017 [cited 29 December 2017]. Available from: https://www.accenture.com/us-en/insight-artificial-intelligence-healthcare
- Aidoc Receives CE Mark for First Commercial Deep Learning Solution Streamlining Head and Neck Imaging for Radiologists [Internet]. Prnewswire.com. 2017 [cited 29 December 2017]. Available from: https://www.prnewswire.com/news-releases/aidoc-receives-ce-mark-for-first-commercial-deep-learning-solution-streamlining-head-and-neck-imaging-for-radiologists-660261973.html
- Halpern Y, Horng S, Choi Y, Sontag D. Electronic medical record phenotyping using the anchor and learn framework. Journal of the American Medical Informatics Association. 2016;23(4):731-740.
- Wang E, Li W, Hawkins D, Gernsheimer T, Norby-Slycord C, Patel S. HemaApp. GetMobile: Mobile Computing and Communications. 2017;21(2):26-30.
- ai [Internet]. Doc.ai. 2017 [cited 30 December 2017]. Available from: https://doc.ai/#products
- Patel A. Macroeconomic trends in the healthcare sector [Internet]. Merciatech.co.uk. 2017 [cited 30 December 2017]. Available from: https://www.merciatech.co.uk/news-media/insights/2017/dec/20/macroeconomic-trends-healthcare-sector/
- The European Parliament and the Council of the European Union. General Data Protection Regulation. Official Journal of the European Union; 2016.
- Autor D. Why Are There Still So Many Jobs? The History and Future of Workplace Automation. Journal of Economic Perspectives. 2015;29(3):3-30.
In 2016 the essay title was 'Discuss the impact of obesity on the incidence and management of haematological disorders'. The winner was Prateek Yadav from UCL Medical School and the runner-up was Maria Fala from the University of Cambridge.
Introduction
The obesity epidemic is one of the biggest healthcare challenges of our lifetimes. The WHO reports that obesity has more than doubled worldwide since 1980, By 2014 1.9 billion adults were overweight with 600 million of these classified as obese. This accounts for 39% of adults over 18 years of age being overweight worldwide. When looking at child and adolescent populations, it is estimated that 41 million children under 5 years of age are overweight worldwide. Most notably, developing countries and recently developed countries account for a large proportion of these; in 2014 almost half of overweight children under 5 lived in Asia. In addition, the number of overweight children under 5 years of age doubled in the African continent from 1980 to 20141. Both the rate of rapid development across the world and the increasing proportion of children who are obese indicate that this is not a problem that is going to go away; if anything, the pressures of obesity on our healthcare systems will only increase in the coming years. Furthermore, haematologists must be highly aware of how obesity and the associated metabolic syndromes interact with haematological conditions to provide high quality personalised care for patients. This essay looks at the interplay between obesity and haematological conditions and the impact on patients and our healthcare systems.
What drives the pro-coagulable state in obese patients?
Obesity is associated with hypertension, hyperlipidaemia and atherosclerosis, which are all risk factors for clotting events and obese patients are more likely to have difficulties with mobility, causing venous stasis. There are also a multitude of co-existing factors that create the increased clotting risk in the obese, and a plethora of hormonal disturbances and inflammatory reactions putting patients at a higher risk. Adipose tissue is an active endocrine organ, releasing cytokines such as leptin, adiponectin, interleukin-6 and tumour necrosis factor-α (TNF-α) that have subsequent effects on insulin resistance, haemostasis and inflammation. In terms of clotting components, obese patients tend to have higher circulating levels of fibrinogen, von Willebrand factor and plasminogen activator inhibition as well as factors VII and VIII2. These increased levels are likely to be produced by alterations in hepatic synthesis via pro-inflammatory cytokines. A single standard deviation increase in fibrinogen, in both men and women, was associated with a 20% increased incidence of primary cardiovascular events when adjusted for age and other risk factors2. Atherosclerotic disease is also promoted by increased expression of intercellular adhesion molecules such as ICAM -1 which are at higher levels in obese patients3.
Higher than normal levels of plasminogen activator inhibitor-1 (PAI-1) is regarded as part of the metabolic syndrome of insulin resistance and obesity4. High levels of PAI-1 is a risk factor for atherosclerosis and thrombosis, as it is the principle inhibitor of tissue plasminogen activator which in turn has a key role in fibrinolysis. It has been shown that adipocytes can produce PAI-1, which is a potential mechanism for increased coagulability in obesity and indeed waist circumference and PAI-1 levels correlate strongly. Weight loss in turn reduces PAI-1 levels but does not necessarily reduce fibrinogen levels, providing further evidence that PAI-1 is a key player in the higher clotting risk in the obese [2][5].
The inflammatory markers that are raised in obesity and insulin resistance, including TNF-α and transforming growth factor-β, have been shown to promote PAI-1 production5. This can contribute to the already significant production of PAI-1 by adipose tissue. These effects may be further compounded by disturbances in glucocorticoid and insulin levels in these patients, although the causal direction is unclear; there is some evidence to suggest that PAI-1 drives the development of insulin resistance rather than the other way around.
The leptin produced by adipose tissue increases thrombogenicity via a variety of potential mechanisms, including increasing platelet aggregation and von Willebrand factor levels3. Leptin also enhances calcification of the vasculature, providing targets for thrombogenic processes. The concentration of pro-inflammatory cytokines increases with adiposity; obesity is accompanied by a chronic inflammatory process. IL- 6 can be three times higher in obese patients than slim patients and it is thought to contribute to insulin resistance syndrome. The commonly measured inflammatory marker C reactive protein (CRP) has a strong association with obesity even accounting for age, race and smoking status differences6. CRP is independently associated with atherothrombotic risk and is an important direct link between obesity, inflammation and thrombotic risk. Raised CRP induces platelet adhesion to endothelial cells and interestingly, activated platelets convert pentameric CRP to its monomeric form, where it is more likely to capture neutrophils. This evidently makes up part of the atherosclerotic chain of events. Raised CRP levels also inhibit release of tissue-type plasminogen activator, which is important to produce the fibrinolytic substance plasmin, and upregulates release of PAI-1 from endothelial cells. Furthermore, in vitro experiments have shown CRP stimulates blood monocytes to produce tissue factor, which is a key part of the clotting cascade7.
Management of Haemostasis in Obese Patients
Evidence suggests that normal doses of thromboprophylaxis are suboptimal in morbidly obese patients and a trend towards higher doses of thromboprophylaxis may be seen. This is because, despite clinicians currently prescribing weight-adjusted doses for VTE treatment, the doses for thromboprophylaxis are often fixed dosage regimens. This regardless of evidence of a dose-response relationship for VTE prevention and is likely to be due to fears of bleeding risk. It has been shown that high dose thromboprophylaxis with heparin (7500 units TDS) or enoxaparin (40mg BD) nearly halved the incidence of VTE in the morbidly obese when compared to standard doses without increasing bleeding risk8. It is important to note that while this study has a very large sample size of 9241 and a good mix of patient settings, it has borderline statistical significance (p=0.050).
The tendency towards higher doses is compounded by the fact that stockings and pneumatic compression has reduced efficacy in the severely obese. More insight and clarity is needed into the specifics of dosage regimens in the obese for prevention of VTE as there are a large variety of dosages tested in studies. There are other practical considerations to consider, for example enoxaparin is often manufactured in pre-filled 40mg syringes which might make it difficult for early adopters of a 0.5mg/kg regimen or similar. It remains to be seen whether dosage regimens for the new direct oral anticoagulant drugs (DOACs) will need to be optimised for patient weight.
Knowledge of the pathways and mechanisms influencing the high risk of clotting events may open more obscure avenues to optimise patient care for prevention. For example, it is useful to note that decreases in PAI-1 activity are noted with ACE-inhibitors and angiotensin receptor blockers, along with diabetes drugs such as metformin and thiaizolidinediones9. This could potentially allow doctors to use synergistic drug combinations in comorbid patients in the future. Many patients at thrombotic risk are taking statins which have been shown to reduce CRP levels7 and in the future anti-inflammatory medications could potentially mitigate the inflammation mediated thrombotic risk in obese patients.
Obesity and Transfusion Medicine
A subject of much debate is whether being obese leads to increased numbers of surgical complications and therefore increased requirement for transfusions, or whether higher BMI and circulating volume protects against blood loss. The ‘obesity paradox’ of outcomes is the idea that overweight or obese patients may have better outcomes in certain situations than those who are of normal weight. The relationship between obesity and the need for perioperative transfusions is not clearly identified and much of the evidence is from low powered studies with no uniform definition of obesity across studies. In one recent study of elective procedures, namely total hip and total knee arthroplasties, patients who had an elevated BMI (>30) had decreased rates of blood transfusion (p=0.001). Patients with elevated BMI also lost significantly smaller percentages of blood volume perioperatively.10 While this example is apt, as arthroplasties are more common in the obese population due to increased risk of osteoarthritis, this is not a generalisable result.
The relationship between obesity and transfusion needs in trauma has not been fully established. It is possible to speculate that an obese patient might lose a lower percentage of their blood volume than a non-obese patient in comparable situations. However, patients who are obese are at higher initial risk of mortality after trauma mostly due to haemorrhagic and hypovolaemic shock.11 Risk in obese patients is compounded by the difficulty doing other procedures during resuscitation such as intubation and insertion of central venous catheters. A study looking at the frequency of massive transfusion in obese patients, with massive transfusion (MT) defined as 10 units of packed red cells in the first 24 hours, found that obese patients were more likely to need massive transfusion than non-obese patients with an odds ratio of 1.68 (95%CI 0.97 – 2.72), p = 0.07. The p value is relatively large, and the 95% confidence intervals overlap; obese patients had 15%(95%CI: 9-23%) receiving MT, non-obese 10% (95%CI: 8-12%) [11]. Despite the drawbacks to this study it might point to a new direction in transfusion protocol and while scores such as the Trauma Associated Severe Haemorrhage score (TASH) score do not mention BMI or weight, this might be a useful parameter in the future to assess transfusion demands in trauma patients.
Obesity and Benign Haematology
Despite associations with thin children with poor growth, conditions like sickle cell disease and thalassaemia now have drastically improved lifespans and prognoses and to see an obese patient with these conditions is not uncommon. Obesity complicates care as systemic changes in obesity add to the risk already present in these patients. The spectrum of complications that sickle cell anaemia can cause overlap significantly with risk factors obesity brings. Obesity and sickle cell both contribute to risk of stroke, cardiovascular events and vascular disease. In addition, obesity related hypertension combined with sickle cell anaemia can contribute to retinopathy and end-organ damage. It follows that these patients need close monitoring and careful management, and sickle cell patients of normal BMI do have lower admission rates than those with very high or low BMI.12 However, it was found that BMI has an overall inverse relationship with number of admissions. More research is needed in this area to see how metabolic changes in obesity will affect patients with benign haematological conditions.
Obesity and haematological malignancies
Obesity increases the risk of the haematological malignancies, including leukaemias, lymphomas and myeloma.13 If the obesity epidemic is not tackled successfully, demands on haemato-oncology services are going to increase in coming years. The distribution of risk is a dose-response relationship; increasing weight leads to increasing risk and vice versa. The meta-analysis of cohort studies found significant association between obesity and risk of developing non-Hodgkin’s lymphoma and myeloma. The evidence for increased risk of the leukaemia generally was significant in 11 out of 15 studies, however stratification into different subtypes was not carried out in most of the studies so specific information on these was not reported.13 However, a strong association between obesity and promyelocytic leukaemia was found.
The mechanism by which obesity increases cancer risk is not fully clear but two major theories are called the ‘inductive’ and ‘selective’ hypotheses. The former relies on inflammatory and metabolic changes in obesity encouraging neoplastic changes, while the latter hypothesises that the environment obesity produces selects in favour of already present abnormal cells that are dormant. Leptin produced by adipocytes induces cancer progression via the MAPK, PI3K and STAT pathways. In a similar way to the disturbances in haemostasis in obesity, proinflammatory cytokines including TNF-α, IL-2, IL-8 and IL-10 mediate increased cancer risk. Insulin and IGF-1 levels in obese patients is also implicated in cancer risk and mortality, thought to be acting via the AKT/PI3K/mTOR cascade. The fact that metformin reduces cancer incidence and mortality, but drugs that stimulate insulin secretion increase incidence and mortality, emphasizes the importance of insulin and IGF-1 in cancer biology.14
The presence of a malignancy drastically increases risk of clotting events and may produce a perfect storm in obese patients who are already high risk and much of the mortality and morbidity is accounted for by hypercoagulation.15 These patients need close attention to prevent VTE, cardiopulmonary events and stroke. There are further complications in that conditions such as type 2 diabetes, with high prevalence in the obese population, can modulate toxicity of cancer therapy regimens. The presence of diabetes significantly increases the risk of cardiotoxicity, from anthracycline treatment in patients with lymphoma, breast cancer and gastric cancer.16 Chemotherapy regimens may have to be adjusted to prevent adverse effects that are exacerbated by comorbid conditions, especially as cancer prognoses improve.
Obesity and Healthcare Funding
It is important to look at the way funding structures across healthcare systems change as the burden of obesity-related problems increases. In the UK, the direct cost to the NHS was estimated at £4.2 billion in 2007, with indirect costs estimates ranging to up to £15.8 billion.17 When compared to the estimate of cost in 1998, at 469.9 million, it puts into perspective the speed and magnitude of the growth in spending. Future projections of total indirect costs reach as high as £15.8 billion. This will put pressure on all branches of medicine, but haematology is a speciality that could be particularly affected due to the high costs of chemotherapy drugs for haematological malignancies. An instructive example of this is the drug ibrutinib for chronic lymphocytic leukaemia, which was not approved by NICE due to its high cost (£55.000 per patient) until a recent deal where prices were lowered for the NHS.18 As the obesity burden increases, healthcare providers may be forced to make difficult decisions about funding treatments and more of the financial burden of these expensive treatments may have to be shouldered by organisations such as the Cancer Drugs Fund. This will have a major impact on the range treatments available to haematologists and will affect how funding decisions are made in the future.
Conclusion
Obesity brings with it increased risk of VTE, cardiovascular and cerebrovascular events, and increasing obesity rates may change the landscape of thromboprophylaxis of the future. It will also be a strong driving force for innovations in this area, as seen recently with the development of the DOAC drugs. More investigation is needed into the interaction between obesity and benign haematological conditions, and patient management may have to change to account for greater risk. Obesity also increases the incidence of almost all the haematological malignancies and the associated metabolic syndromes may complicate management of cancer. Mechanistically, hormonal imbalances and inflammatory processes influence many of these risk increases and are still fertile areas for more research. The severe financial pressures obesity puts on healthcare services will force providers to make difficult decisions about resource allocation in the future, and may reduce the range of treatments available in the NHS to haematologists in the future.
References:
- World Health Organization, “Media centre Obesity and overweight,” January, pp. 1–5, 2015.
- I. Mertens and L. F. Van Gaal, “Obesity, haemostasis and the fibrinolytic system,” Obes. Rev., vol. 3, no. 2, pp. 85–101, 2002.
- L. F. Van Gaal, “Mechanisms linking obesity with cardiovascular disease,” Diabetes, Obes. Metab., vol. 12, no. December, p. 21, 2010.
- I. Juhan-Vague, M. C. Alessi, and P. E. Morange, “Hypofibrinolysis and increased PAI-1 are linked to atherothrombosis via insulin resistance and obesity.,” Ann. Med., vol. 32 Suppl 1, pp. 78–84, Dec. 2000.
- S. J. Appel, J. S. Harrell, and M. L. Davenport, “Central obesity, the metabolic syndrome, and plasminogen activator inhibitor-1 in young adults,” J Am Acad Nurse Pr., vol. 17, no. 12, pp. 535–541, 2005.
- M. Visser, L. M. Bouter, G. M. McQuillan, M. H. Wener, and T. B. Harris, “Elevated C-reactive protein levels in overweight and obese adults.,” JAMA, vol. 282, no. 22, pp. 2131–5, Dec. 1999.
- W. P. Fay, “Linking inflammation and thrombosis: Role of C-reactive protein.,” World J. Cardiol., vol. 2, no. 11, pp. 365–9, Nov. 2010.
- T.-F. Wang, P. E. Milligan, C. A. Wong, E. N. Deal, M. S. Thoelke, and B. F. Gage, “Efficacy and safety of high-dose thromboprophylaxis in morbidly obese inpatients.,” Thromb. Haemost., vol. 111, no. 1, pp. 88–93, Jan. 2014.
- M. Gnacińska, S. Małgorzewicz, M. Stojek, W. Lysiak-Szydłowska, and K. Sworczak, “Role of adipokines in complications related to obesity. A review.,” Adv. Med. Sci., vol. 54, no. 2, pp. 1–8, 2009.
- N. Frisch, N. M. Wessell, M. Charters, E. Peterson, B. Cann, A. Greenstein, and C. D. Silverton, “Effect of Body Mass Index on Blood Transfusion in Total Hip and Knee Arthroplasty.,” Orthopedics, vol. 39, no. 5, pp. e844-9, 2016.
- A. De Jong, P. Deras, O. Martinez, P. Latry, S. Jaber, X. Capdevila, and J. Charbit, “Relationship between obesity and massive transfusion needs in trauma patients, and validation of TASH score in obese population: A retrospective study on 910 trauma patients,” PLoS One, vol. 11, no. 3, pp. 1–15, 2016.
- M. W. Farooqui, N. Hussain, J. Malik, Y. Rashid, M. Ghouse, and J. Hamdan, “Prevalence of Obesity in Sickle Cell Patients,” Blood, vol. 124, no. 21, 2014.
- M. A. Lichtman, “Obesity and the Risk for a Hematological Malignancy: Leukemia, Lymphoma, or Myeloma,” Oncologist, vol. 15, no. 10, pp. 1083–1101, 2010.
- I. Vucenik and J. P. Stains, “Obesity and cancer risk: Evidence, mechanisms, and recommendations,” Ann. N. Y. Acad. Sci., vol. 1271, no. 1, pp. 37–43, 2012.
- G. J. Caine, P. S. Stonelake, G. Y. H. Lip, and S. T. Kehoe, “The hypercoagulable state of malignancy: pathogenesis and current debate.,” Neoplasia, vol. 4, no. 6, pp. 465–73, 2002.
- A. Gomes, L. Lopes, A. Ferreira, M. Correia, H. Mansinho, and H. Pereira, “The effect of cardiovascular risk factors and cancer type in anthracycline’s cardiotoxicity,” Eur. Hear. J. ( 2016 ) 37 ( Abstr. Suppl. ), 572, vol. 17 (Supple, p. ii269, 2016.
- L. Morgan and D. Monica, “The economic burden of obesity,” Natl. Obes. Obs., no. October, pp. 1–13, 2010.
- [18] “NICE reverses decision on CDF leukaemia drug after price drop | News and features | News | NICE.”
Obesity is currently one of the main challenges experienced by medicine. The obesity epidemic is constantly spreading, with the worldwide rates more than doubling in the last thirty-five years.2 Its consequences are numerous and diverse. Obesity has an impact on all systems of the body, including haematology. It is stratified in terms of the Body Mass Index (BMI), where a value of 20-25 kg/m2 is generally considered to be normal weight, 25-30 kg/m2 is overweight, 30-35 kg/m2 is obese and anything above 35 kg/m2 is superobese.2 Haematology is affected by obesity-driven systemic and local changes in the Bone Marrow, many of which are still unknown. This essay will explore these changes, their effect on haematological disorders and their management.
Haematological cancers form a large part of the haematological disorders in terms of their prevalence in the human population as well as their morbidity and mortality. Obesity has been found to increase the incidence and worsen the prognosis of many different types of cancers. It is, therefore, not surprising that haematological cancers are also affected by obesity.
Adipocytes constitute the most abundant cell type in bone marrow.6 They play an important role in the tissue microenvironment within the bone marrow. In obese patients, the number of adipocytes in the bone marrow is increased and their cytokine and lipid profile changes, affecting the neighbouring cells. For example, studies on obese mice have demonstrated that adipocytes release more leptin and inflammatory markers and less adiponectin and anti-inflammatory proteins.6 These chemicals activate various signalling cascades and lead to increased genomic instability, impaired DNA repair, tumour progression, local immunosuppression and epigenetic changes, all of which are detrimental for the patient.6 For this reason, adipocytes now constitute a potential therapeutic target for several cancers.
A cancer that has been found to be affected by obesity is Multiple Myeloma(MM). This is a plasma cell neoplastic tumour which constitutes 10% of haematological cancers.6 It is characterised by a clonal expansion of abnormal plasma cells in the bone marrow, osteolytic bone disease, anaemia and renal failure.5 It is currently incurable. Risk factors include older age, African ethnicity, family history and Monoclonal Gammopathy of Undetermined Significance (MGUS).6 Obesity has recently been identified as another important risk factor for multiple myeloma, causing an increase in incidence and mortality.6 A recent paper suggests that this increased risk is an indirect effect because obesity is, in fact, a risk factor for MGUS, which was found to be twice as common among obese patients compared to non-obese patients.3 MGUS is the stage which commonly precedes MM, in which an increase in the secretion of monoclonal immunoglobulin is observed without any accompanying myeloma features such as osteolytic changes.5
One of the mechanisms by which obesity is linked to increased risk of cancer is the communication between adipocytes and cancer cells, aiding tumour initiation, growth and metastasis.6 In a murine study, the role of adipocytes and the bone marrow microenvironment in tumour growth and progression has been demonstrated. 5T myeloma cells were inoculated in mice whose diet was varied. In mice on a high-fat diet, an increased amount of myeloma-specific IgG2bk para-protein was detected, before myeloma inoculation, suggesting that obese mice were in an obesity-driven MGUS state before tumour inoculation. They developed myeloma after the tumour was inoculated and the tumour burden was reduced once the high fat diet was removed. Collectively, these results suggested that the obese host provided a myeloma-permissive microenvironment for the myeloma cells to grow. Also, obesity did not have a direct effect on tumour growth and survival, but had an important role in enabling the initiation of the tumour.5 A possible driver for this change in the microenvironment is Insulin-like Growth Factor 1 (IGF-1) which was raised in obese mice, even before inoculation of the myeloma cells. Therefore, IGF-1 acts as a potent myeloma growth factor. Interleukin-6, on the other hand, commonly raised in cancer, only appeared to be raised in myeloma-bearing mice, suggesting that it is released by myeloma cells.6
Conversely, some study results suggest a role of the altered microenvironment in tumour growth and progression. One of the causal mechanisms for this relates to the increased amount of Interleukin-6 (IL-6) in the bone marrow microenvironment. IL-6 leads to activation of the STAT-3 signalling pathway, leading to increased proliferation and reduced apoptosis in monoclonal plasma cells.6 Additionally, tumour growth and progression is aided by the obesity-driven changes enabling better cell adhesion and angiogenesis. Better adhesion is correlated with the alpha-4 integrin, whose expression has been shown to increase in obesity.6 This offers increased proliferation, survival, migration and drug resistance to the tumour cells, which is why alpha-4 integrin blockers can restore sensitivity of the myeloma cells to bortezomib.6 Angiogenesis is enhanced in the obese state via two- to three-fold overexpression of Matrix Metalloproteinase 2 (MMP-2) in obese compared to normal.6
Another haematological cancer affected by obesity is Acute Myeloid Leukaemia (AML). This is the most common form of acute leukaemia in adults and is frequently fatal.14 Obesity is known to increase both incidence and mortality rates but research is still undergoing to investigate the pathogenesis mechanism. In a study undertaken using murine models, the leukaemia burden was shown to be higher in diet-induced obese mice.14 This was associated with higher levels of Fatty Acid Binding Protein 4 (FABP-4) and Interleukin-6 (IL-6) in serum. The Fatty Acid Binding Proteins are cytosolic intracellular receptors that can bind hydrophobic ligands and mediate lipid trafficking in the cell. FABP-4 is a good marker of obesity as it is highly expressed in adipocytes and macrophages of the obese. Upregulation of FABP-4 has been shown to promote tumour growth in AML and this study proposes a mechanism through which this occurs. They have shown that FABP-4 upregulation is correlated with increased amounts of IL-6, which leads to activation of the STAT-3 transcription factor. This transcription factor promotes DNA Methyl Trasferase-1 (DNMT-1) expression, overexpression of which leads to DNA hyper-methylation and silencing of Tumour Suppressor Genes such as p53. Aberrant DNA methylation has already been shown to be involved in AML, which makes the proposed mechanism highly likely.14
Non-Hodgkin’s lymphoma is a group of malignant diseases which originate from lymphocytes. The effect of obesity on the risk of non-Hodgkin’s lymphoma was investigated in a meta-analysis study which used the random effects model.4 This showed that obesity is associated with a statistically significant increase in the risk of non-Hodgkin’s lymphoma and in particular in the risk of diffuse large B-cell lymphoma. Overweight people were found to have a 7% higher risk and obese patients were found to have a 20% higher risk of developing the disease.4 The mechanism through which obesity increases the risk of this cancer is still unclear but several hypotheses exist. Altered immune function and chronic inflammation are risk factors for non-Hodgkin’s lymphoma, therefore this might be the mechanism via which obesity increases the risk of lymphoma. For example, the obesity-driven reduction in adiponectin can lead to such immune changes since adiponectin normally has anti-inflammatory effects and reduces lymphocyte proliferation. In contrast, leptin, whose levels are increased in obesity, enhances monocyte proliferation and hence the release of inflammatory cytokines such as Tumour Necrosis Factor-alpha (TNF-α). Another possible mechanism is related to the resistance to insulin which develops in obesity. As a result, the body produces more insulin which leads to a compensatory hyperinsulinaemia. This leads to elevated levels of IGF-1 which leads to increased cell proliferation and reduced apoptosis. This could also explain why Type II diabetes is also associated with an elevated risk for non-Hodgkin’s lymphoma.4
The coagulation and fibrinolytic cascades are very important cascades in haematology, as they determine a major property of the circulating blood, which is its tendency to clot. Obesity has been associated with higher levels of coagulation factors VII, VIII, IX, XII as well as the Von Willebrand Factor (VWF), tissue factor, fibrinogen and plasminogen activator inhibitor-1 and reduced levels of tissue plasminogen activator activity and activated protein C (APC) ratio.1,7 As a result of this imbalance, obesity leads to a hypercoagulable state.
These coagulation and fibrinolytic factors are affected by obesity via different mechanisms. A study investigating the effects of obesity on the expression of tissue factor, found that compensatory hyperinsulinaemia in obesity might play a role in inducing tissue factor overexpression.7 High insulin levels also lead to increased expression of Plasminogen Activator Inhibitor-1 (PAI-1), which also contributes to the hypercoagulable state. Thirdly, hyperinsulinaemia could also be the cause of the reduced Tissue Factor Pathway Inhibitor (TFPI) levels, leading to reduced inhibition of tissue factor activity in the clotting cascade.7
Based on the hypercoagulable state, a study has been undertaken to investigate the risk of bleeding in obese patients compared to normal weight patients, hypothesising that the hypercoagulable state would lead to a protection against bleeding in obese. However, even though the number of bleeding events was reduced in normal weight participants compared to underweight, the risk of bleeding in obese was not significantly different to the risk in normal weight participants.1
The hypercoagulable state in obesity is very important because it is a risk factor for thromboembolism. A study investigating the combined effect of obesity with other risk factors on the risk of thromboembolism has identified a number of interactions between risk factors.15 People with genetic mutations that predispose them to thromboembolic events, have a higher combined risk in obesity compared to the sum of the two individual risks. Also, obesity in combination with oral steroids increases the risk of venous thromboembolism. This is because obesity produces a pro-thrombotic state while oestrogen increases the resistance to activated protein C and further increases the concentration of factors II, VII, VIII and X, thus enhancing the effects of obesity.
Haemophilia A is a hereditary X chromosomal recessive haematological disorder which is related to the coagulation cascade.10 It is caused by a deficiency or a functional defect in clotting factor VIII, leading to impaired coagulation of blood and bleeding episodes, causing various problems such as limitations in joint range of motion due to haemorrhage in the joint. These episodes are prevented and controlled by intravenous infusion of Clotting Factor Concentrate (CFC). Several studies have been conducted to investigate the effect of the obesity-driven hypercoagulable state on the presentation of Haemophilia A. An increased net CFC usage was observed in obese patients compared to normal BMI patients, but this difference disappeared when adjusting the CFC usage for the patients’ weight.10 No difference was seen in FVIII activity between obese and non-obese patients, which is not surprising as even though obesity leads to overexpression of factor VIII, in Haemophilia patients this would not be possible, or the protein would be non-functional. Plasminogen Activator Inhibitor–1 (PAI-1) has been shown to be increased in in obese patients but led to no reduction in the need for coagulation.10 Additionally, for reasons that continue to be unclear, obesity has been found to be associated with further limitations in joint range of motion and further joint mobility loss compared to non-obese patients. More research needs to be undertaken in the effects of obesity on haemophilia, for correct dosing of Clotting Factor Concentrate, according to the patient’s weight, to be determined.
The coagulation imbalance is also important to consider when treating patients with anticoagulants. These are needed when thrombus formation is likely and needs to be prevented or treated. However, clinical trials for most of these drugs excluded obese participants. There are many uncertainties regarding dosing in obese patients due to lack of evidence. This is a serious problem, because obesity increases the risk of venous thromboembolism and the duration of inpatient stay. Studies have been undertaken to determine whether the current drug dosing recommendations are appropriate for obese patients.6 For venous thromboembolism prophylaxis, fixed doses of enoxaparin, dalteparin or tinzaparin are currently prescribed in the UK. However, this study provides evidence that enoxaparin and tinzaparin dose adjusted for BMI is more effective than the fixed dose6. The pharmacokinetics of each drug depend on its volume of distribution and its clearance, hence the effective dose and its dependence on the patient’s BMI is drug-specific. Therefore, mathematical models need to be applied to predict each drug’s behaviour.
Another systemic effect of obesity discovered, is the premature thymic involution that it causes. As a result, T cell generation is compromised and T cell apoptosis is increased leading to reduced thymocyte counts.16 Consequently, obesity leads to reduced peripheral naïve T cells, causing impaired immunity and increased susceptibility to persistent infections.
Lastly, obesity can affect other haematological disorders such as amyloidosis, a disease involving extracellular deposition of protein fibrils. In amyloidosis AA, the protein fibrils are derived from SAA, an acute phase protein produced by hepatocytes under inflammatory conditions. A study on the effect of obesity on amyloidosis revealed a positive correlation between body weight and degree of amyloidosis, suggesting that obesity can cause enhanced chronic secretion of SAA.11
Surgical interventions to treat morbid obesity can also affect haematology. Case reports have suggested that gastric bypass leads to microcytic anaemia due to reduced iron absorption. This is because iron absorption normally occurs in the bypassed duodenum and because iron chelation, that normally occurs when iron moves from the acidic environment to the alkaline duodenum and precipitates as a hydroxide, is impaired.13 Gastric bypass surgery also leads to reduced vitamin B12 levels, due to the loss of parietal cells which normally release intrinsic factor enabling vitamin B12 absorption in the distal ileum. Another case report presents the effects of total gastrectomy on haematology.8 In this case, the vitamin B12 deficiency led to high homocysteine levels, a risk factor for thrombophilia. Therefore, it might be useful to provide prophylaxis against thrombosis in patients undergoing such interventions.
Unfortunately, haematological conditions themselves are also related with obesity. Many debilitating diseases can lead to a sedentary lifestyle which can cause weight gain and obesity. It has been shown that patients suffering from childhood acute lymphoblastic leukaemia, gain excessive weight during and after two years of therapy.12 Previously, hypothalamic dysfunction due to cranial irradiation in treatment of the leukaemia was suspected to cause the increased rates of obesity in the survivors. However, a study on the causes of weight gain, has suggested that weight gain occurs even in the absence of cranial irradiation.12 Reduced total energy expenditure, mainly due to reduced energy expanded on activity is the main cause of obesity, meaning that measures can be taken to reduce obesity in these patients.
To conclude, medicine must face the serious problem of obesity. This is a challenge due to the many diverse effects that obesity has on health but also because of its increasing prevalence. In haematology, obesity constitutes a risk factor and affects progression of many diseases, including cancers, coagulopathies and amyloidosis. Research in this field needs to be continued to find out how to deal with these emerging problems and how current treatments need to be modified to accommodate obese patients. Importantly, preventative measures need to be taken in order to minimise the cases of obesity to avoid its destructive consequences.
References:
- Braekkan, S.K., Graaf, Y., Visseren, F.L.J. and Algra, A., 2016. Obesity and risk of bleeding: the SMART study.Journal of Thrombosis and Haemostasis, 14(1), pp.65-72.
- Bullwinkle, E.M., Parker, M.D., Bonan, N.F., Falkenberg, L.G., Davison, S.P. and DeCicco-Skinner, K.L., 2016. Adipocytes contribute to the growth and progression of multiple myeloma: Unraveling obesity related differences in adipocyte signaling.Cancer Letters, 380(1), pp.114-121.
- Landgren, O., Rajkumar, S.V., Pfeiffer, R.M., Kyle, R.A., Katzmann, J.A., Dispenzieri, A., Cai, Q., Goldin, L.R., Caporaso, N.E., Fraumeni, J.F. and Blot, W.J., 2010. Obesity is associated with an increased risk of monoclonal gammopathy of undetermined significance among black and white women.Blood, 116(7), pp.1056-1059.
- Larsson, S.C. and Wolk, A., 2007. Obesity and risk of non‐Hodgkin's lymphoma: A meta‐International Journal of Cancer, 121(7), pp.1564-1570.
- Lwin, S.T., Olechnowicz, S.W., Fowler, J.A. and Edwards, C.M., 2015. Diet-induced obesity promotes a myeloma-like condition in vivo.Leukemia, 29(2), p.507.
- Patel, J.P., Roberts, L.N. and Arya, R., 2011. Anticoagulating obese patients in the modern era.British journal of haematology, 155(2), pp.137-149.
- Samad, F., Pandey, M. and Loskutoff, D.J., 2001. Regulation of tissue factor gene expression in obesity.Blood, 98(12), pp.3353-3358.
- Shin, S.B., Jang, Y.N., Lee, H.J., Yi, Y.M., Lee, J.W., Min, W.S. and Eom, K.S., 2016. Thrombophilia after total gastrectomy for morbid obesity.The Korean journal of internal medicine.
- Silva, Simone Vargas, et al. "Obesity modifies bone marrow microenvironment and directs bone marrow mesenchymal cells to adipogenesis."Obesity 12 (2016): 2522-2532.
- Tuinenburg, A., Biere‐Rafi, S., Peters, M., Verhamme, P., Peerlinck, K., Kruip, M.J.H.A., Gorkom, B.A.P., Roest, M., Meijers, J.C.M., Kamphuisen, P.W. and Schutgens, R.E.G., 2013. Obesity in haemophilia patients: effect on bleeding frequency, clotting factor concentrate usage, and haemostatic and fibrinolytic parameters.Haemophilia, 19(5), pp.744-752.
- Van der Heijden, R.A., Bijzet, J., Meijers, W.C., Yakala, G.K., Kleemann, R., Nguyen, T.Q., de Boer, R.A., Schalkwijk, C.G., Hazenberg, B.P., Tietge, U.J. and Heeringa, P., 2015. Obesity-induced chronic inflammation in high fat diet challenged C57BL/6J mice is associated with acceleration of age-dependent renal amyloidosis.Scientific reports,
- Ventham, J.C. and Reilly, J.J., 1999. Childhood leukaemia: a model of pre-obesity.Proceedings of the Nutrition Society, 58(02), pp.277-281.
- Yale, C.E., Gohdes, P.N. and Schilling, R.F., 1993. Cobalamin absorption and hematologic status after two types of gastric surgery for obesity.American journal of hematology, 42(1), pp.63-66.
- Yan, F., Shen, N., Pang, J.X., Zhang, Y.W., Rao, E.Y., Bode, A.M., Al-Kali, A., Zhang, D.E., Litzow, M.R., Li, B. and Liu, S.J., 2016. Fatty acid-binding protein FABP4 mechanistically links obesity with aggressive AML by enhancing aberrant DNA methylation in AML cells.
- Yang, G. and Christine De Staercke, W., 2012. The effects of obesity on venous thromboembolism: A review.Open journal of preventive medicine, 2(4), p.499.
- Yang, H., Youm, Y.H., Vandanmagsar, B., Rood, J., Kumar, K.G., Butler, A.A. and Dixit, V.D., 2009. Obesity accelerates thymic aging.Blood, 114(18), pp.3803-3812.
In 2015 the essay title was 'Haematology and the ageing population – Implications for the practising haematologist'. The winner was Jess Dunphy and the runner-up was Jemma Proudfoot-Jones, both of the University of Birmingham.
Introduction
The United Kingdom, like the world as a whole, is home to an ageing population. Currently, there are over 11 million people in Britain aged 65 or over.1 The number of people aged 60 or over in Britain is now greater than the population aged 18 and under.1 Furthermore, it is predicted that by 2040 almost a quarter of the UK population will be over 65.2 The ageing population is likely to have a large impact on health services, both in terms of increased access to services and of financial cost to the National Health Service (NHS).3 This essay will examine the implications of an ageing population for haematologists. It will briefly discuss the ageing process, before exploring various aspects of haematologists’ work that may be impacted upon. These aspects include a greater need for shared care, considerations about treatment options, increased workload and ethical considerations.
What Happens as People Age?
The ageing process is characterised by “declining functional capacity and increasing vulnerability to disease, disability and death”.4 As people age, they are more likely to have multiple and complex conditions, including dementia and frailty.3 On average, an elderly person has 3 to 4 chronic illnesses.5 In terms of haematological conditions, longevity increases the risk of developing both haematological malignancies (such as myeloma and lymphoma) and non-malignant conditions (such as anaemia and susceptibility to coagulation disorders).6 As a consequence of multiple comorbidities, the elderly are also more likely to be on multiple medications than the younger population.3
The ageing process is driven by a gradual accumulation of both molecular and cellular defects that occur throughout our lifetime.4 One important aspect of ageing is “immunosenence”, the ageing of the immune system. This encompasses two main areas: decreased function of memory T cells and inflammaging.7
1. Memory T cells
As people age, the number of T-cells in the peripheral blood remains constant but there is a shift towards a greater production of memory T cells (rather than naïve T cells). This means that the elderly have a decreased capacity to respond to novel antigens. Furthermore, the memory T cells in the elderly function less well and have a less diverse repertoire than those in young adults, and so are able to respond less well to known antigens.7
2. Inflammaging
Inflammaging refers to clinical syndromes that are associated with ageing and include some form of inflammation (for example, atherosclerosis). It is thought that these age-related syndromes may be related to a loss of tolerance, either to self-antigens or to foreign antigens.7
Immunosenescene is important clinically because it means that elderly individuals are more predisposed to develop certain infections, or to develop more severe symptoms as a result of infections, than younger individuals. In addition, it is thought that the increased malignancy rate in the elderly, including haematological malignancies, may be due to a failure to provide immune surveillance to tumours.7
Another important facet of ageing for haematologists is the decline in function of haematopoietic stem cells (HSCs). Both the ability of HSCs to self-renew and to differentiate into mature blood lineages declines with age. This results from both intrinsic cell damage (such as DNA damage) and from changes to the micro-environment of the stem cell, including the aforementioned inflammatory changes that occur with age.8 Decline in HSC function may contribute to both myelodysplasia and, possibly, anaemia of the elderly.8
A Greater Need for Collaboration with Other Specialities
The first implication of an ageing population for practising haematologists is a greater need for collaborating with other specialities. The key speciality that haematologists need to liaise with to ensure optimum management of their elderly patients is geriatrics. One example of the importance of communicating with geriatricians is during the treatment of haematological cancer. A comprehensive geriatric assessment (CGA) is a process used to determine the health status of older patients, encompassing somatic, functional and psychosocial domains.9 A CGA can aid clinical decision making about the health status of an elderly patient with haematological cancer.9 Furthermore, it can result in the implementation of interventions which enhance a patient’s quality of life, such as measures to reduce social dysfunction and bodily pain.10 While a CGA does not have to be carried out by a geriatrician, the implementation of non-oncological interventions decreases considerably when left to the oncology specialist’s discretion rather than involving elderly care specialists.9,11 Thus, working with geriatricians can improve care of an elderly patient. As the size of elderly population increases, this collaboration between haematologists and geriatricians will occur more frequently.
Furthermore, the aforementioned increase in multiple comorbidities with age is likely to result in an increase in collaboration between haematologists and many other medical specialities, as well professions allied to medicine such as physiotherapy. There has been a drive within the UK to shift towards integrating care services, for example, merging health and social care services.3 Integrated care is coordinated, patient-centred care (rather than care focused on specific diseases), which aims to optimise patient health outcomes and satisfaction.3,12 There is no specific model of integrated care; the best approach is dependent upon local context. Certain models of integrated care, however, have been shown to improve both patient experience and care outcomes.3 Moreover, integrated care may be more cost-effective that delivering care based on single diseases. This may occur by reducing complications or reducing healthcare utilisation (which in turn frees up resources for other needs).3,11 This is important as average NHS spending for retired households is nearly double that for non-retired households.13 In addition, integrated care may reduce both gaps and duplication in service delivery, preventing repetition or delay in provision of services.3 This both reduces cost and improves patient satisfaction. For the haematologist, greater integration of care will therefore likely result in an increased collaboration with various medical professions. It should also reduce duplication or delay in seeing patients, resulting a more efficient service delivery.
Treatment Considerations
Additionally, age may impact on a haematologist’s management plans for patients. The prognosis of certain haematological conditions (for example, both acute and chronic myeloid leukaemias) decreases with age.14 The worsening prognosis is a result of both comorbidities (including an increased risk of other haematological disorders) and the biological ageing process. The increase in comorbidity with age results in an increase in polypharmacy.3 Furthermore, the pharmacodynamics and pharmacokinetics of drugs alter with age. Consequently, first-line medications for haematological diseases may be contra-indicated, less effective or cause more side effects in elderly patients.14,15 For example, vincristine, steroids and L-asparaginase – all potentially used in the treatment of acute lymphoblastic leukaemia – are generally more toxic in older patients.14 Problems with using the best available treatment do not just apply to medications. Elderly patients are also less likely than younger patients to be considered fit for certain procedures, such as stem cell transplantation, due to an increased likelihood of multiple and/or serious comorbidities.16 In short, multiple comorbidities and resultant polypharmacy, combined with a biologically poor prognosis, mean that haematologists may not always be able to offer first-line treatments to elderly patients. In addition, the treatments they do offer, even if first-line, may be less effective in elderly patients.
The consequence of an ageing population, therefore, is that more patients with haematological diseases may be receiving less effective therapy to treat or manage their disease. One potential result of this is an increase in research specifically targeted at the management of haematological conditions in elderly patients, which is currently lacking.14 Examples of potential research include new drug treatments or different treatment regimes for elderly patients. Any research of this nature could impact on all haematologists. Evidently, haematologists are likely to be involved in carrying out any such clinical trials. More generally, however, research of this nature may impact on treatment guidelines used by all haematologists.
Also, there is a possibility that normal laboratory reference values may differ between older and younger patients. Take, for example, the case of anaemia. Anaemia is common in older adults. It affects around 10% of the population over the age of 65, and the prevalence rises with age.8 There has been debate amongst experts about whether the World Health Organisation’s definition of anaemia (haemoglobin concentration <120g/L in women and < 130g/L in men)17 should be revised for people aged over 65. The increasing prevalence of anaemia with age suggests that perhaps a lower threshold for anaemia could be considered, however research has demonstrated that low haemoglobin concentrations in adults are significantly correlated with increased mortality and the development of physical, functional or cognitive decline.8 Should consensus on this issue be reached, it is likely that new guidelines on the diagnosis and management of anaemia will be released. Changes to this laboratory value (or many others) amongst the elderly would surely impact on a haematologist’s practice, for example, through alterations to patient management.
In terms of the impact of using age to determine patient management, it should be noted that the elderly are a very diverse group in terms of functional capacity. On the one hand, there are fit and healthy elderly people who do not have functional limitations or disabilities, despite the overall population increase in the prevalence of chronic disease (a process known as compression of morbidity).18 On the other hand, there are elderly people who age faster and die younger than others, partly due to differences in social environments.18 This marked heterogeneity in function means that a person’s physiological state, rather than their age per se, may be more important in making decisions about patient management.14,18 This is already reflected in management guidelines in the UK. For example, the British guidelines on treatment of acute myeloid leukaemia state that standard treatment should be offered to patients up to the age of 60 years. Above 60 years, however, the patient’s ability to tolerate intensive chemotherapy (for example, due to other comorbidities) must be considered.16 Consequently, as the population ages, the need for haematologists to make such decisions about capacity to tolerate treatment are likely to become more frequent. Overall, the variability in functional capacity amongst the elderly, including the need to consider comorbidities, is likely to result in haematologists delivering more individualised patient care.
An Increasing Workload for Haematologists
A further effect of an ageing population may be an increasing workload for haematologists, despite increasing numbers of both haematology trainees and consultants with the UK.19 The incidence of both haematological malignancies and non-malignant haematological conditions increases with age.6 Consequently, as the population ages, more people are developing haematological disease and need to be seen by a haematologist. Furthermore, the management of haematological diseases has become more complicated and/or more intensive and thus requires more of a haematologist’s time.20 In addition, the need to collaborate with other specialities and consider the (potentially complex) management of the patient’s other conditions further increases clinical workload.20 Elderly patients are especially likely to have complicated management plans as a result of comorbidities and reduced physiological reserve. Laboratory work is also increasing each year, which generates more new patient referrals and increases the demand for haematologists to give advice on how to interpret test results.20 Combined, all of these factors contribute to an increased clinical workload for the practicing haematologist. Perhaps in the future, integrating care will streamlines services and thus contribute to a reduction in the haematologist’s workload. At least in the short-term, however, haematologists are likely to have an increased workload. This is mainly the result of an increase in the number of patients needing to be seen by a haematologist and the increased complexity of patient management, especially in the elderly.
A Possible Increase in Ethical Issues
Also, treating more elderly patients may mean that haematologists are faced with more ethical issues. While ethical concerns may occur with patients of any age, they more frequently occur with older people. This is because conditions that impair understanding and decision making, such as dementia, are more prevalent within the elderly population.3,5 This may make it more difficult for doctors, including haematologists, to ensure that elderly patients have understood their management options and given informed consent for treatment.5 Furthermore, it may be difficult for haematologists to determine if some elderly patients have the capacity to make certain decisions, especially important decisions such as those surrounding end-of-life care.5 When the patient lacks capacity and has no advanced directive, the haematologist may be involved in deciding whether to withdraw life-sustaining interventions.5 As the population ages, more patients will potentially have conditions that could impair understanding and decision making. As a result, ethical issues surrounding consent and capacity will become more frequent for haematologists.
Conclusion
In conclusion, as the population ages, more people will develop haematological diseases. In addition, these people are more likely to have multiple medical conditions requiring treatment. Consequently, haematologists will need to liaise with other medical specialities, especially geriatrics, as well as professions allied to medicine more frequently than in the past. Furthermore, haematologists are more likely to need to create personalised management plans for patients, based on their age, functional status and comorbidities. This, along with the increase in haematological diseases, will probably result in an increased workload for haematologists. Additionally, haematologists may be faced with more ethical issues, such as those regarding consent, capacity and decisions about withdrawing life-sustaining interventions, as they treat more elderly patients. Overall, perhaps the biggest short-term impact of the ageing population for haematologists is not a change in the way that they manage elderly patients but rather an increase in the number of elderly patients that they encounter, and thus a greater need to consider the patient’s age as part of his or her management.
References
- Office for National Statistics. Annual mid-year population estimates, 2014 [online]. 2015 [cited 2015 Nov 14]. Available at: http://www.ons.gov.uk/ons/rel/pop-estimate/population-estimates-for-uk--england-and-wales--scotland-and-northern-ireland/mid-2014/stb---mid-2014-uk-population-estimates.html#tab-Main-Points
- Office for National Statistics. National population projections: 2014-based projections [online]. 2015 [cited 2015 Nov 14]. Available at: http://www.ons.gov.uk/ons/rel/npp/national-population-projections/index.html
- Oliver D, Foot C, Humphries R. Making our health and care systems fit for an ageing population. London, UK: The Kings Fund; 2014.
- Mitnitski A, Collerton J, Martin-Ruiz C, Jagger C, von Zglinicki T, Rockwood K et al. Age-related frailty and its association with the biological markers of ageing. BMC Medicine 2015; 13: 161-9.
- Mueller PS, Hook CC, Fleming KC. Ethical issues in geriatrics: a guide for clinicians. May Clin Proc 2004; 79: 554-562.
- Chomienne C, McCann S, Green T, Hagenbeek A, Lacombe C, Guenova M et al. Age and ageing in blood disorders: EHA theme of the year 2013-2014. Haematologica 2013; 98: 831-832.
- Suchard M. Immunosenescence: ageing of the immune system. S Afr Pharm J 2015; 82 (8): 28-31.
- Merchant AA, Roy CN. Not so benign haematology: anaemia of the elderly. Br J Haematol 2011; 156: 173-185.
- Hamaker ME, Prins MC, Stauder R. The relevance of a geriatric assessment for elderly patients with a haematological malignancy – a systematic review. Leuk Res 2014; 38 (3): 275-283.
- Rao AV, Hsieh F, Feussner JR, Cohen HJ. Geriatric evaluation and management units in the care of frail elderly cancer patients. J Gerontol A Biol Sci Med Sci 2005; 60 (6): 798-803.
- Kenis C, Bron D, Libert Y, Decoster L, Van P, Scalliet P et al. Relevance of a systematic geriatric screening and assessment in older patients with cancer: results of a prospective multicentre study. Ann Oncol 2013; 24 (5): 1306-1312.
- Nolte E, Pitchforth E. What is the evidence on the economic impacts of integrated care [online]. 2014 [cited 2015 Nov 15]. Available at: http://webcache.googleusercontent.com/search?q=cache:r-o-XD1Oo-MJ:www.kingsfund.org.uk/sites/files/kf/media/Ellen%2520Nolte%2520-%2520What%2520is%2520the%2520evidence%2520on%2520the%2520economic%2520impacts%2520of%2520integrated%2520care.pdf+&cd=1&hl=en&ct=clnk&gl=uk
- UK Parliament. The ageing population: key issues for the 2010 parliament [online]. 2010 [cited 2015 Nov 15]. Available at: http://www.parliament.uk/business/publications/research/key-issues-for-the-new-parliament/value-for-money-in-public-services/the-ageing-population/
- Hassan M, Abedi-Valugerdi M. Hematologic malignancies in elderly patients. Haematologica 2014; 99: 1124-1127.
- Golchin N, Frank S, Vince A, Isham L, Meropol S. Polypharmacy in the elderly. J Res Pharm Pract 2015; 4 (2): 85-88.
- Milligan DW, Grimwade D, Cullis JO, Bond L, Swirsky D, Craddock C, et al. Guidelines on the management of acute myeloid leukaemia in adults. London, UK: British Society for Haematology; 2006.
- World Health Organisation. Haemoglobin concentrations for the diagnosis of anaemia and assessment of severity [online]. 2011 [cited 2015 Nov 17]. Available at: http://www.who.int/vmnis/indicators/haemoglobin.pdf
- Suzman R, Beard JR, Boerma T, Chatterji S. Health in an ageing world – what do we know? Lancet 2015; 385: 484-6.
- Centre for Workforce Intelligence. Haematology: CfWI medical fact sheet and summary sheet [online]. 2011 [cited 2015 Nov 17]. Available at: http://www.cfwi.org.uk/publications/haematology-cfwi-medical-fact-sheet-and-summary-sheet-august-2011
- British Society for Haematology and Royal College of Pathologists. Haematology consultant workforce: the next 10 years [online]. 2008 [cited 2015 Nov 17]. Available at: https://www.rcpath.org/Resources/RCPath/Migrated%20Resources/Documents/P/pubhaem0108.pdf
Introduction
There are 11.4 million people aged 65 or above in the UK and this figure is expected to increase by around 40 per cent to over 16 million by 2032.1 Many haematological diseases are more prevalent in the older population, particularly haematological malignancies and their prevalence is expected to increase as the population ages. In this essay I will describe how age associated changes in haematopoiesis, inflammation and protein levels influence the presentation and management of haematological cancer, anaemia and disorders of haemostasis. The treatment of these conditions is complicated by the presence of comorbidities and frailty. However, the health status of the over 65 age group is highly heterogeneous and mean life expectancy at the age of 70 ranges between 6.7 and 18 years.2 This creates the dilemma of deciding which patients will tolerate aggressive treatment with curative intent and in which a palliative approach is necessary.
Haematopoiesis and ageing
The increase in haematological dysfunction with age is associated with a reduction in the number of haematopoietic stem cells and a decline in haematological cell function. Additionally there is preferential production of cells of the myeloid lineage, which may explain why CML almost exclusively occurs in adults. These observations are thought to be due to a complex interaction between genetic changes, changes in the bone marrow microenvironment and systemic inflammation.3 Most of these changes have been demonstrated in mouse models but the existence of the decline in haematopoietic function and proliferative capacity in humans is supported by the observation that bone marrow donor age is a risk factor in recipients.4 These haematological changes form the basis of immunosenescence of ageing which in turn causes reduced immune surveillance of malignant cells and increased development of cancer, including haematological cancers. The impaired stem cell pool reduces tolerance to chemotherapy and necessitates less intense treatment.
Haemoncology and ageing
The cumulative exposure to environmental insults and progressive telomere shortening that occurs with ageing facilitates the process of clonal evolution, which is driven by mutational changes. The understanding that initiation and progression of cancer requires several mutations or ‘hits’ supports this. In addition to this mutation-centric view, age associated changes in selection pressures such as a modified bone marrow microenvironment and immunosenescence promotes expansion of malignant clones.3 It therefore follows that, with the exception of ALL, the incidence of all haematological cancers peak in the elderly population.
Age is a major risk factor for all cancers. Additionally, the manifestation of haematological cancers differs between young and elderly patients so that older patients have a biologically inferior prognosis. In AML ageing is associated with an increased occurrence of adverse cytogenetic changes while cytogenetically normal patients show decreased incidence of favourable mutations e.g. NPM1 and increased occurrence of those that confer a worse prognosis e.g. FLT3.5 Similarly, the subtype of DLBCL associated with unfavorable outcome, activated B-cell like DLBCL, is increasingly more common with advancing age.6
The poorer outcomes in elderly patients can also be attributed to their reduced tolerance of aggressive therapies. The diminished stem cell population in the elderly increases the risk of febrile neutropenia due to the myelopsuppressive effect of chemotherapy. Prophylactic granulocyte colony stimulating factor is recommended where the risk of febrile neutropenia is >20%.7 Increased toxicity of chemotherapy in the elderly also results from altered pharmacodynamics; the age-associated decline in renal function may lead to increased toxicity. Additionally age associated comorbidities carry risk; anthracycline, the first line therapy for Non-Hodgkin lymphomas, is cardiotoxic and should be avoided in those with increased cardiac risk.8
Guidelines for treatment of haematological malignancies are disproportionately based on outcomes in younger patients rather than the population age group most likely to be affected. This is due to exclusion of patients with comorbidities from clinical trials. However, one of a very small number of trials focussing on patients >80 years of age, the R-miniCHOP trial, showed that full dose targeted CD20 monoclonal antibody Rituximab combined with low dose CHOP immunochemotherapy provided a trade off between efficacy and toxicity in DLBCL patients. This was the first study to show that reduced dose chemotherapy could improve survival in addition to reducing toxicity.9
The addition of Rituximab to CHOP had previously been shown to improve rate of complete response and overall survival in patients aged 60-80 in comparison to CHOP alone.10 The success of this targeted therapy in the elderly population is mirrored in the tyrosine kinase inhibitor imatinib that has revolutionised the treatment of CML. Survival of patients on imatinib does not significantly differ below and above 60 years of age, most likely because targeted therapies have fewer side effects and are therefore better tolerated by the older population.11 However, no molecular response is achieved where adherence to imatinib is below 80%. Adherance to medication is an issue of particular importance in the elderly outside of clinical trials, particularly if they live alone or if they are taking multiple daily medications.12
Where targeted therapies are not yet readily available, for example multiple myeloma, the redeployment of already licenced drugs with a lower toxicity profile is providing a cost effective alterative to reducing toxicity of treatment. The use of thalidomide to treat myeloma has extended median survival to 4-5 years. More recently the screening of 100 off-patent drugs identified an antihelminth Niclosamide as a potential anti-myeloma therapy.13 Myeloma remains incurable and 85% of cases occur in over 60 year olds.13 Myeloma therefore represents a growing problem as the population ages and advances in treatment are highly anticipated.
Considerable debate surrounds the potential use of reduced intensity allogeneic stem cell transplant (SCT) in myeloma along with other haematological malignancies. Reduced intensity allogeneic SCT exploits the graft versus tumour effect of donor T lymphocytes not seen in autologous SCT but is preceded by considerably less myeloablative conditioning with chemotherapy and radiotherapy. It is therefore better tolerated in older patients. A study comparing reduced intensity allogeneic SCT with standard chemotherapy in patients aged 60-70 showed a 49% lower rate of relapse at 3 years in the SCT arm.14 However, graft versus host disease is still a significant risk.
Some caution should be executed in interpretation of trials in elderly patients. The sample may not be representative of the age group due to exclusion criteria although the therapy may be successful in a carefully selected group of patients. The challenge then lies in identifying which individuals are suitable for high intensity chemotherapy or SCT in a clinical setting. There is some evidence for the use of comprehensive geriatric assessment (CGA) to stratify patients. A retrospective study comparing survival of patients identified as ‘fit’ by CGA and survival of patients selected for treatment with curative intent by clinical judgement increased survival in those identified by CGA.15 However CGA can be time consuming and assessment of activities of daily living which have been shown to correlate strongly with overall survival in Non-Hodgkin Lymphoma, may be a more efficient and cost-effective alternative.2
Anaemia and ageing
Anaemia is extremely common in the elderly, occurring in 10% of adults over 65 and 20-30% of adults aged over 85. There is a strong link between anaemia and morbidity and mortality and it has been correlated with a decline in physical, functional and cognitive functioning. In the elderly this may translate to an increased fall risk. Anaemia is defined as a haemoglobin concentration of <120 g/l in women and <130 g/l in men. There has been considerable debate as to whether this figure should be adjusted for the elderly population. Some believe that the cut off should be raised given that some functional issues are noted at borderline anaemic levels while others suggest that the limit should be lowered to modulate the raised occurrence of anaemia with age. Further investigation into the effect on morbidity and mortality of a range of haemoglobin levels is required to settle this dispute.(16)
Around one third of anaemic individuals over 65 have anaemia due to a nutritional deficiency. Iron deficiency anaemia is the most common nutrient deficiency and is most commonly due to gastrointestinal bleeding. This may suggest malignancy and requires further investigation. Folate deficiency is common in the elderly due to relative malnutrition. Access to fresh fruit and vegetables may be difficult for elderly people particularly for those living alone or in residential care.(16) A further third of anaemia over 65 is due to anaemia of chronic disease, which occurs alongside conditions characterised by long-term inflammation such as malignancy or rheumatoid arthritis. The inflammatory cytokine IL6 stimulates release of hepcidin from the liver. Hepcidin triggers internalisation and degradation of ferroportin, the protein responsible for transporting iron out of cells. Iron becomes trapped, particularly in macrophages, and is therefore unavailable.17
The remaining third of the anaemic elderly are labelled with the term ‘unexplained anaemia of the elderly’ for which there is no guidelines for management. A proportion of this group may be explained by an inflammatory state of ageing which appears to have some overlap with anaemia of chronic disease. Some studies have noted raised hepcidin and ESR levels in unexplained anaemia of the elderly while IL6 levels have been found to be both raised and reduced compared to controls on separate occasions. Unexplained anaemia of the elderly may be partially explained by the observed insensitivity of HSCs to erythropoietin with ageing and the declining and dysfunctional haematopoietic stem cell population, which have reduced potential for erythropoiesis.16 These mechanisms are speculative and further research into the aetiology of anaemia in the elderly is necessary.
Anaemia appears to be a strong marker for physical decline but should anaemia of chronic disease and unexplained anaemia be treated? The most effective means of raising haemoglobin levels is through blood transfusion. However, in elderly patients with cardiac failure this is not appropriate given the risk of transfusion associated circulatory overload. Given that unexplained anaemia is typically more mild than iron deficiency anaemia, improving haemoglobin levels may not significantly improve function as any improvement may be negated by other factors associated with ageing such as reduction in muscle mass and decline in pulmonary and cardiac function.16 However, it may be dangerous to simply attribute anaemia to ageing without investigation, as a reduced haemoglobin level could be an indication of serious pathology.
Haemostasis and ageing
The increased risk of thrombosis with age is reflected in changes of components of the haemostatic system. Coagulation system proteins including FV, FVII, FVIII, FX and fibrinogen increase with age. Fibrinogen and VIII are acute phase proteins and fibrinogen production is in fact stimulated by IL6, suggesting that the inflammatory state of ageing may in part underlie these observed changes in coagulation factors. Fibrinogen levels have been identified as a risk factor for stroke and MI while increased FVIII has been shown to correlate with risk of venous thrombosis. In addition to these changes, fibrinolysis in the elderly is impaired as evidenced by the increased levels of plasminogen activator inhibitor (PAI-1) which inhibits fibrinolysis. PAI-1 is itself another acute phase protein. A corresponding increase in anticoagulant proteins with age has not been observed and therefore this imbalance could shift the haemostatic system towards a hypercoagulable state.18
The increased thrombotic risk with age necessitates continued emphasis of VTE prophylaxis in hospitals. Additionally a greater proportion of the population will require oral anticoagulants as the population ages. Elderly patients taking Warfarin require more frequent monitoring of INR as they are more susceptible to bleeding side effects.19 Increased monitoring by pharmacists and self-monitoring may be necessary to help the haematology profession deal with the increased demand on their anticoagulation clinics.
Contrastingly, population ageing also has implications for management of haemophilia patients. Previously a childhood disease, haemophilia now has an almost normal life expectancy due to advances in treatment with the development of recombinant factor VIII and IX in the 1990s. Therefore the haematology specialty must adapt to expect more elderly patients with haemophilic arthropathy and associated chronic pain. Additionally, there is a large population of patients who contracted hepatitis C and HIV via coagulation factor transfusion before viral inactivation of blood products became routine who are only now reaching old age. Before purification of blood products, 80-90% of haemophilia patients were infected with hep C. Patients coinfected with Hep C and HIV are twice as likely to develop cirrhosis and liver failure than those infected with Hep C alone. Studies suggest that around 1/3 of haemophilia patients are depressed and therefore there is a need for a psychiatric component of care.20
Conclusion
Elderly patients with haematological cancer have a worse prognosis due to comorbidities and their impaired tolerance of chemotherapy. Management of haematological malignancy in the elderly often requires less toxic treatment. However, management decisions should be made on a case-by-case basis and take into account the biological in addition to the chronological age of the patient. Formal geriatric assessment may aid selection of good candidates for intensive treatment and cooperation with geriatric doctors facilitates provision of sufficient nutrition and social support, which may influence outcome. Significant treatment advances have been made in some cancers; CML is now extremely treatable. However, others such as myeloma lag behind and will represent a significant burden as the population ages until more targeted therapies are available. There is desperate need for increased recruitment of patients over 75 into cancer trials. Only then can evidence based treatment decisions be made for this age group. Guidelines for target haemoglobin levels in the elderly and treatment of unexplained anaemia are required based on evidence of patient functional ability. The elderly are inherently thrombotic although age is a risk factor for bleeding for those anticoagulated on warfarin. An emerging cohort of elderly virally infected haemophilia patients exists and management must be adapted to accommodate their associated comorbidities. Inflammation and haematopoietic decline are recurrent themes that underlie haematological dysfunction in ageing and further research into these areas will aid management in the future.
References
- Later Life in the United Kingdom 2015 [updated 7 December 2015]. Available from: http://www.ageuk.org.uk/Documents/EN-GB/Factsheets/Later_Life_UK_factsheet.pdf?dtrk=true. (accessed 30 December 2015)
- Peyrade F, Gastaud L, Ré D, Pacquelet-Cheli S, Thyss A. Treatment decisions for elderly patients with haematological malignancies: a dilemma. The Lancet Oncology. 2012;13(8):e344-e52.
- Henry CJ, Marusyk A, DeGregori J. Aging-associated changes in hematopoiesis and leukemogenesis: what's the connection? Aging (Albany NY). 2011;3(6):643-56.
- Kollman C. Donor characteristics as risk factors in recipients after transplantation of bone marrow from unrelated donors: the effect of donor age. Blood. 2001;98(7):2043-51.
- Kumar CC. Genetic abnormalities and challenges in the treatment of acute myeloid leukemia. Genes Cancer. 2011;2(2):95-107.
- Mareschal S, Lanic H, Ruminy P, Bastard C, Tilly H, Jardin F. The proportion of activated B-cell like subtype among de novo diffuse large B-cell lymphoma increases with age. Haematologica. 2011;96(12):1888-90.
- Aapro M CJ, Kamioner D. Prophylaxis of chemotherapy-induced febrile neutropenia with granulocyte colony-stimulating factors: where are we now? Support Care Cancer. 2010;18(5):529-41.
- Aapro M, Bernard-Marty C, Brain EG, Batist G, Erdkamp F, Krzemieniecki K, et al. Anthracycline cardiotoxicity in the elderly cancer patient: a SIOG expert position paper. Ann Oncol. 2011;22(2):257-67.
- Peyrade F, Jardin F, Thieblemont C, Thyss A, Emile J-F, Castaigne S, et al. Attenuated immunochemotherapy regimen (R-miniCHOP) in elderly patients older than 80 years with diffuse large B-cell lymphoma: a multicentre, single-arm, phase 2 trial. The Lancet Oncology. 2011;12(5):460-8.
- Coiffier B, Lepage E, Briere J, Herbrecht R, Tilly H, Bouabdallah R, et al. CHOP chemotherapy plus rituximab compared with CHOP alone in elderly patients with diffuse large-B-cell lymphoma. N Engl J Med. 2002;346(4):235-42.
- Gugliotta G, Castagnetti F, Palandri F, Breccia M, Intermesoli T, Capucci A, et al. Frontline imatinib treatment of chronic myeloid leukemia: no impact of age on outcome, a survey by the GIMEMA CML Working Party. Blood. 2011;117(21):5591-9.
- Marin D, Bazeos A, Mahon FX, Eliasson L, Milojkovic D, Bua M, et al. Adherence is the critical factor for achieving molecular responses in patients with chronic myeloid leukemia who achieve complete cytogenetic responses on imatinib. J Clin Oncol. 2010;28(14):2381-8.
- Khanim FL, Merrick BA, Giles HV, Jankute M, Jackson JB, Giles LJ, et al. Redeployment-based drug screening identifies the anti-helminthic niclosamide as anti-myeloma therapy that also reduces free light chain production. Blood Cancer J. 2011;1(10):e39.
- Champlin R. Reduced-intensity allogeneic hematopoietic transplantation should be considered a standard of care for older patients with acute myeloid leukemia. Biol Blood Marrow Transplant. 2011;17(12):1723-4.
- Tucci A, Ferrari S, Bottelli C, Borlenghi E, Drera M, Rossi G. A comprehensive geriatric assessment is more effective than clinical judgment to identify elderly diffuse large cell lymphoma patients who benefit from aggressive therapy. Cancer. 2009;115(19):4547-53.
- Merchant AA, Roy CN. Not so benign haematology: anaemia of the elderly. Br J Haematol. 2012;156(2):173-85.
- Ganz T. Hepcidin, a key regulator of iron metabolism and mediator of anemia of inflammation. Blood. 2003;102(3):783-8.
- Franchini M. Hemostasis and aging. Crit Rev Oncol Hematol. 2006;60(2):144-51.
- Shoeb M, Fang MC. Assessing bleeding risk in patients taking anticoagulants. J Thromb Thrombolysis. 2013;35(3):312-9.
- Canaro M, Goranova-Marinova V, Berntorp E. The ageing patient with haemophilia. Eur J Haematol. 2015;94 Suppl 77:17-22.
In 2014 the essay title was 'Does the 21st century haematologist need a microscope?'. The winner was Tom Handley of the University of Oxford and the runner-up was Kiloran Metcalfe, of the Oxford Medical School.
A haematologist, from the Greek haima "blood" and logos “to study”, studies the blood and haematopoietic tissues of the body, in order to diagnose disorders of blood components.1 The study of blood permeates medicine, and the microscope is an iconic tool that is bound to our perception of the field. The fate of this instrument is dependent on a technological arms race, where more recent technology has the potential to render the microscope’s place as a primary diagnostic tool obsolete. This essay will discuss haematology’s past, present and conceivable future. It will explore the microscope’s precarious position in haematology, representative of potential paradigmatic shifts in healthcare over the next hundred years.
What is the role of a haematologist in the 21st century?
A haematologist’s principal concern is the accurate identification of disease caused by (or relating to) disorders of the blood. A modern haematologist can split their time between the laboratory and the clinic, deriving the aetiology of blood-based disease through laboratory diagnostics, and implementing treatments as a clinician. In order to understand what is aberrant, they must first fully understand what is normal. This requires a comprehensive understanding of the cells and tissues of the blood system. In order for blood to fulfil its function in the circulatory system, the presence and relative abundance of the cellular and proteinaceous components of the solution must be maintained to ensure continued physiological function. However, these components are too small to be seen by the naked eye, and their interactions too fleeting and complex to be understood visually. We must therefore use tools to analyse the blood’s morphological and functional characteristics.
Microscope: A tool that founded a field
An informed diagnosis requires an understanding of the cellular appearance and physiological performance of the blood. The microscope enables haematologists to understand the constituent parts of the blood. It allowed the field to develop beyond philosophical ponderings about the substance of this life giving fluid, and visualise what lay within the murky liquid. The microscope gives an image of the subject at a higher magnification and resolution than can be seen by the naked eye, founding a morphological understanding of the cellular composition of blood.
Microscopes were discovered in the late 16th century, but the name was first used to describe Galileo’s compound optical microscope in the 17th century.2 When a generation of scientists led by Van Leeuwenhoek in the 17th century studied living organisms using this optical microscope, a new world opened before their eyes. The “mikros” (small) “skopeîn” (to view) allowed them to discover the world of microbiology3, and with it tools and information that would become pivotal to haematology. Being able to image cells for the first time became one of the most important developments in life-sciences, and allowed scientists to push the boundaries of how they understood the world.
However, it would be another 150 years before other scientists used the microscope to further their understanding of the component parts of the blood beyond Leeuwenhoek’s ‘Red Corpuscles’.4 The 19th century saw the discovery of white cells and platelets. Osler, along with Schultze and Bizzozero are among a number of scientists who contributed to the discovery of platelets.5 The gap between the first discovery of the cellular organisation of the blood and a more comprehensive understanding of its component parts was due in part to the development of the microscope as a tool to study the blood. Traditional light microscopy is limited by the quality of illumination, the magnification capacity of the lens, and the staining techniques that are employed. Biological tissue has little inherent contrast under a microscope6, and thus requires staining to both give contrast to the tissue and highlight particular features of interest. Improvements in the technological capabilities of the microscope enabled a much greater morphological understanding of the blood, its appearance in vitro, and some of the basic pathologies.
How is a microscope used today?
Microscopes are used to view cells in an in vitro environment (for example in a thin blood smear). This allows some conclusions to be drawn about their physiological state in the body (for example, an elevated number of white cells in a sample may be indicative of an infection in vivo). Today, the microscopic appearance of cells is used to create a picture of how the cells may be performing in the body. Aberrant shapes characterise a multitude of diseases, and fill our textbooks and journals with examples of different states of disease. Microscopes are particularly useful in circumstances where a rapid diagnosis is important, for example, the initial stage of diagnosing acute leukaemias uses a morphological analysis, enabling fast initiation of treatment.7
The 20th century brought great technological advancements, including the development of a new way of studying cells with microscopes using electrons, rather than beams of light. As microscopy techniques moved toward greater levels of magnification and higher levels of resolution we began to understand more about the morphological characteristics of the cells and tissues of the body. Clinicians and scientists also began to employ diagnostic techniques that did not require imaging of the cells, but were based on molecular signals too small and complex to be detected by the microscope.
What technologies may supersede the microscope?
A modern haematologist’s task is to quickly detect a wide range of diseases and disorders, often in a large number of patients. In order to do this, machines have been designed to detect the presence of molecular markers correlated with sequences that are specific to a certain condition, or to a mutation that is related to a certain prognosis (for example JAK2 mutations linked with poor prognosis in myeloproliferative disorders8). The myriad of machines that have been developed to assess such molecular parameters have helped us to develop better markers, tactics and techniques for detecting disease. A molecular approach enables ‘personalised medicine’, where the treatment of disease is specific to the patient.
This is useful, for example, in some cancers where specific protein mutations can lead to different treatment regimes. Perhaps the classic example of this hails from haematology – specifically the treatment Chronic Myeloid Leukaemia. David Hungerford’s discovery of the bcr/abl (Philadelphia) chromosomal translocation allowed the development of a targeted treatment for CML. In the 1990’s the tyrosine kinase imatinib was developed, which blocks the BCR-Abl enzyme, and stops it from adding phosphate groups. As a result, these cells stop growing, and can commit apoptosis9,10. One could argue that the goal of personalised medicine is to re-create this achievement for other conditions.
Genomics uses gene sequencing and bioinformatics to analyse genomes and investigate the roles different polymorphisms can play in disease. Genomics may well be the next iteration of personalised medicine, and could enable the development of treatments specific to an individual’s disease. The recent announcement of a program to sequence 100,000 genomes within three years at 11 centres across the U.K. aims to develop new tests and drugs and is a sign of rapidly developing research on a molecular level.11
This program is symbolic of a movement in the medical field, which is no longer so focused on a morphological relationship between form and function, but rather has adopted a ‘black box’ methodology. A doctor or scientist may take a sample from a patient and present this sample to a machine. This machine then runs a number of tests on the sample designed to detect minute differences between cells, and generate a (usually quantitative) response. Even the strongest microscopes are unable to resolve differences at these levels. Such machines generate a series of results from an array of molecular diagnostic machinery to come to a relatively binary decision as to whether a patient has ‘disease X’ or not.
However, these machines are not always available to clinicians, and as such haematology is in a transitional period. Exclusive use of the microscope is not practical in some clinical settings, as better molecular options can provide more conclusive and specific diagnoses; however these molecular techniques cannot be solely used, as they are expensive and slow, and not yet widely distributed. Will the 21st century change the way technology is used, and will there continue to be a place in the haematologist’s toolbox for the microscope?
The microscope’s uncertain future
Regardless of the obvious merits of molecular diagnostics, microscopes are still used in clinics and continue to be critical to the cutting edge of research. The 2014 Nobel Prize for Chemistry was jointly awarded to Hell, Betzig & Moerner for the development of super-resolved fluorescence microscopy, surpassing the previous limitations of the light microscope.12 They had separately found different solutions to pass Abbe’s diffraction limit, the point that had previously limited the resolution of light microscopes. By bypassing this physical limitation on imaging, higher resolution images can now be made of living cells.
Such advances in microscope technology indicate that the potential exists for even greater development in this area. There are numerous ways that microscopy could be manipulated to provide novel findings, from advances in staining techniques to further revisions in the limits of resolution. Indeed, in some areas of research intra-vital microscopy is enabling real-time in vivo visualisation of the interactions between cells. Ronald Germain and his colleagues have been able to challenge the immune system and visualise the consequences in the lymph nodes13. Perhaps advances in techniques in these areas may enable us to image cells in their physiological environment.
Advances in these areas may be symbolic of revolutions in the field of microscopy. The microscope of the future may be as different to today’s as our microscopes, with their beams of electrons and high fluorescence multi-coloured images, would seem to Galileo or Van Leeuwenhoek. The advances that the next hundred years may bring could allow us to visualise not only cells, but proteins on cells. Enabling analysis of morphological appearance, and real-time changes in response to physiological or test interventions. A “mikros” (small) “skopeîn” (to view) may enable us to see things that today we cannot even imagine.
The response to this question also depends on where one sits in the world. In many Western medical systems a movement toward increasingly complex and expensive technologies persists, with complex molecular analyses of many conditions leading the spear-tip of research. However, in the developing world access to haematological facilities and molecular diagnostic laboratories is rare, or even non-existent. Many developing countries use basic equipment to provide the fundamentals of haematological analysis and diagnosis. Microscopes are essential under these circumstances. They are cheap, easily transportable, and efficient in the hands of a skilled technician. However, if molecular diagnostics were to continue to miniaturise and become even cheaper, there is a chance that they could leapfrog microscopy to become the most prevalent diagnostic technique.
Conclusion
The fate of the microscope in haematology raises a more philosophical point about how we are going to treat patients in the future. If a haematologist no longer needs to use a microscope, they may no longer be basing their diagnoses upon the relationship between morphological form and pathological dysfunction that the field was founded upon. Instead, they may come to rely entirely upon a series of machines and analytical tests. The laboratory-based side of a haematologist’s work would move further towards the bioinformatic world, caught up in the rush of a new era of genomic science.
Currently, it is vital for a haematologist to learn how to use a microscope, as a great deal of the diagnostics and protocols currently in place require an ability to act rapidly and effectively. As healthcare in the world develops, different healthcare systems will progress from using the microscope at different rates, and thus its use will certainly not cease in the immediate future. Some older medical technologies have been cast aside once they were superseded by new inventions (such as the cane once used by physicians to ward off the miasmas of their patients)14. However, unlike some of the technologies history has cast aside, microscopes have had decades of empirically proving their worth in the medical field, and so are unlikely to meet the same obscure fate.
Does the 21st century haematologist need a microscope? At the moment, they do. However, over the course of the next 85 years of our century, a technological arms race may change the face of medicine. A new, exclusively molecular, branch of medicine may appear in the place of the discipline we currently call haematology. New technologies within such a discipline may entirely supersede the humble microscope, or they may simply work alongside it. Science is a discipline in constant flux, where it is nigh on impossible to stipulate the importance of a tool in a future environment that cannot be predicted. In the immediate and foreseeable future, however, microscopes will continue to be used by haematologists, inform a large portion of their diagnostic work, and remain rooted in the field of haematology.
References
- NHS cited from [Internet].; cited [November 2014] Available from: www.NHScareers.nhs.uk
- Gould, Stephen Jay (2000). "Chapter 2: The Sharp-Eyed Lynx, Outfoxed by Nature". The Lying Stones of Marrakech: Penultimate Reflections in Natural History. New York, N.Y: Harmony.
- Wootton, David (2006). Bad medicine: doctors doing harm since Hippocrates. Oxford [Oxfordshire]: Oxford University Press. ISBN 0-19-280355-7
- M. Bessis and G. Delpech.(1981), Discovery of the Red Blood Cell with notes on priorities and credits of discoveries. Blood Cells 7:447-48
- Max Schultze (1865), G. Bizzozero (1882) and the discovery of the platelet, 2006 Blackwell Publishing Ltd, British Journal of Haematology, 133, 251–258
- Young, B., Heath, J. W., Stevens, A., Lowe, J. S., Wheater, P. R., & Burkitt, H. G. (2000). Wheater's functional histology: A text and colour atlas. Edinburgh: Churchill Livingstone.
- Bennet, J (1985) Proposed Revised Criteria for the Classification of Acute Myeloid Leukemia: A Report of the French-American-British Cooperative Group. Ann Intern Med. 1985;103(4):620-625
- Peter J. Campbell (2006) V617F mutation in JAK2 is associated with poorer survival in idiopathic myelofibrosis; Blood: 107 (5)
- Hungerford et al. (1960) Chromosome preparations of leukocytes cultured from human peripheral blood. Experimental Cell Research Volume 20, Issue 3, Pages 613-616
- Goldman JM, Melo JV (October 2003). "Chronic myeloid leukemia – advances in biology and new approaches to treatment". N. Engl. J. Med. 349 (15): 1451–64
- Genomics England project cited from [Internet].; cited [December 2014]Available from: http://www.genomicsengland.co.uk/
- Nobel Prize cited from [Internet],; "The Nobel Prize in Chemistry 2014". Nobelprize.org. Nobel Media AB 2014. Web. 31 Dec 2014.
- Bajenoff, M. & Germain, R. N (2007).Seeing is believing: a focus on the contribution of microscopic imaging to our understanding of immune system function. Eur. J. Immunol. 37, S18–S33
- Entry to the Royal College of Physicians, based upon an engraving by William Hogarth, (1736) “Consultation of physicians or the arms of the undertaker”, cited from: https://www.rcplondon.ac.uk/update/physicians-cane-and-secrets-it-contained
Introduction
The microscope has been a mainstay of haematological investigation for hundreds of years. Microscopy was first used to observe red blood cells as early as 1658, but it took until the mid-19th century before the components of blood and their role in disease were more fully examined. Using stains developed by Ehrlich, microscope wielding researchers started to link what they saw in their blood films to clinical syndromes. Different forms of anaemia were identified and their causes classified. Identification of leukocytes and their role in diseases such as leukaemia came later. Microscopic examination of blood films and bone marrow samples now forms an important part of haematological diagnosis. However, new technologies such as automated cell counting, flow cytometry and genetic analysis may provide alternatives to the traditional method. Here we will examine what such techniques bring to the process of haematological diagnosis and whether they can truly replace the microscope.
Automated blood cell counting
The full blood count is one of the most routine tests done in any diagnostic setting. It provides a breakdown of the numbers of the different blood cells present in a sample. This is important in the diagnosis of conditions such as anaemia or haematological malignancies but is also used in other conditions such as infection or in inflammatory disorders. Traditionally, a full blood count is done manually. A blood film preparation is examined to provide an estimate of the numbers of different cells. This process is laborious, time consuming and only examines a small number of cells. While manual counting remains the gold standard, automated analysers are now used routinely to perform blood counts. Improved software and new technologies have allowed automated blood cell counters to evolve dramatically over the last two decades. New analysers are able to perform not only simple cell type counts, but also identify cells not normally found in peripheral blood, e.g. blasts, atypical lymphocytes and nucleated red cells.
While analytic performance is good for leukocyte and red cell counts, it is less satisfactory with some aspects of the leukocyte differentiation count and reticulocyte or platelet counts particularly when cell numbers are low.3 However the technology continues to improve and newer analysers are already showing comparable results to manual counting in areas such as immature granulocyte and nucleated red cell counts. One area where analysers have continued to have poor performance is in platelet counts. While some studies have indicated that automated platelet counts are a reliable predictor of clinical bleed risk it has been shown that analysers are inaccurate in measuring platelets in severe thrombocytopenia. They produce variable results and often wrongly over estimate platelet counts, which could clinically result in under perfusion of platelets. For low platelet counts external quality control, such as manual counting, is still needed until analyser accuracy is improved.
For simple blood cell counts, analysers do seem able to replace microscopy. They are able to provide rapid results and examine a far larger number of cells but there are still doubts about the efficacy of automated analysers where there is abnormal cell morphology. While it has been shown that counters are still able to count the abnormal and immature cells that can be seen in peripheral blood in disease states such as haematological malignancies,2 other abnormalities will still disrupt the count. Analysers can, in some circumstances, produce spurious counts e.g. if blood sample is inadequate, if there is platelet agglutination (such as that caused by EDTA), or if the patient’s pathology leads to fragmented red cells, other cell fragments, bacteria, fungi or abnormal lipids . In these cases a manual count is still often required. So while analysers can relieve much of the burden of blood counting, the microscope is still required in many abnormal states.
Analysis of Cell Morphology
One major advantage manual counting has over automated counting is that it allows the study of abnormal cellular characteristics at the same time. For example abnormal red cells such as spherocytes and poikilocytes are readily observed down the microscope but may be missed in an automated cell count and could even cause an error in the count. Some analysers are able to detect cellular abnormalities and can ‘flag’ these samples for further examination5. But there is variability in how analysers are calibrated and not all will flag every sample that needs further examination,8 meaning that some samples will be missed or subject to error prone counting.
It is likely that newer analysers will be able to detect and report on more abnormal cell types. Already in the last few decades, they have gone from just being able to detect red cells and leukocytes, to performing differential counts, reticulocyte counts and identify immature white cells. However, histological examination remains important. In many haematological malignancies, differential diagnoses can often only be ruled out after histological examination of a bone marrow or lymph node sample. A histological examination can also often give a very rapid diagnosis, for example the presence of Reed-Sternberg cells in a lymph node biopsy is diagnostic for Hodgkin’s lymphoma. Indeed, for some conditions e.g. Myelodysplasia, blood film analysis and histological examination of a bone marrow sample are actually required for diagnosis and classification.
Blood films also allow use of techniques such as immunohistochemistry where presence or absence of specific cell markers can be analysed. For example, presence or absence of specific markers can be used to predict prognosis in diffuse large B-cell lymphoma . However, technological advances have led to the development of flow cytometry as an alternative to immunohistochemistry, allowing more sophisticated analysis.
Flow Cytometry
Flow cytometry is a laser based technique which can be used to measure properties of individual cells such as size or surface antigens. Examination of surface antigens allows leukaemia or lymphoma typing. It can also be used to look for antibodies e.g. against platelets or neutrophils which is an important diagnostic feature of certain autoimmune diseases. DNA content can also be measured, allowing examination of aneuploidy seen in some cancers e.g. multiple myeloma and acute lymphoblastic anaemia.11
Flow cytometry can also be used for cell counting or sorting. For example, it allows the detection and quantification of fetal red cells in maternal blood. This can be important clinically when there is a need to prevent maternal exposure to fetal blood cells such as in the case of an Rh- mother carrying a Rh+ fetus. Flow cytometry also allows counting of reticulocytes, in a manner that is more sensitive and precise than manual counting. However, this method does not distinguish Howell-Jolley bodies from reticulocytes.11 So while it is more accurate than other methods of automated counting, it is not infallible.
Flow cytometry has also become an integral part of diagnosis of leukaemias and lymphomas. It is as effective as manual histological examination and allows more subtle differentiation between different antigen profiles. It is thus becoming a very useful diagnostic tool and in certain aspects of cellular analysis it is already replacing microscopy.
Genetic analysis
In certain haematological conditions, diagnosis could theoretically be made solely on the basis of a genetic test. This applies to certain inherited disorders such as heamoglobinopathies and clotting disorders and also to mutations found in certain myeloid malignancies. For example, Sickle cell anaemia can be diagnosed based on a blood film but equally, one could be just as sure of the diagnosis after a genetic test. Such genetic tests even allow a prenatal diagnosis . In sickle cell anaemia, genetic testing is relatively simple, as there is a recognised single point mutation. In other conditions e.g. thalassemia, the mutation is more variable, but within the last decade DNA sequencing has become relatively cheap, so genetic testing is also readily available.
Genetic testing is also used in malignancy. Particular translocations or mutations are characteristic of certain diseases. For example, in chronic myelogenous leukaemia (CML) there is the BCR-ABL1 translocation, which is easily detected using real time quantitative PCR (though it can also be detected using fluorescence microscopy). This translocation is diagnostic for CML and the mutated gene product produced can now be targeted with specific therapies. Detection of BCR-ABL1 positive cells using sensitive PCR can also be used for disease monitoring e.g. to detect malignant cells after transplant . Similarly, Polycythaemia Vera can be diagnosed solely on the basis of a high haematocrit and a JAK2 mutation in a non-hypoxic patient.
In certain conditions, genetic testing is therefore a viable alternative to microscopy. It can provide rapid results at an ever lower cost. But it is only relevant for inherited conditions with a known gene mutation or in malignancies where there is a characteristic genetic mutation. In other malignancies it may provide prognostic information, but is unlikely to be diagnostic and so in these conditions and other haematological disorders, histological examination is still likely to be required.
Conclusions
In conclusions, it appears that the 21st century haematologist does still require a microscope. While new technologies are providing alternatives and are likely to dramatically reduce the amount of time spent on histological examination, for some things the human eye is still the best we’ve got. Automated cell counting can manage most routine blood counts but errors still occur and in abnormal blood films, further manual examination is required, particularly when it comes to cell morphology. Flow cytometry allows rapid analysis of cellular characteristics and its uses are ever increasing. Similarly, genetic analysis also has the potential to replace the microscope in certain fields and is a very important diagnostic and prognostic marker.
It seems probable that, over time, use of the microscope will decrease as many aspects of microscopic examination are superseded by newer technologies. Perhaps a haematologist at the end of the 21st century will have no use for a microscope, but that seems unlikely. At the moment, manual histological examination remains a gold standard for many aspects of haematological diagnosis and even if the machines can take over some of that role, there will always be occasions when something goes wrong and it’s just easier to take a look yourself.
References
- Rolleston, R. The History of Haematology (Section of the History of Medicine). Proc R Soc Med 27, 1161-1178 (1934)
- Hijiya, N. et al Utility of automated counting to determine absolute neutrophil counts and absolute phagocyte counts for paediatric cancer treatment protocols. Cancer 101, 2681-6 (2004)
- Buttarello, M., Plebani, M. Automated Blood Cell Counts: State of the Art. Am J Clin Pathol 130, 104-116 (2008)
- Field, D., Taube, E. and Heumann, S. Performance evaluation of the immature granulocyte parameter on the Sysmex XE-2100 automated hematology analyser. Lab Hematol 12, 11-14 (2006)
- Ruzicka, K., Veitl, M., Thalhammer-Scherrer, R. and Schwarzinger, I. The new hematology analyzer Sysmex XE-2100: performance evaluation of a novel white blood cell differential technology. Arch Pathol Lab Med 125, 391-6 (2001)
- Lawrence, J. B. et al Reliability of automated platelet counts: comparison with manual method and utility for prediction of clinical bleeding. Am J Hematol 48, 244-250 (1995)
- Segal, H. C. et al Accuracy of platelet counting haematology analysers in severe thrombocytopenia and potential impact on platelet transfusion. Br J Haematol 128, 520-525 (2005)
- Zandecki, M., Genevieve, F., Gerard, J. and Godon, A. Spurious counts and spurious results on haematology analysers: a review. Part I: platelets. Int J Lab Hematol 29, 4-20 (2007)
- Killick, S. B. et al Guidelines for the Diagnosis and Management of Adult Myelodysplastic Syndromes. British Committee for Standards in Haematology (2013)
- Sjo, L. D. et al Profiling of diffuse large B cell lymphoma by immunohistochemistry: identification of prognostic subgroups. Eur J Haematol 79, 501-507 (2007)
- Brown, M. and Wittwer, C. Flow Cytometry: Principles and Clinical Applications in Hematology. Clinical Chem 46, 1221-1229 (2000)
- Kawano-Yanamoto, C. et al Two-color Flow cytometry with a CD19 Gate for the Evaluation of Bone Marrow Involvement of B-cell Lymphoma. Leuk Lymphoma 43, 2133-2137 (2002)
- Saiki, R. K. et al Enzymatic amplification of beta-globin genomic sequences and restriction site analysis for diagnosis of sickle cell anaemia. Science 230, 1350-1354 (1985)
- Eder, M. et al Monitoring of BCR-ABL expression using real time RT-PCR in CML after bone marrow or peripheral blood stem cell transplantation. Leukemia 13, 1383-1389 (1999)
- Spivak, J. L and Silver, R. T. The revised World Health Organisation diagnostic criteria for Polycythaemia vera, essential thrombocytosis, and primary myelofibrosis: an alternative proposal. Blood 112, 231-239 (2007)
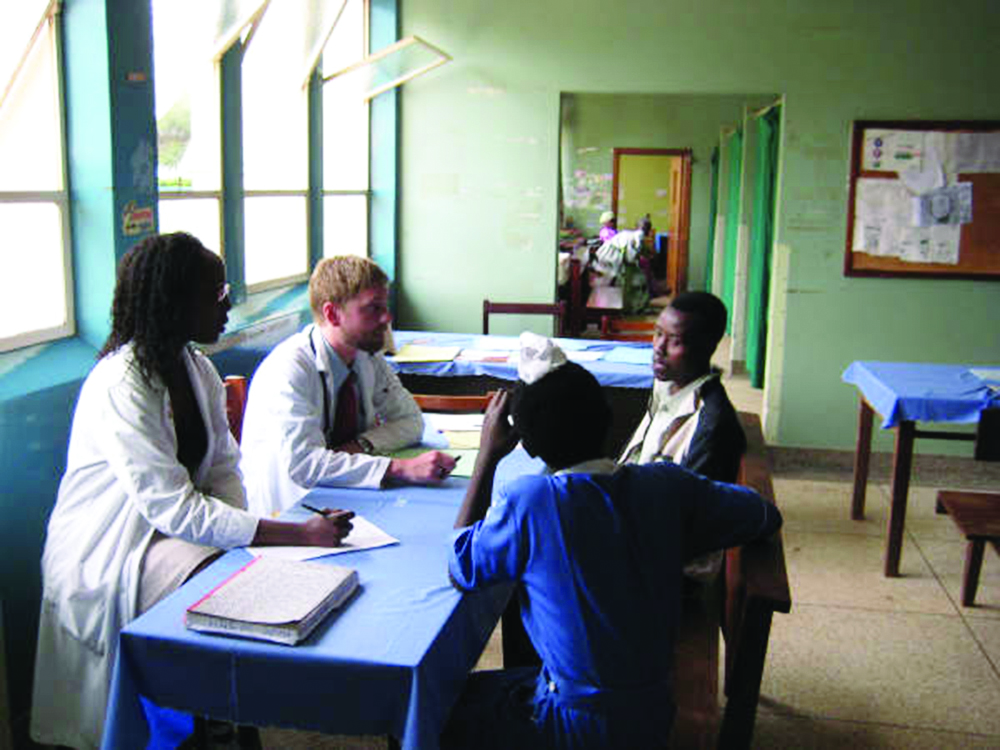
Student Elective Scholarships
The grants support UK-based undergraduate medical or Master in Science (MSc) students and biomedical and clinical sciense Students undertaking electives in the field of haematology.
Scholarships are available from 1 January and 1 June each year and are allocated on a first-come, first-served basis until the fund is depleted for teh six-month period. Successful applicants will have thier expenses reimbursed on completion of their proposed electives.